

AI-first infrastructure: The key to faster time to market
source link: https://venturebeat.com/ai/ai-first-infrastructure-the-key-to-faster-time-to-market/
Go to the source link to view the article. You can view the picture content, updated content and better typesetting reading experience. If the link is broken, please click the button below to view the snapshot at that time.
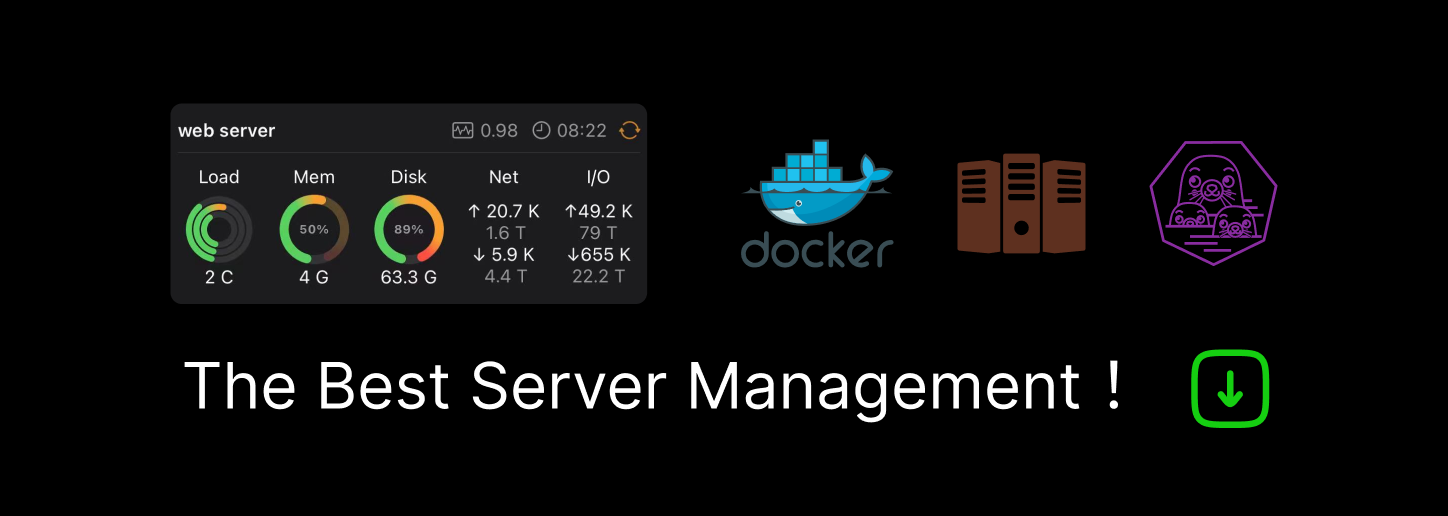
AI-first infrastructure: The key to faster time to market

Presented by Microsoft + NVIDIA
Purpose-built cloud infrastructure is the best way to quickly and economically grow AI at scale to create business value and growth. In this VB Spotlight, experts from Microsoft and NVIDIA explore infrastructure’s make-or-break role in the success of your AI strategy.
“The most important thing enterprises can do today is embrace a growth mindset when it comes to AI, and embrace it with both arms,” says Nidhi Chappell, General Manager, Azure HPC and AI, at Microsoft. “I have the privilege of a front-row seat, so I’ve seen how big a differentiator it is, and how much innovation it sparks.”
But the complexity and cost of implementing an AI strategy, especially putting pilots into production, remains a major challenge. That’s where high-performance AI infrastructure comes in.
An end-to-end purpose-built platform based in the cloud encompassing optimized processors, accelerators, networks, storage and software – enables enterprises to successfully operationalize and scale AI into production with improved standardization, cost control and governance.
An “AI-first” foundation can eliminate unmanaged procurement, uneven development and uncertain model performance, help cut down on duplicated efforts, tighten up workflows and eliminate many resource and time costs of getting all the parts of the tech stack to play nicely together.
