

Minimal character-level language model with a Vanilla Recurrent Neural Network,...
source link: https://gist.github.com/karpathy/d4dee566867f8291f086
Go to the source link to view the article. You can view the picture content, updated content and better typesetting reading experience. If the link is broken, please click the button below to view the snapshot at that time.
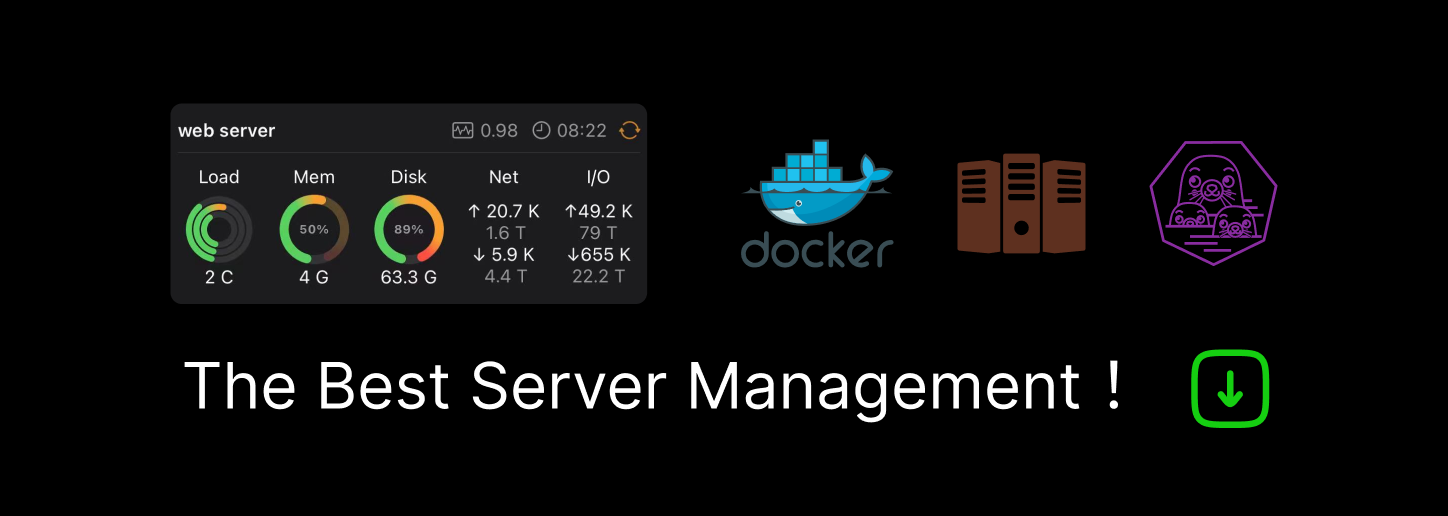
Minimal character-level language model with a Vanilla Recurrent Neural Network, in Python/numpy · GitHub
Instantly share code, notes, and snippets.
How would you use the new biases (Wxh, Whh, Why, bh, by) in the program in order to have the finished product? I save the arrays to a .npz and load them again, but I receive the same amount of loss and similar samples of random characters as I did from starting the first time.
I'm running the python version of this and its not saving the training, when I stop the program there is no memory of all the millions of cycles we completed. Is there a "save the results" function I am missing?
I need help with ----- loss += -np.log(ps[t][targets[t],0]) ----.
dont understand the syntax. looked it up on google but no solution. Is there any other way around.
does sum(-np.log(ys[t])*<one hot array of targets[t]>) mean the same thing?
Also is the same loss_func applicable to python 3 too? (apart from changing the xrange to range)
Thanks very much for your code. It is the code that I can understand the RNN more deeply. I wander that what dose the code of "# prepare inputs (we're sweeping from left to right in steps seq_length long)" mean. I have read your blog http://karpathy.github.io/2015/05/21/rnn-effectiveness/. and test the very simple example "hello". I would be very appreiciate if I could receive your anwser.
That is not code, but rather a one-line comment. Same with anything else starting with the #
character.
data = open("C:\Users\Amir\Desktop\Serious Programming\Neural Networks\Training Data.txt", 'r')
^
SyntaxError: (unicode error) 'unicodeescape' codec can't decode bytes in position 2-3: truncated \UXXXXXXXX escape
C:\Users\Amir\Desktop\Serious Programming\Neural Network\Training Data.txt
should be C\:\\Users\\Amir\\Desktop\\Serious Programming\\Neural Network\\Training Data.txt
as to escape the :
and the backslashes. \Users
is interpreted as Unicode character with code 'sers'
which, "sers" is not a valid code. The :
will act as a special character before the backslash and will also need escaping.
guys, could you tell me please where can I find the output of this code?
Rumi4 commented Jul 15, 2019
does the program generate new text or the one same from the input file
pzdkn commented Jul 20, 2019
Stupid question : In which lines does the vanishing gradient problem manifest itself ?
I didn't see wheren or when to stop or break "the while True:" loop?
I have over 630k iterations and my loss is 42.9 I'm using shakespears poems as training data am I doing something wrong?
mkffl commented Sep 22, 2019
To anyone finding the code hard to understand, I provide detailed explanations here: https://mkffl.github.io/
Hope it helps
@karpathy thanks a lot for this posting! I tried to run it with your hello example ... and this is what I get
Traceback (most recent call last):
File "min-char-rnn.py", line 100, in
loss, dWxh, dWhh, dWhy, dbh, dby, hprev = lossFun(inputs, targets, hprev)
File "min-char-rnn.py", line 43, in lossFun
loss += -np.log(ps[t][targets[t],0]) # softmax (cross-entropy loss)
IndexError: list index out of rangeAny ideas?
