

Removing Demographic Data Can Make AI Discrimination Worse
source link: https://hbr.org/2023/03/removing-demographic-data-can-make-ai-discrimination-worse
Go to the source link to view the article. You can view the picture content, updated content and better typesetting reading experience. If the link is broken, please click the button below to view the snapshot at that time.
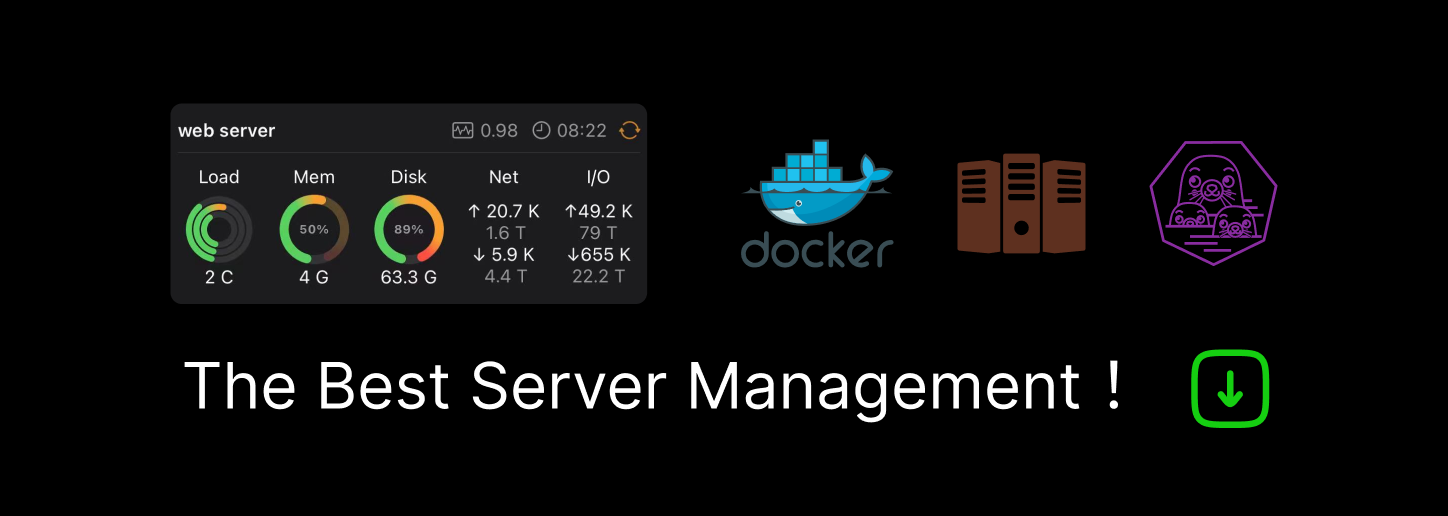
Removing Demographic Data Can Make AI Discrimination Worse

Decisions about who to interview for a job, who to provide medical care to, or who to grant a loan were once made by humans, but ever more frequently are made by machine learning (ML) algorithms, with eight in 10 firms planning to invest in some form of ML in 2023 according to New Vantage. The number one focus of these investments? Driving business growth with data.
While data can come in many forms, when focused on generating business growth a firm is usually interested in individual data, which can belong to customers, employees, potential clients, or almost anyone the organization can legally gather data on. Data is fed into ML algorithms which find patterns in the data or generates predictions — these outcomes are then used to make business decisions — generally about who or what to focus business efforts on.
While investment in ML algorithms continues to grow and drive greater business efficiencies — 30% or more, according to a recent McKinsey report — the use of ML models and individual data does come with some risks, ethical ones to be specific. The World Economic Forum cites unemployment, inequality, human dependency, and security amongst its top risk of using artificial intelligence and ML, but by far the biggest ethical risk in practice is discrimination.
The Biggest Risk
To be sure, unjustified discrimination by firms has always existed. Discrimination of historically disadvantaged groups has led to the formulation of several anti-discrimination laws, including the Fair Housing Act of 1968 and the Equal Credit Opportunity Act of 1974 in the United States, and the European Union Gender Directive. The lending space, in particular, has been a ground for discriminatory treatment, up to the point that discrimination in mortgage lending has been viewed as one of the most controversial civil rights topics.
