

[2012.06713] Approximate Trace Reconstruction
source link: https://arxiv.org/abs/2012.06713
Go to the source link to view the article. You can view the picture content, updated content and better typesetting reading experience. If the link is broken, please click the button below to view the snapshot at that time.
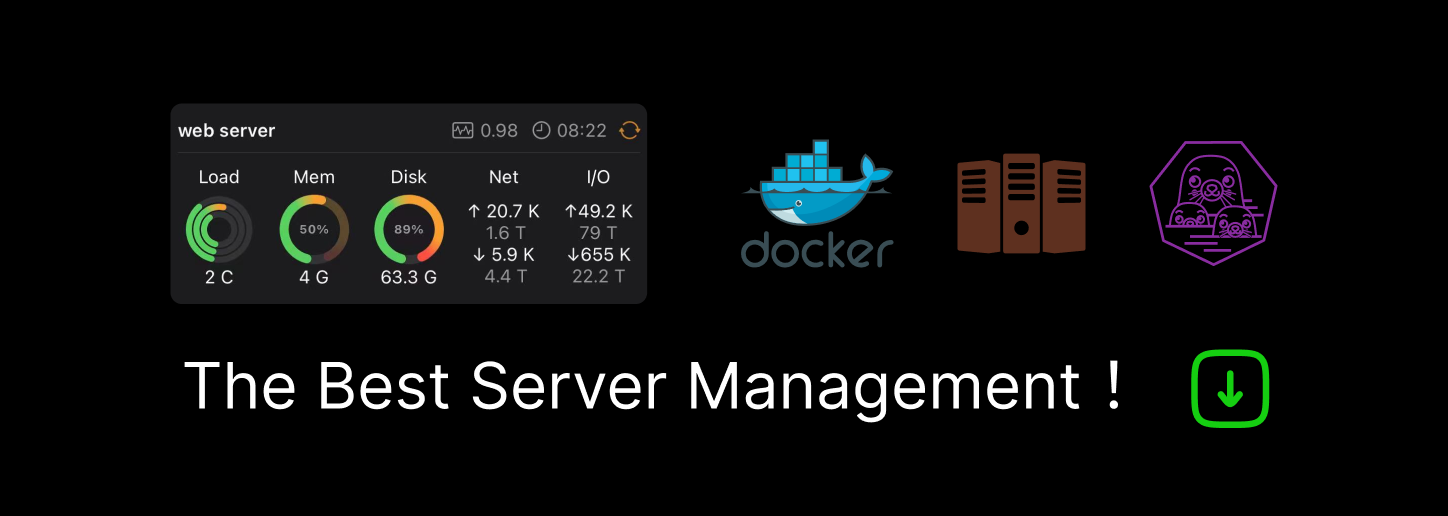
[Submitted on 12 Dec 2020 (v1), last revised 16 Dec 2020 (this version, v2)]
Approximate Trace Reconstruction
In the usual trace reconstruction problem, the goal is to exactly reconstruct an unknown string of length n after it passes through a deletion channel many times independently, producing a set of traces (i.e., random subsequences of the string). We consider the relaxed problem of approximate reconstruction. Here, the goal is to output a string that is close to the original one in edit distance while using much fewer traces than is needed for exact reconstruction. We present several algorithms that can approximately reconstruct strings that belong to certain classes, where the estimate is within n/\mathrm{polylog}(n) edit distance, and where we only use \mathrm{polylog}(n) traces (or sometimes just a single trace). These classes contain strings that require a linear number of traces for exact reconstruction and which are quite different from a typical random string. From a technical point of view, our algorithms approximately reconstruct consecutive substrings of the unknown string by aligning dense regions of traces and using a run of a suitable length to approximate each region. To complement our algorithms, we present a general black-box lower bound for approximate reconstruction, building on a lower bound for distinguishing between two candidate input strings in the worst case. In particular, this shows that approximating to within n^{1/3 - \delta} edit distance requires n^{1 + 3\delta/2}/\mathrm{polylog}(n) traces for 0< \delta < 1/3 in the worst case.
Subjects: | Data Structures and Algorithms (cs.DS); Computational Complexity (cs.CC); Information Theory (cs.IT); Machine Learning (cs.LG); Probability (math.PR) |
Cite as: | arXiv:2012.06713 [cs.DS] |
(or arXiv:2012.06713v2 [cs.DS] for this version) | |
https://doi.org/10.48550/arXiv.2012.06713 |
Recommend
About Joyk
Aggregate valuable and interesting links.
Joyk means Joy of geeK