

[2211.10887] Differential Privacy from Locally Adjustable Graph Algorithms: $k$-...
source link: https://arxiv.org/abs/2211.10887
Go to the source link to view the article. You can view the picture content, updated content and better typesetting reading experience. If the link is broken, please click the button below to view the snapshot at that time.
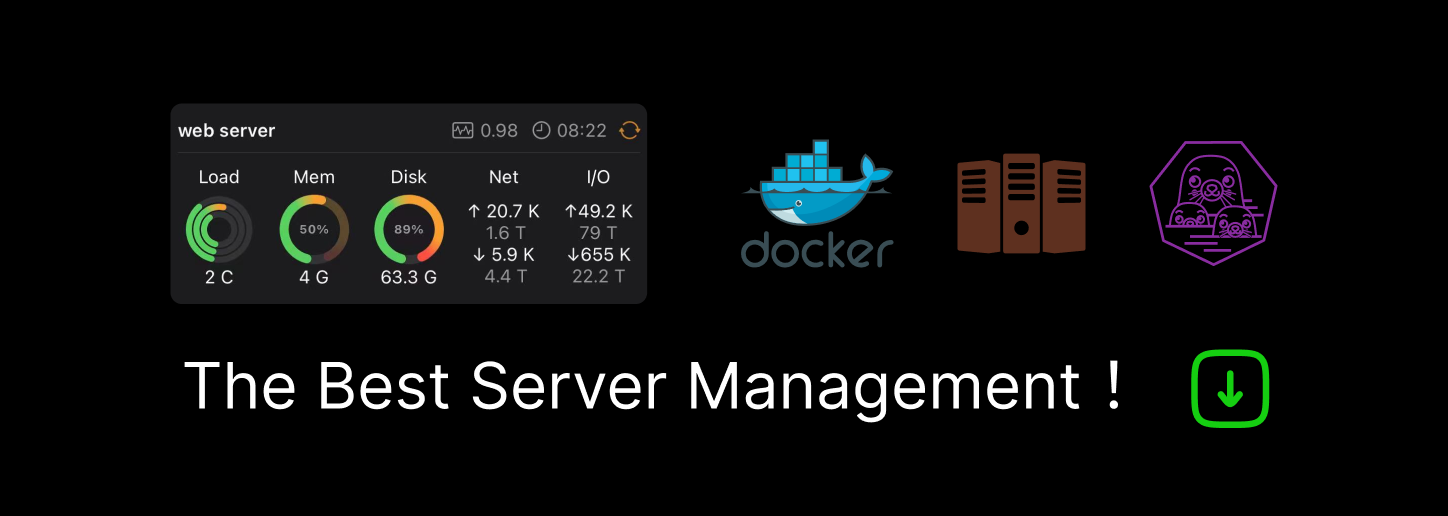
Computer Science > Data Structures and Algorithms
[Submitted on 20 Nov 2022]
Differential Privacy from Locally Adjustable Graph Algorithms: k-Core Decomposition, Low Out-Degree Ordering, and Densest Subgraphs
Differentially private algorithms allow large-scale data analytics while preserving user privacy. Designing such algorithms for graph data is gaining importance with the growth of large networks that model various (sensitive) relationships between individuals. While there exists a rich history of important literature in this space, to the best of our knowledge, no results formalize a relationship between certain parallel and distributed graph algorithms and differentially private graph analysis. In this paper, we define \emph{locally adjustable} graph algorithms and show that algorithms of this type can be transformed into differentially private algorithms.
Our formalization is motivated by a set of results that we present in the central and local models of differential privacy for a number of problems, including k-core decomposition, low out-degree ordering, and densest subgraphs. First, we design an \varepsilon-edge differentially private (DP) algorithm that returns a subset of nodes that induce a subgraph of density at least \frac{D^*}{1+\eta} - O\left(\text{poly}(\log n)/\varepsilon\right), where D^* is the density of the densest subgraph in the input graph (for any constant \eta > 0). This algorithm achieves a two-fold improvement on the multiplicative approximation factor of the previously best-known private densest subgraph algorithms while maintaining a near-linear runtime.
Then, we present an \varepsilon-locally edge differentially private (LEDP) algorithm for k-core decompositions. Our LEDP algorithm provides approximates the core numbers (for any constant \eta > 0) with (2+\eta) multiplicative and O\left(\text{poly}\left(\log n\right)/\varepsilon\right) additive error. This is the first differentially private algorithm that outputs private k-core decomposition statistics.
Subjects: | Data Structures and Algorithms (cs.DS) |
Cite as: | arXiv:2211.10887 [cs.DS] |
(or arXiv:2211.10887v1 [cs.DS] for this version) | |
https://doi.org/10.48550/arXiv.2211.10887 |
Recommend
About Joyk
Aggregate valuable and interesting links.
Joyk means Joy of geeK