

OneFlow源码解析:自动微分机制
source link: https://blog.csdn.net/OneFlow_Official/article/details/128108109
Go to the source link to view the article. You can view the picture content, updated content and better typesetting reading experience. If the link is broken, please click the button below to view the snapshot at that time.
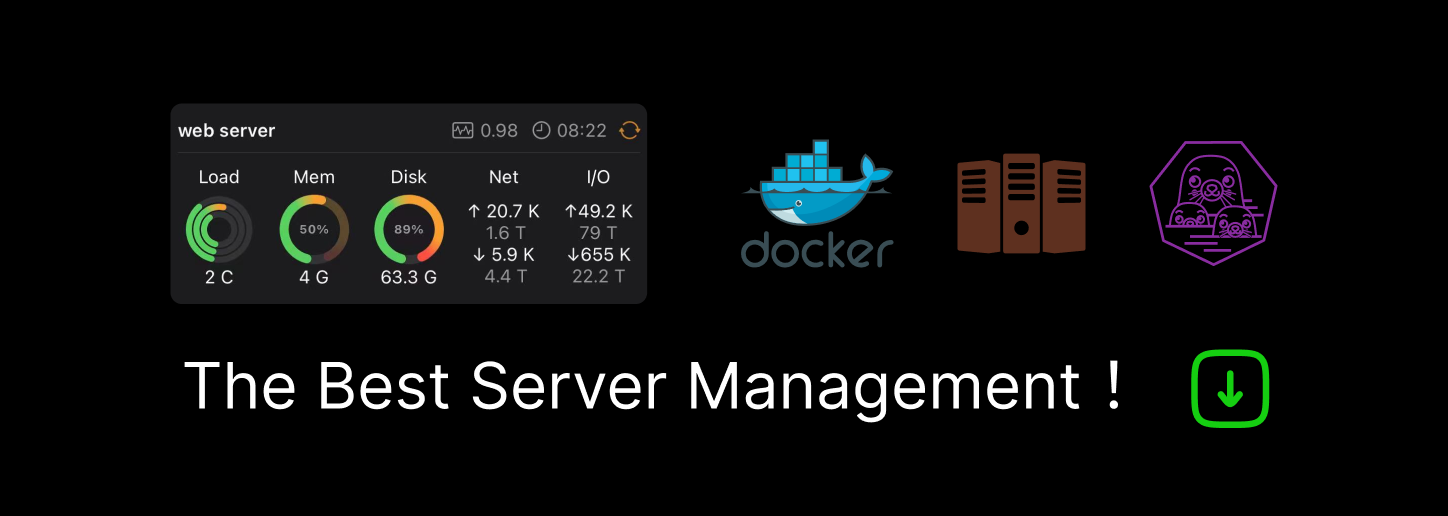
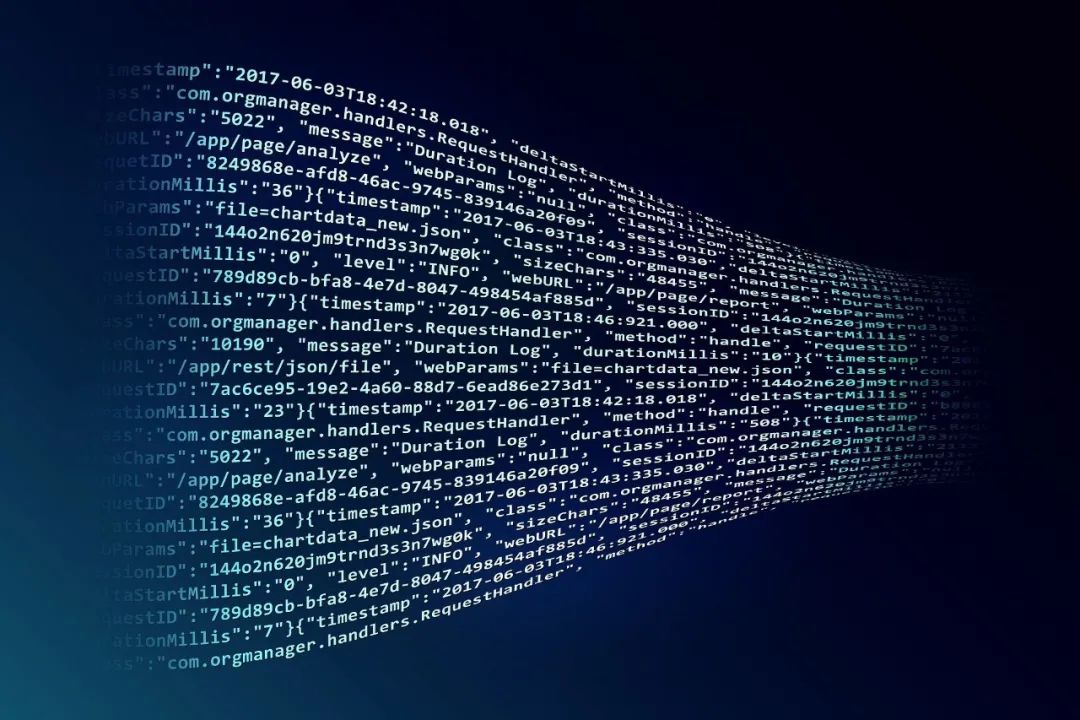
撰文 | 郑建华
更新|赵露阳、王迎港
深度学习框架一般通过自动微分(autograd)机制计算梯度并反向传播。本文尝试通过一个简单的例子,粗浅地观察一下OneFlow的autograd的实现机制。
1
自动微分基础
自动微分相关的资料比较多,个人感觉自动微分的原理介绍(https://mp.weixin.qq.com/s/BwQxmNoSBEnUlJ1luOwDag)这个系列及其引用的资料对相关背景知识的介绍比较完整清晰。
下面分几种情况对梯度传播的原理做一些直观解释。
1.1 stack网络的梯度传播
以x -> f -> g -> z这个stack网络为例,根据链式法则:
∂z/∂x = ∂z/∂g * ∂g/∂f * ∂f/∂x
实际运行时,在梯度反向传播过程中:
-
z将∂z/∂g传给g。
-
如果节点g有权重w需要计算梯度,就计算∂z/∂w = ∂z/∂g * ∂g/∂w。
