

Using Metrics to Evaluate Chatbot Effectiveness
source link: https://uxplanet.org/using-metrics-to-evaluate-chatbot-effectiveness-3506330ea1b2
Go to the source link to view the article. You can view the picture content, updated content and better typesetting reading experience. If the link is broken, please click the button below to view the snapshot at that time.
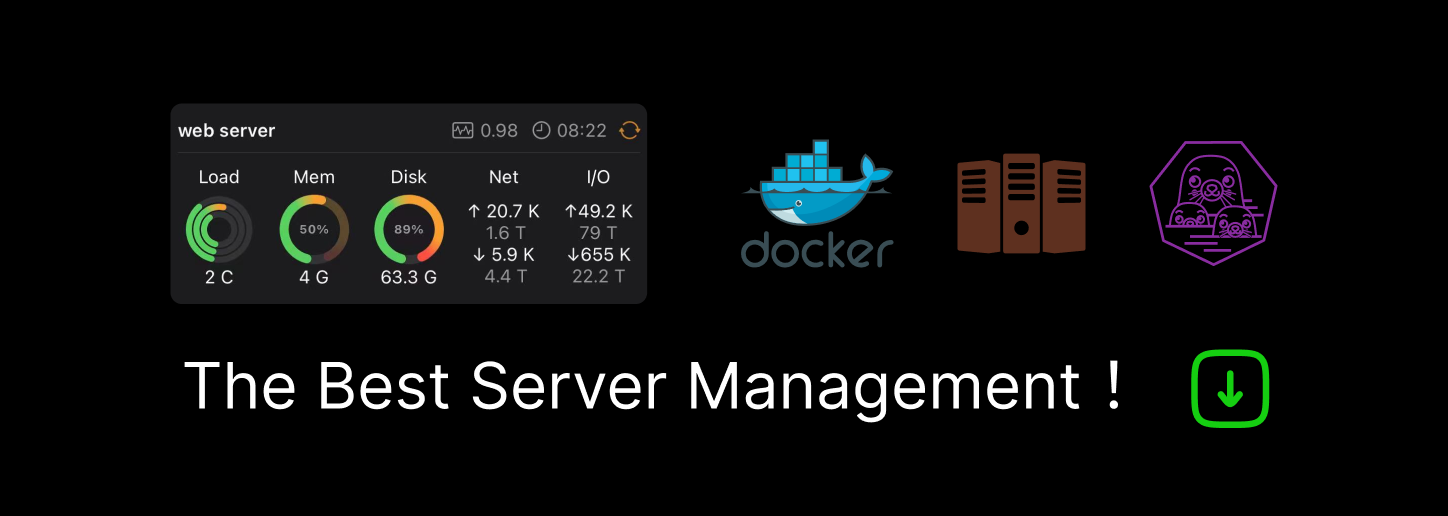
Using Metrics to Evaluate Chatbot Effectiveness
Three types of metrics you should be measuring
Imagine you’re the proud owner of a popular online store. You’ve just installed a new chatbot to help customers with their purchases. You’re excited about the bot’s potential to elevate the customer experience and take some of the pressure off your support team. But the question is: how do you know if the chatbot is actually effective?
To understand how well your chatbot is performing and make informed decisions on how to improve its effectiveness, it’s important to track key performance indicators (KPIs). By leveraging metrics, you can gain valuable insights into how users are engaging with your chatbot and identify areas for improvement. This article will dive into the various metrics used to evaluate chatbot effectiveness across channels and provide practical tips for optimizing your chatbot’s performance.
Defining chatbot metrics
Before we dive into specific metrics, let’s define what we mean by “chatbot metrics.” Chatbot metrics are key performance indicators (KPIs) that measure the effectiveness of a chatbot in achieving its goals. These goals can include improving customer engagement, driving sales, or reducing support tickets.
So, what are some of the key chatbot metrics you should be tracking?
1. Engagement
Engagement metrics, for instance, give you insight into how many users are interacting with your chatbot and for how long. Tracking engagement metrics can help you understand the effectiveness of your chatbot’s design, content, and user experience. Some examples of engagement metrics include:
- The number of conversations started by users.
- The length of each conversation.
- The average time spent by users engaging with the chatbot.
2. Satisfaction
Satisfaction metrics, on the other hand, measure how content users are with their chatbot experience. This metric is especially important because happy users are more likely to return and recommend your chatbot to others. Some examples of satisfaction metrics include:
- The percentage of users who complete a conversation with the chatbot.
- The percentage of users who leave a positive rating or feedback.
- The number of unresolved support tickets.
3. Retention
Finally, retention metrics track how often users come back to use your chatbot. This metric is crucial because it measures the long-term success of your chatbot and its ability to keep users engaged. Some examples of retention metrics include:
- The number of repeat users over a given period.
