

"Traditional software is deterministic, whereas machine learning is probabi...
source link: https://devm.io/machine-learning/aporia-ml-observability-platform
Go to the source link to view the article. You can view the picture content, updated content and better typesetting reading experience. If the link is broken, please click the button below to view the snapshot at that time.
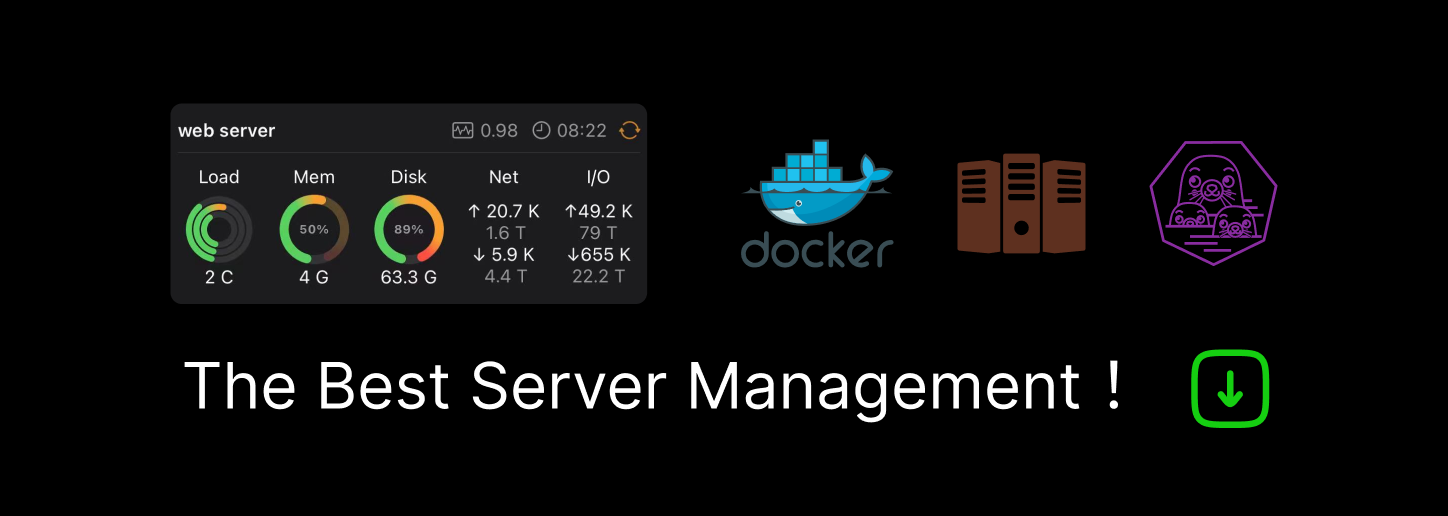
Interview with Liran Hason, CEO and co-founder of Aporia
"Traditional software is deterministic, whereas machine learning is probabilistic"
07. Feb 2023
We had the opportunity to sit down with Liran Hason, the CEO and founder of Aporia, a machine learning observability platform. Established in 2019, Aporia provides data science and ML teams with the tools they need to effectively monitor, explain, and gain insights from their models in production, and has received support from notable investors including Tiger Global, Samsung Next, TLV Partners, and Vertex Ventures.
devmio: AI and Machine learning are being applied across a variety of industries. What are the main challenges organizations face while implementing their AI initiatives?
Liran Hason: There are many challenges to overcome to ensure AI/ML can be adopted with confidence across industries:
- Lack of skilled AI and machine learning personnel: Just imagine trying to build a skyscraper with a hammer and some nails. Building and implementing AI and machine learning systems requires specialized skills, which can be difficult to find in the market. This can make it challenging for organizations to build and maintain an in-house team of ML experts.
- Data quality and availability: Machine learning models rely on large amounts of high-quality data for training and operation. However, many organizations struggle to collect and clean the data needed for their AI initiatives, which can hinder their ability to achieve the desired results.
- Integration with existing systems: ML systems often need to be integrated with other systems and processes within an organization, which can be a complex and time-consuming task.
- Explainability and bias: ML models are black-box by nature and can be difficult to understand and explain, which can make it challenging for organizations to build trust in the technology. Additionally, models can sometimes exhibit bias, which can lead to unfair or inaccurate results.
- Ethical concerns: As machine learning becomes more advanced and widespread, there are growing concerns about its potential impact on society and the ethical implications of their use. This can make it difficult for organizations to navigate the ethical landscape and ensure that their AI initiatives are aligned with their values.
devmio: How is monitoring ML models different from traditional software application monitoring?
Liran Hason: The main difference is that traditional software is deterministic, whereas machine learning is probabilistic. Another key difference is the input data that the models are trained on. While the input data for traditional software applications is usually well-defined and follows a predictable pattern, the input data for machine learning models can be more varied and may change over time, which can affect the model's performance. To expand on my first point – traditional software applications have a fixed set of functions and a predictable flow of control, while machine learning models can exhibit dynamic behavior and change their output based on the input data. This can make it more difficult to monitor and understand their behavior. Other differences include the performance metrics used to evaluate the models, the need for continuous evaluation, and the explainability of the models. Traditional software applications are typically easier to interpret and debug, while machine learning models can be more complex.
devmio: How does Aporia work?
