

Training Compute-Optimal Large Language Models 简读
source link: https://finisky.github.io/training-compute-optimal-large-language-models-summary/
Go to the source link to view the article. You can view the picture content, updated content and better typesetting reading experience. If the link is broken, please click the button below to view the snapshot at that time.
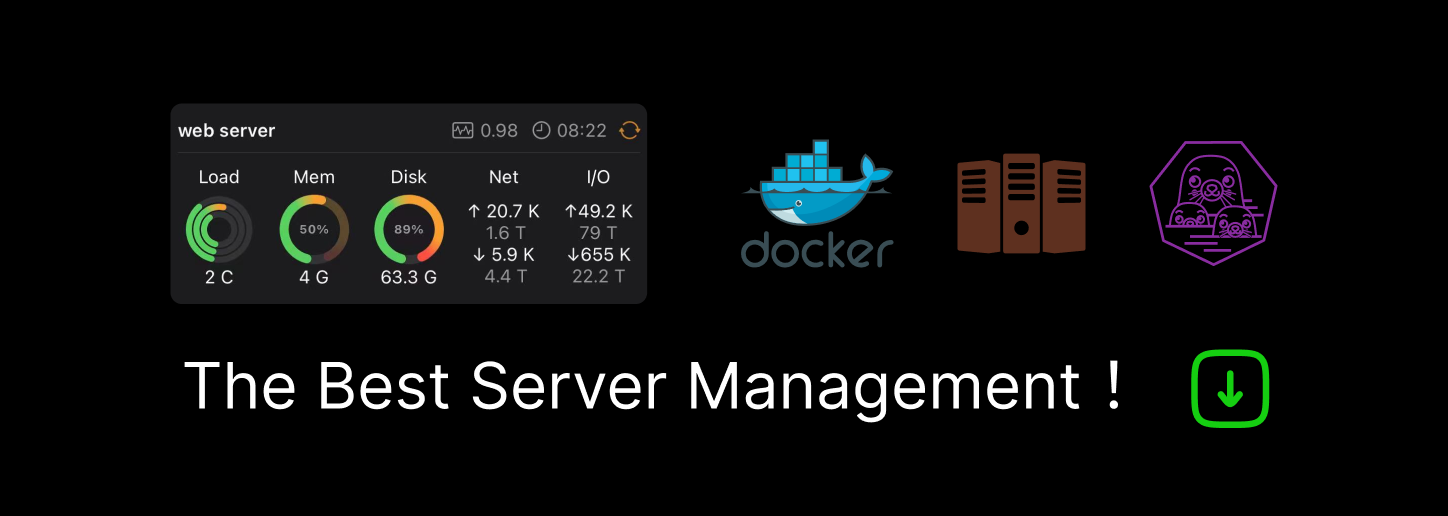
Training Compute-Optimal Large Language Models 简读
DeepMind去年在 NeurIPS 2022 发表了一篇如何在给定计算资源条件下,用多少tokens训练最优大小的 Large Language Models (LLM)。之前的许多工作都仅专注于扩大模型规模,而并不增加训练数据规模,导致这些模型显著地训练不到位 (undertrained)。DeepMind训练用不同规模的数据 (从5B到500B tokens) 训练超过400个不同大小的模型 (从70M到超过16B),发现 模型和训练数据规模需要同比增大。根据这个假设,使用与 Gopher (280B) 同样的计算量且4倍的数据,训练了70B的最优模型 Chinchilla。它在许多下游任务上的性能显著超过了 Gopher (280B), GPT-3 (175B) Jurassic-1 (178B) 和 Megatron-Turing NLG (530B)。
[NeurIPS 2022] Training Compute-Optimal Large Language Models Training Compute-Optimal Large Language Models
本文的 Chinchilla 也是后续对话系统 Sparrow 的基模型。
大模型面临的一些挑战,主要是训练开销及高质量数据:
Large language models face several challenges, including their overwhelming computational requirements (the cost of training and inference increase with model size) and the need for acquiring more high-quality training data.
名词解释:
- FLOPs:s小写,floating point operations,指浮点运算次数,作为计算量的衡量指标。
本文主要解决的问题,在给定计算量预算的情况下,如何在模型大小和训练数据量之间取得平衡:
Given a fixed FLOPs budget, how should one trade-off model size and the number of training tokens?