Thanks!
This is only working for a file with at least a few sentences. I think the reason is that RNN works with a large dataset. For the smaller datasets, we don't need to use it.
Thanks for your sharing! It helps a lot!
I switched numpy to cupy and when I run this code at iteration 100 I get the following error that is caused by something turning into Nan during training. What can I do to fix this?Traceback (most recent call last):
File "C:\...\hello.py", line 168, in <module>
sample_ix = sample(hprev, inputs[0], 100)
File "C:\...\hello.py", line 130, in sample
ix = int(np.random.choice(range(vocab_size), (1, 1), p=p))
File "C:\...\cupy\random\sample.py", line 196, in choice
return rs.choice(a, size, replace, p)
File "C:\...\cupy\random\generator.py", line 982, in choice
raise ValueError('probabilities are not non-negative')
ValueError: probabilities are not non-negative
I want the paython code of neurel network where: input layer part is composed of two neurons, . The hidden layer is constituted of two under-layers of 20 and 10 neurons for the first under-layer and the second under-layer respectively. The output layer is composed of 5 neurons.
Traceback (most recent call last):
File "minimal_rnn.py", line 100, in
loss, dWxh, dWhh, dWhy, dbh, dby, hprev = lossFun(inputs, targets, hprev)
File "minimal_rnn.py", line 43, in lossFun
loss += -np.log(ps[t][targets[t],0]) # softmax (cross-entropy loss)
IndexError: list index out of range
Why am I getting this error?
Can somebody explain why we need to multiply 0.01 when initializing the weights ? Arent we already using np.random.randn to sample from a normal distribution?
I am talking about this specific line of code
Wxh = np.random.randn(hidden_size, vocab_size)*0.01
@MistryWoman Its essential to break the symmetry here. Since the outputs of the succeeding weights depends on the sum of inputs multiplied by the corresponding weight. If all weights are assigned to zero, every hidden unit will get same the type of signal ie zero in this case. No matter what was the input - if all weights are the same, all units in hidden layer will be the same too. This is not something we desire because we want different hidden units to compute different functions. However, this is not possible if you initialize all to the same value.
Wonderful code,but there are somecodes confused me.
# perform parameter update with Adagrad for param, dparam, mem in zip([Wxh, Whh, Why, bh, by], [dWxh, dWhh, dWhy, dbh, dby], [mWxh, mWhh, mWhy, mbh, mby]): mem += dparam * dparam# 梯度的累加 param += -learning_rate * dparam / np.sqrt(mem + 1e-8) # adagrad update
Wxh,..mby were defined as global variables,while param,dparam,mem are just local variables.How could Adagrad update change the value of global variables?I tried to test my thought by code like below.
import numpy as np Wxh, Whh, Why, bh, by=1,2,3,4,5 dWxh, dWhh, dWhy, dbh, dby=1,2,3,4,5 mWxh, mWhh, mWhy, mbh, mby=1,2,3,4,5 while True: for param, dparam, mem in zip([Wxh, Whh, Why, bh, by], [dWxh, dWhh, dWhy, dbh, dby], [mWxh, mWhh, mWhy, mbh, mby]): mem += dparam * dparam# 梯度的累加 param += dparam / np.sqrt(mem + 1e-8) print(Wxh)#Output never change!
It's might be a very simple problem.Just puzzled me!Someone helps?Thanks a lot.
Not sure if anyone ever addressed this further down the thread but I found an answer on stack overflow that addresses modifying mutable global variables like Wxh and the like within a local scope.
'param' is referencing all the weight arrays rather than copying their values in their original locations. It is not copying them into a new storage location. So when adding to all the values in 'param' with adagrad we are actually modifying the global values of Wxh, etc... not the values in param. I think?
@MistryWoman We are trying to push our weights closer to zero, so that gradient doesn't vanish. For large weights , gradients become very small while using tanh. Please take a look at https://gist.github.com/karpathy/d4dee566867f8291f086#gistcomment-2180482
@karpathy Thank you for posting this. I had a small doubt in line 58. Why do we perform Whh.T
in np.dot(Whh.T,dhraw)
? Since Whh
has (hidden_size,hidden_size)
as shape, we can directly multiply it with dhraw
. Or am I missing something here?
Traceback (most recent call last):
File "minimal_rnn.py", line 100, in
loss, dWxh, dWhh, dWhy, dbh, dby, hprev = lossFun(inputs, targets, hprev)
File "minimal_rnn.py", line 43, in lossFun
loss += -np.log(ps[t][targets[t],0]) # softmax (cross-entropy loss)
IndexError: list index out of rangeWhy am I getting this error?
I was getting the same error but it seems like the reason is that your input file is too small. Try writing more stuff in your input.txt
I made a two layer recurrent neural network based off of this and I am not sure why it does not work. So, if anyone could check out and make a PR if you find a problem?
https://github.com/lanttu1243/vanilla_recurrent_neural_network.git
Hi, can anyone explain the line where we pass in the gradient through the softmax function " dy [targets[t]] -=1 ". Why are we doing this operation ??
To anyone finding the code hard to understand, I provide detailed explanations here: https://mkffl.github.io/ Hope it helps
Good explanation for second part of lossFunction.
Sign up for free to join this conversation on GitHub. Already have an account? Sign in to comment
Recommend
About Joyk
Aggregate valuable and interesting links.
Joyk means Joy of geeK