Historically, in hopes of preventing discriminatory decisions, sensitive data, such as individual race, gender, and age has been excluded from important individual decisions such as loan access, college admission, and hiring. Whether sensitive data has been excluded in line with anti-discrimination laws (such as the exclusion of race and gender data from consumer non-mortgage loan applications in the United States due to the Equal Credit Opportunity Act) or a firm’s risk management practices, the end result is the same; firms rarely have access to, or use sensitive data to make decisions that impact individuals — whether they are using ML or human decision makers.
At first glance this makes sense; exclude individual sensitive data and you cannot discriminate against those groups. Consider how this works when determining who to interview for a job, first with human-based decision making. A human resources expert would remove the names and genders of applicants from resumes before analyzing candidate credentials to try to prevent discrimination in determining who to interview. Now, consider this same data exclusion practice when the decision is made with a ML algorithm; names and genders would be removed from the training data before it is fed into the ML algorithm, which would then use this data to predict some target variable, such as expected job performance, to decide who to interview.
But while this data exclusion practice has reduced discrimination in human-based decision making, it can create discrimination when applied to ML-based decision making, particularly when a significant imbalance between population groups exists. If the population under consideration of a particular business process is already skewed (as is the case for credit requests and approvals) ML will not be able to solve the problem by merely replacing the human decision maker. This became evident in 2019 when Apple Card faced accusations of gender-based discrimination despite not having used gender data in the development of their ML algorithms. Paradoxically, that turned out to be the reason for the unequal treatment of customers.
The phenomenon is not limited to the lending space. Consider a hiring decision-making process at Amazon which aimed to use a ML algorithm. A team of data scientists, trained a ML algorithm on resume data to predict job performance of applicants in hopes of streamlining the process of selecting individuals to interview. The algorithm was trained on the resumes of current employees (individual data), with gender and names removed, in hopes of preventing discrimination, per human decision-making practices. The result was the exact opposite — the algorithm discriminated against women, by predicting them to have significantly lower job performance than similarly skilled men. Amazon, thankfully, caught this discrimination before the model was used on real applicants, but only because they had access to applicant gender, despite not using it to train the ML algorithm, with which to measure discrimination.
The Case for Including Sensitive Data
In a recent study published in Manufacturing & Services Operations Management we consider a fintech lender who uses a ML algorithm to decide who to grant a loan to. The lender uses individual data of past borrowers to train a ML algorithm to generate predictions about whether a loan applicant will default or not, if given a loan. Depending on the legal jurisdiction and the lender’s risk management practices, the lender may or may not have collected sensitive attribute data, such as gender or race, or be able to use that data in training the ML algorithm. (Although our research focuses on gender, this should not diminish the importance of investigating other types of algorithmic discrimination. In our study, gender was reported as either woman or man; we acknowledge gender is not binary, but were restricted by our dataset.)
Common practice, as we noted above, whether it be for legal or risk management reasons, is for the lender to not use sensitive data, like gender. But we ask instead, what might happen if gender was included? While this idea may come as a shock to some, it is common practice in many countries to collect gender information (for example, Canada and countries in the European Union) and even to use it in ML algorithms (for example, Singapore).
Including gender significantly decreases discrimination — by a factor of 2.8 times. Without access to gender, the ML algorithm over-predicts women to default compared to their true default rate, while the rate for men is accurate. Adding gender to the ML algorithm corrects for this and the gap in prediction accuracy for men and women who default diminishes. Additionally, the use of of gender in the ML algorithm also increases profitability on average by 8%.
The key property of gender data in this case is that it provides predictive power to the ML algorithm.
Given this, when gender is excluded, three things can happen: 1) some amount of predictive information directly tied to gender is lost, 2) unfair gender discrimination that may be introduced in the process cannot be efficiently controlled or corrected for and 3) some portion of that information is estimated by proxies — variables which are highly correlated with another, such that when one variable, such as gender, is removed, a series of other variables can triangulate that variable.
We find that proxies (such as profession, or ratio of work experience to age) can predict gender with 91% accuracy in our data, so although gender is removed, much gender information is estimated by the algorithm through proxies. But these proxies favor men. Without access to the real gender data the ML algorithm is not able to recover as much information for women compared to men, and the predictions for women suffer, resulting in discrimination.