Liran Hason: Aporia is the machine learning observability platform, trusted by Fortune500 and industry leaders to visualize, monitor and maximize the value of their machine learning models in production. It addresses many challenges unique to machine learning models, such as the need to continuously evaluate and compare models, the complexity of interpreting performance metrics, and the difficulty of understanding and debugging dynamic behavior.
Our platform is highly intuitive and flexible, allowing every ML stakeholder to gain insights that are valuable to them. Integration takes less than 5 minutes. With Aporia, you can automate or customize automation to perform ML critical tasks instead of hiring, training, or repurposing valuable personnel to maneuver the complexities of model observability.
Regarding the monitoring of quality model production data and ease of integration, Aporia provides an industry-first solution with Direct Data Connectors, which eliminates the drawbacks and pitfalls of sampling and duplicating data (the current market practice). By integrating in less than 5 minutes and monitoring production data directly from your database, Aporia centralizes model management and ensures no data discrepancies, keeps your data yours and secure, while providing advanced monitoring for all your production models. With Aporia’s single source of truth data reliability, ML models can maximize their value and provide business impact.
Aporia includes a range of features designed to help organizations understand and explain the inner workings of their ML models, as well as to identify and address any potential bias. This includes tools for visualizing and analyzing model behavior, as well as for evaluating and validating models against various datasets. Aporia’s Explainable AI toolbox enables data science and ML professionals to easily explain predictions in human-readable text, highlight feature impact, and simulate ‘what if’ scenarios to help root out potential bias outcomes.
Key for practicing responsible AI, Aporia enables organizations to act upon their ethical AI initiatives by first ensuring that their models work as they intended, catch bias, and drive value. Only then can businesses truly start thinking from an ethical point of view.
devmio: What industries can benefit from using Aporia’s platform?
Liran Hason: Any and all industries employing machine learning in a production environment can benefit from Aporia’s ML observability platform to gain valuable insights into model performance and maximize.
Aporia is use case agnostic out of the box, supporting both models that create and capture value. From recommender and ranking systems, dynamic pricing, NLP, and credit risk models to lead scoring, churn prediction, demand forecasting, and fraud detection models.
AI/ML is being adopted across industries, from healthcare and financial institutions to large manufacturing, ecommerce/retail, insurance, entertainment and sports, car rental, and others employing AI products.
devmio: How is Aporia different from other solutions in the market?
Liran Hason: Aporia is the only ML observability platform to provide organizations with a way to monitor model production data at scale, directly from their own data source. In today’s market, many machine learning monitoring solutions provide an SDK integration which is cumbersome and leads to increased cloud computing spend and generally takes a long time to integrate. Additionally, current monitoring solutions require data sampling or duplication which puts production data monitoring quality at risk. Through Aporia’s Direct Data Connectors (DDC), organizations can simply monitor their model output with zero risk of losing their production data, or skewing production data due to data sampling and duplication, all while reducing cloud costs. Aporia empowers ML teams with a single source of truth to ensure their production data is reliable and drives business impact and integration is done in less than 5 minutes.
With Aporia’s unparalleled customization ML stakeholders are able to tailor performance visibility dashboards and model monitors to their specific needs. This allows our users the flexibility they need to get the most out of their complex models and see how they shine in production.
devmio: What’s next for Aporia?
Liran Hason: 2022 was an amazing year – we closed the largest Series A in the ML Observability market, added thousands of new users, more than doubled our roster, and expanded our US presence. We are looking to build on our successful year to continue growing and adding top talent to the Aporia team. So, if you think outside the box and have a passion to make a product that changes lives, check out our openings and drop us a line.
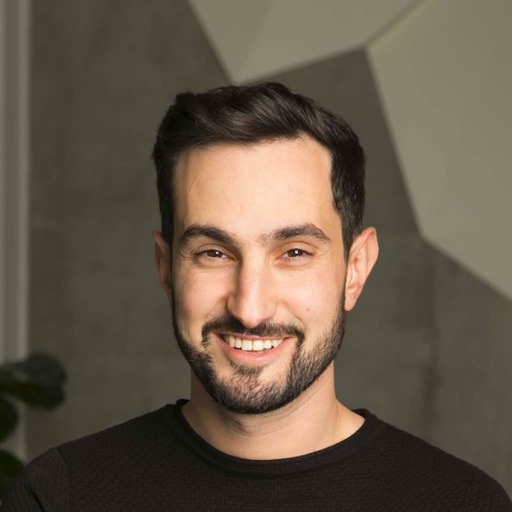
Liran Hason, the founding CEO of Aporia, is a veteran of the IDF's elite 81 intelligence unit. He was one of the first employees of Adallom (acquired by Microsoft), where he led the ML production architecture, serving millions of users. Before starting Aporia, Hason was part of Vertex Ventures' investment team, and was involved in over 30 investments including Axonius, Spot.io, and others.
Recommend
About Joyk
Aggregate valuable and interesting links.
Joyk means Joy of geeK