- The frequency of chatbot usage by individual users.
- The percentage of users who return to the chatbot after a set period of time.
By closely monitoring these metrics, you can detect user behavior patterns and trends, assess whether your chatbot is being used as intended, and make the necessary adjustments to enhance the user experience. Now, let’s explore how you can use these metrics in greater detail to evaluate the effectiveness of your chatbot.
Setting goals and objectives
To effectively measure chatbot metrics, it’s important to set specific goals and objectives. Without clear goals, it’s difficult to know what metrics to track and how to optimize your chatbot’s performance.
SMART goals
SMART goals are Specific, Measurable, Achievable, Relevant, and Time-bound. When setting goals for chatbot metrics, it’s important to make sure they are defined well enough that you can realistically attain them within a specific time-frame, and measure the results against key metrics.
An example of a SMART goal for a chatbot could be to increase customer engagement by 20% within the next three months.
Objectives and Key Results (OKRs) is a popular goal-setting framework used by many tech companies. OKRs are set by identifying one or more specific objectives and defining measurable key results that indicate progress toward achieving those objectives.
An example of OKRs for a chatbot could be:
- Objective: Improve user satisfaction with the chatbot’s customer service interactions within the next quarter.
- Key Result #1: Increase chatbot satisfaction rating from 3.5 to 4.5 stars.
- Key Result #2: Reduce the number of negative feedback responses received through the chatbot by 50%.
Regardless of the framework you choose, it’s important to involve all relevant stakeholders in the goal-setting process, including your chatbot development team, customer service team, and any other departments that may be impacted by the chatbot’s performance. This helps ensure that everyone is aligned on the goals and objectives and is working towards a common vision.
Measuring engagement
By tracking engagement metrics, you can gain a better understanding of how users are interacting with your chatbot and make data-driven decisions to improve its performance. Engaged users are more likely to make purchases, provide feedback, and become loyal customers.
Here are a few metrics you can use to measure user engagement.
1. Active users
Active users measure how many people are using your chatbot on a regular basis. You can calculate this by looking at the number of unique users who interacted with your chatbot during a specific period.
For example, if 100 users interacted with your chatbot during a week, your active user count for that week is 100. If the number of active users is stagnating or declining, that could indicate that users are finding the chatbot less helpful or engaging, or that they are switching to a competitor’s chatbot.
To improve active users, the chatbot should be easy to find and access. Promoting and integrating the chatbot into existing communication channels can accomplish this. Creating a seamless user experience and providing personalized recommendations can help keep users engaged and coming back.
2. Session length
Session length measures how long users are interacting with your chatbot during a single session. Longer session lengths suggest that users are finding value in the chatbot and engaging with it for an extended period.
For example, if the average session length for your chatbot is five minutes, this suggests that users are spending a considerable amount of time engaging with your chatbot. A dropoff in session length can mean that users are losing interest or not finding the information they need, and are exiting the chatbot earlier than before.
Session length can be addressed by improving the chatbot’s content and functionality. Offering relevant and valuable information can keep users engaged for longer periods. The chatbot should be responsive and provide quick and helpful responses to user inquiries.
3. Conversion rate
Conversion rate measures how many users who interacted with your chatbot ended up making a purchase or completing a desired action.
For example, if 10 users interacted with your chatbot and two of them made a purchase, your conversion rate is 20%. High conversion rates suggest that your chatbot is effective at driving user actions and achieving business goals. If your conversion rates are low, this could mean that the chatbot is not effectively guiding users toward the desired outcome, or that the outcome itself is not compelling enough to motivate users to take action.
Conversion rates can be improved by understanding user pain points. Simplifying a checkout process, offering incentives or promotions, or providing additional information or support can help users make informed decisions.
Measuring satisfaction
Measuring user satisfaction is important for understanding the user experience of the chatbot. Satisfied users are more likely to continue using the chatbot, recommend it to others, and make purchases through the chatbot. They may also provide positive feedback or leave reviews, which can help attract new users and improve the overall reputation of the chatbot.
Here are a few metrics that can be used to evaluate user satisfaction.
1. Customer satisfaction (CSAT)
Customer satisfaction (CSAT) is a widely used metric to measure customer satisfaction with a product or service, including chatbots. CSAT scores are typically collected through post-interaction surveys or polls where users are asked to rate their satisfaction on a scale of 1–5 or 1–10. These scores can be used to gauge how well the chatbot is meeting the needs and expectations of its users.
A CSAT score of 4 or higher (out of 5) is considered good, and a score of 3 or lower indicates that improvements need to be made. For example, if a chatbot receives a low CSAT score, the chatbot team can review the chat logs and identify areas for improvement, such as adding more relevant content or improving the accuracy of responses.