Infrastructure optimized for diverse AI workloads
“AI is hardly a monolith,” reminds Chappell. “It’s a generic term that encompasses many different types of workloads — cost profiles vary widely especially for enterprises in varying stages of AI maturity”.
On one end of the spectrum, there are enterprises involved in high-end model training and inferencing large amounts of data. At the other end are enterprises using very light, prebuilt models and inferencing them in the field.
Rather than wrestling a behemoth into a smaller footprint, a standardized, cloud-based AI infrastructure can be optimized for a wide variety of use cases and workloads and a company’s particular scenarios. For example, a retailer might use AI for inventory management at the store level either daily or weekly. The cost structure for each end-to-end solution varies just as broadly.
To be clear, standardizing on an AI platform and cloud doesn’t mean vendor lock-in or relinquishing the reins of development. Instead, containerization, Kubernetes and other open, cloud-native approaches give enterprises portability across providers and clouds, giving CIOs the visibility and control they need without inhibiting innovation.
Calculating the costs
Terms like “purpose-built” can put IT decision-makers concerned about costs on guard.
“For those companies that are developing their own sophisticated models, concerned about their intellectual property, and need to string together thousands of GPUs for big model training, cost is often no barrier,” Chappell says. However, she adds, “The general enterprise market needs GPUs that are cost-optimized for training or fine tuning a pre-built model, low power and inexpensive inferencing.”
For every enterprise, it’s a delicate balance. Over-provisioning means expensive, under-utilized infrastructure; under-provisioning slows development and deployment, and can mean unexpected expenses to plug in the gaps or overages on cloud services. For companies deploying less sophisticated AI, purpose-built infrastructure can be scaled down to a cost-effective level.
And because standardized infrastructure accelerates development and deployment, companies gain a competitive advantage from putting AI into production faster, which canreduce total cost of ownership (TCO).
Advises Chappell: “Don’t look at the cost of infrastructure, look at the cost of developing a model or doing inference. That’s the real metric. Then consider the intellectual property you’re developing — what’s that worth?”
To learn more about purpose-built AI platforms, how end-to-end AI environments help lower costs, improve innovation, and speed time to production and real-world ROI, don’t miss this VB Spotlight.
Agenda
- Enabling orderly, fast, cost-effective development and deployment
- Focusing and freeing funds for ongoing innovation and value
- Ensuring accountability, measurability and transparency
- How infrastructure directly impacts the bottom line
Speakers
- Nidhi Chappell, General Manager, Azure HPC and AI, Microsoft
- Manuvir Das, Head of Enterprise Computing, NVIDIA
- Joe Maglitta, Host and Moderator, VentureBeat
Recommend
-
8
TechCryptocurrency market value tops $2 trillion for the first time as ethereum hits record highPublished Tue, Apr 6 20212:26 AM EDTUpdated Tue, Apr...
-
7
News For the First Time, Xilinx Breaks Into the System-on-Module (SOM) Market 20 hours ago by Jake Hertz All About Circuits sat down with Xi...
-
5
Chip Huyen on Streaming-First Infrastructure for Real-Time ML Dec 07, 2021...
-
3
<?xml encoding="utf-8" ??>Introduction RPKI (Resource Public Key Infrastructure) is a way to help prevent BGP hijacking. It uses cryptographic signatures to validate that an ASN is allowed to an...
-
4
Sponsored The 3 key strategies to slash time-to-market in any industry
-
4
Machine Learning (ML) is one of the fastest growing segments within cloud and edge infrastructure. Within ML, deep learning inference is expected to grow even faster. In this blog, we compare the ML inference performance of three Amazon Web...
-
6
Accelerating AI for growth: The key role of infrastructure Skip to main content...
-
3
Streaming-First Infrastructure for Real-Time Machine Learning In t...
-
6
Introduction You’ve probably wondered how banks are able to decide precisely who gets a loan and who doesn’t. Despite dealing with thousands of different applicants, their systems are always equipped to make the appropri...
-
4
UK warns of potential Russian hacker attacks on key infrastructure
About Joyk
Aggregate valuable and interesting links.
Joyk means Joy of geeK