

[2209.14375] Improving alignment of dialogue agents via targeted human judgement...
source link: https://arxiv.org/abs/2209.14375
Go to the source link to view the article. You can view the picture content, updated content and better typesetting reading experience. If the link is broken, please click the button below to view the snapshot at that time.
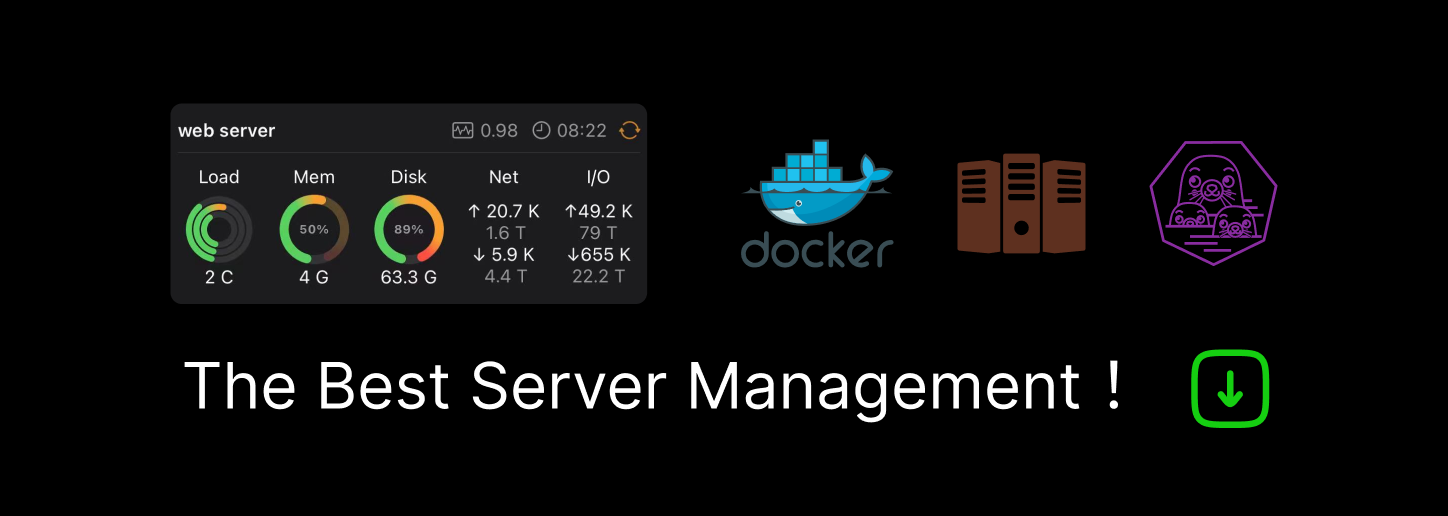
[Submitted on 28 Sep 2022]
Improving alignment of dialogue agents via targeted human judgements
We present Sparrow, an information-seeking dialogue agent trained to be more helpful, correct, and harmless compared to prompted language model baselines. We use reinforcement learning from human feedback to train our models with two new additions to help human raters judge agent behaviour. First, to make our agent more helpful and harmless, we break down the requirements for good dialogue into natural language rules the agent should follow, and ask raters about each rule separately. We demonstrate that this breakdown enables us to collect more targeted human judgements of agent behaviour and allows for more efficient rule-conditional reward models. Second, our agent provides evidence from sources supporting factual claims when collecting preference judgements over model statements. For factual questions, evidence provided by Sparrow supports the sampled response 78% of the time. Sparrow is preferred more often than baselines while being more resilient to adversarial probing by humans, violating our rules only 8% of the time when probed. Finally, we conduct extensive analyses showing that though our model learns to follow our rules it can exhibit distributional biases.
Subjects: | Machine Learning (cs.LG); Computation and Language (cs.CL) |
Cite as: | arXiv:2209.14375 [cs.LG] |
(or arXiv:2209.14375v1 [cs.LG] for this version) | |
https://doi.org/10.48550/arXiv.2209.14375 |
Recommend
About Joyk
Aggregate valuable and interesting links.
Joyk means Joy of geeK