One effective strategy for improving CSAT scores is to provide personalized and relevant interactions with users. This can be accomplished by leveraging user data and past interactions to provide tailored recommendations and solutions. It’s also important to ensure that the chatbot is responsive and helpful in addressing user inquiries and issues.
2. Net promoter score (NPS)
Net promoter score (NPS) is another metric commonly used to measure customer satisfaction and loyalty. NPS surveys ask users a single question: “On a scale of 0 to 10, how likely are you to recommend this chatbot to a friend or colleague?” Based on their response, users are categorized into three groups: Promoters (score 9–10), Passives (score 7–8), and Detractors (score 0–6).
The NPS score is calculated by subtracting the percentage of Detractors from the percentage of Promoters. A high NPS score indicates that users are more likely to recommend the chatbot to others, which can lead to increased user adoption and engagement.
To improve NPS, it’s important to focus on creating a positive overall experience for users. This can be accomplished by providing exceptional customer service, resolving issues quickly and effectively, and offering incentives or promotions to encourage positive word-of-mouth promotion.
3. Sentiment analysis
Sentiment analysis is a technique used to determine the overall sentiment or emotional tone of a text-based conversation, such as chat logs. Sentiment analysis tools use natural language processing (NLP) algorithms to analyze the words and phrases used in the conversation and classify them as positive, negative, or neutral.
Sentiment analysis can be useful for understanding how users feel about their interactions with the chatbot. For example, if the sentiment analysis reveals a high percentage of negative comments or feedback, it could indicate that users are experiencing frustration or dissatisfaction with the chatbot. In this case, the chatbot team can use this information to identify areas for improvement, such as improving the accuracy of responses or adding more personalized recommendations.
Measuring retention
Retention is a measure of how frequently users return to use a chatbot. It’s an important metric for measuring the effectiveness of a chatbot because it indicates the level of engagement and value that users are receiving from the chatbot. A high retention rate suggests that users are finding the chatbot helpful and enjoyable to use, while a low retention rate may indicate that users are not finding the chatbot useful or are encountering issues that prevent them from returning.
Here are a few metrics that you can use to measure user retention.
1. Churn rate
Churn rate measures the rate at which users stop using your chatbot over a specific period. It’s calculated by dividing the number of users who stopped using your chatbot by the total number of users at the beginning of the period.
To improve the churn rate, it’s important to understand the reasons why users are leaving and address them directly. This may involve improving the user experience, providing additional support or resources, or offering promotions or discounts to encourage users to stay.
2. Repeat usage
Repeat usage measures how many users come back to use your chatbot multiple times over a specific period. It’s calculated by dividing the number of users who used your chatbot more than once by the total number of users during that period.
To improve the repeat usage rate of a chatbot, provide a valuable and personalized experience for the user by tailoring the chatbot’s responses accordingly to their needs or preferences. Another strategy to improve repeat usage is to offer incentives or rewards for continued usage. This can include special promotions or discounts for frequent users or a loyalty program that rewards users for using the chatbot over time.
3. Time between sessions
Time between session measures the time interval between two user sessions on your chatbot. It’s calculated by dividing the total time between sessions by the number of users who came back for multiple sessions.
To improve the time between sessions, it’s important to focus on providing ongoing value and relevance to users. Regularly updating the chatbot with new and relevant information, personalized recommendations, and features that address user needs can help encourage users to return to the chatbot more frequently.
Measuring effectiveness across channels
Finally, it’s important to measure chatbot effectiveness across different channels, including web, mobile, and voice assistants. Each channel has unique user behavior and interaction patterns, and it’s important to optimize your chatbot’s performance for each channel.
When it comes to web-based chatbots, one of the key metrics to measure is engagement. This can be done by tracking the number of users who initiate conversations, as well as the number of messages exchanged and the average session length. Additionally, tracking user behavior after interacting with the chatbot, such as page views or conversion rates, can provide insight into the chatbot’s impact on overall website performance.
For mobile chatbots, it’s important to measure both engagement and retention. Tracking the number of active users, session length, and frequency of use can provide insight into engagement while measuring retention can be done by tracking the number of returning users and the time between each use. Additionally, tracking app store ratings and reviews can provide valuable feedback on user satisfaction and areas for improvement.
Voice assistant chatbots require a different approach to measurement, as they are typically used in a hands-free manner and are often integrated into other devices or services. Metrics to track may include the number of active users, frequency of use, and the types of queries or actions users are taking. Additionally, tracking user satisfaction through feedback or ratings can help to identify areas for improvement.
By measuring chatbot metrics across channels, you can identify areas for improvement and optimize your chatbot’s performance to improve user experience.
Recommend
About Joyk
Aggregate valuable and interesting links.
Joyk means Joy of geeK