Proxies were also a key factor in the discrimination in Amazon’s hiring ML algorithm, which did not have access to gender, but had access to various gender proxies, such as colleges and clubs. The ML algorithm penalized the resumes of individuals with terms like “women’s chess club captain” and downgraded graduates of all-women’s colleges because it was trained on a sample of current software engineering employees, who, it turns out, were primarily men, and no men belonged to these clubs or attended these colleges.
This is not only a problem with gender discrimination. While our research focuses on gender as the sensitive attribute of interest, a similar effect could occur when any sensitive data with predictive value is excluded from a ML algorithm, such as race or age. This is because ML algorithms learn from the historical skewness in the data and discrimination could further increase when the sensitive data category has smaller minority groups, for instance, non-binary individuals in the gender category, or if we consider the risks of intersectional discrimination (for example, the combination of gender and race, or age and sexual orientation).
Our study shows that, when feasible, access to sensitive attributes data can substantially reduce discrimination and sometimes also increase profitability.
To understand how this works, refer back to the lending situation we studied. In general, women are better borrowers than men, and individuals with more work experience are better borrowers than those with less. But women also have less work experience, on average, and represent a minority of past borrowers (on which ML algorithms are trained).
Now, for the sake of this stylized example, imagine that a woman with three years of work experience is sufficiently credit-worthy while a man is not. Having access to gender data the algorithm would correctly predict that, resulting in the issue of loans to women with three years of experience, but denying them to men.
But when the algorithm does not have access to gender data, it learns that an individual with three years of experience is more like a man, and thus predicts such an individual to be a bad borrower and denies loans to all applicants with three years of experience. Not only does this reduce the number of profitable loans issued (thus hurting profitability), but such a reduction comes solely from denying loans to women (thus increasing discrimination).
What Companies Can Do
Obviously, simply including gender will improve the number of loans granted to women and company profitability. But many companies cannot simply do that. For these, there is some light at the end of the tunnel, with several new artificial intelligence regulations being enacted in the coming few years, including New York City’s Automated Employment Decision Tools Law, and the European Union Artificial Intelligence Act.
These laws appear to steer clear of strict data and model prohibitions, instead opting for risk-based audits and a focus on algorithm outcomes, likely allowing for the collection and use of sensitive data across most algorithms. This type of outcome-focused AI regulation is not entirely new, with similar guidelines proposed in the Principles to Promote Fairness, Ethics, Accountability, and Transparency from the Monetary Authority of Singapore.
In this context, there are three ways companies may in future be able to work gender data into ML decision making. They can 1) pre-process data before a ML algorithm training (e.g., down sampling men or up sampling women) so that the model trains on a more balanced data, 2) impute gender from other variables (e.g., professions, or a relationship between work experience and number of children), and 3) tune model hyper-parameters with gender, and then remove gender for model parameter estimation.
We found that these approaches significantly reduced discrimination with minor impact on profitability. The first approach reduces discrimination by 4.5-24% at the cost of a small reduction in overall loan profitability of 1.5-4.5%. The second reduces discrimination by nearly 70% and increases profitability by 0.15% respectively, and the third reduces discrimination by 37% at the cost of about 4.4% in reduced profitability. (See our paper for more details.)
In some cases, and if these other strategies are not effective, firms may find it better simply to restore decision rights to humans. This, in fact is what Amazon did after reviewing the discrimination issues with its hiring AI software.
We encourage firms, therefore, to take an active role in conversations with regulatory bodies that are forming guidelines in this space, and to consider the responsible collection of sensitive data within the confines of their relevant regulations, so they can, at minimum, measure discrimination in their ML algorithm outcomes, and ideally, use the sensitive data to reduce it. Some firms may even be permitted to use the data for initial ML algorithm training, while excluding it from individual decisions.
This middle ground is better than not using the sensitive data at all as the aforementioned methods can help to reduce discrimination with minor impact, and sometimes even an increase, in profitability. In time, and as more evidence emerges that sensitive data can be responsibly collected and used, we must hope that a framework emerges that enables its use.
Recommend
About Joyk
Aggregate valuable and interesting links.
Joyk means Joy of geeK