本文采用三种不同的方案来回答上述问题。之前的相关工作假设模型大小与计算量之间满足power-law定律,这里也一样。
固定模型大小,改变训练数据量
在 70M ~ 10B 的一系列模型上,用4个不同数据量的模型训练。根据这些训练数据点,可以得到计算量C,模型大小N和数据量 (training tokens) D之间的映射关系,三者之间要满足 FLOPs(Nopt,Dopt)=C。因为假设它们之间有power-law关系,所以 Nopt∝Ca,Dopt∝Cb。这些数据点用来估计超参数a和b。
根据上表 (Table 2)结果,得出a=0.5,b=0.5。
固定计算量,改变模型大小
固定9个不同的计算量 (FLOPs),训练不同模型大小,检查最后的training loss。得到下图:
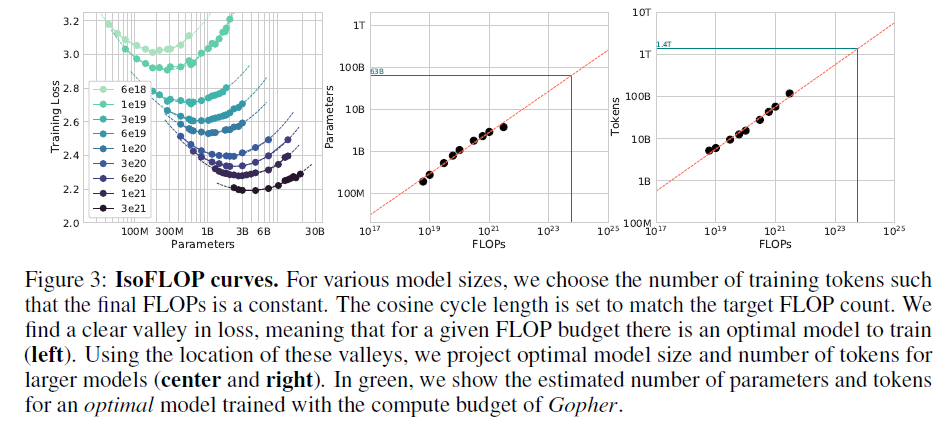
可以发现对每个FLOPs,都有一个最小的loss,也就是最优模型大小。据此,中图和右图对于更大模型的模型大小与计算量关系进行了投影。用这种方式对参数a和b的估计结果列在 Table 2 中,a=0.49,b=0.51。
根据 classical risk decopmosition,将损失建模为函数:
L^(N,D)≜E+ANα+BDβ
超参数估计通过最小化Huber loss完成:
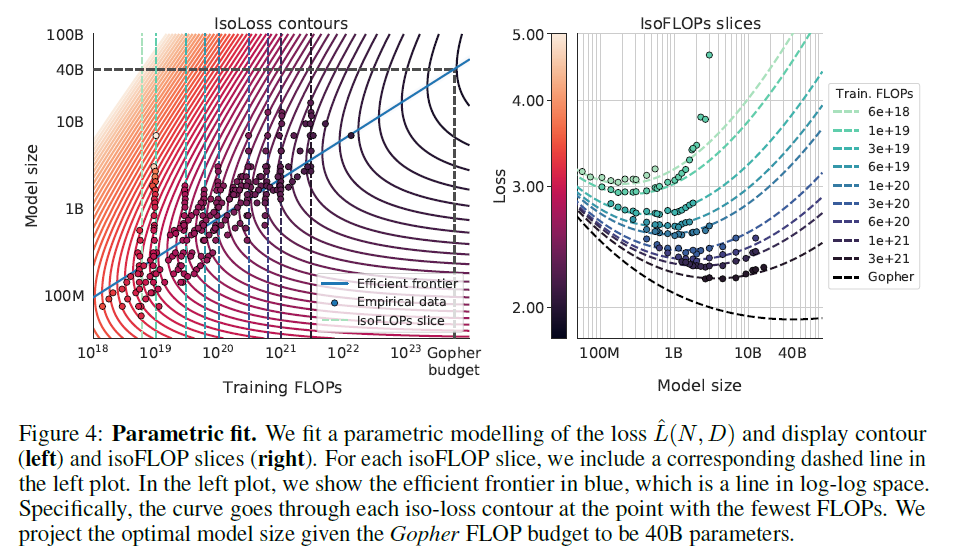
用这种方式对参数a和b的估计结果也在 Table 2中,a=0.46,b=0.54。
Table 2总结了实验结果,三种方案得到的结论基本一致: 在计算量增大时,模型大小和训练数据也应该同比增大。
All three approaches suggest that as compute budget increases, model size and the amount of training data should be increased in approximately equal proportions
通过下图也能发现当前的许多LLM的参数在给定当前计算量的情况下显然过大了,并且训练数据的规模也没有达到,所以目前并不需要一味增加模型的大小:
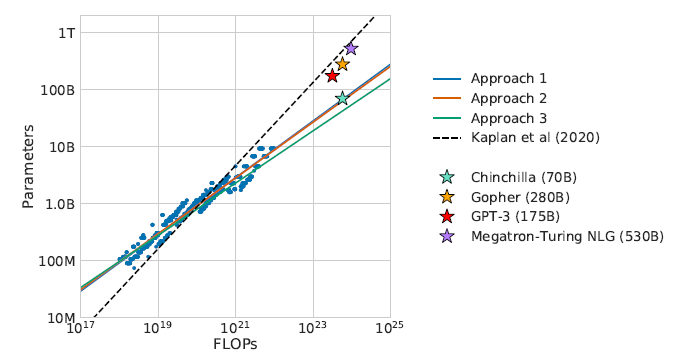
Chinchilla
基于上述分析,Gopher (280B) 模型的最优大小应该在 40B ~ 70B 之间,于是按模型大小的上限 70B 使用 1.4T tokens 训练了模型 Chinchilla,在多个下游任务上的性能都超过了 Gopher。
Recommend
About Joyk
Aggregate valuable and interesting links.
Joyk means Joy of geeK