-
g需要计算∂g/∂f,再乘以z传过来的梯度,将结果传给f。g只需要给f传递链式乘积的结果,不需要传递各项明细。
-
在训练阶段的前向计算时,g需要保存∂g/∂f计算依赖的中间结果、以供反向计算时使用。
-
其它节点的传播情况依次类推。
1.2 简单graph的梯度传播
以下面这个简单的graph拓扑为例。
在继续之前,需要了解一下多元复合函数微分的基本公式。
下图中,u和v都是关于x和y的函数,z是关于u和v的函数。
根据这个公式可以知道,z对x的梯度分别沿两条链路传播,z -> u -> x和z -> v -> x,节点x将两个梯度之和作为z对x的梯度。
1.3 复杂graph的梯度传播
再看一个拓扑稍微复杂点的例子:

上图可以视为x -> U -> L,其中U是e -> ... -> h的子图。f -> g的子图可以视为V。
对于节点h来说,它需要把梯度传给g和k。对节点e来说,它需要对f和k传来的梯度求和,才是∂L/∂e。这样,L对x的梯度,仍可以按链路拆解,一条链路前后节点间的梯度是乘积关系,传入的多条链路梯度是加和关系。
这篇博客(https://blog.paperspace.com/pytorch-101-understanding-graphs-and-automatic-differentiation/)中有一个几乎一样的拓扑图,给出了部分权重参数的梯度公式。
2
autograd中tensor相关的一些基本概念
2.1 叶子节点
OneFlow的autograd文档(https://docs.oneflow.org/en/master/basics/05_autograd.html)中介绍了leaf node和root node的概念。只有输出、没有输入的是leaf node,只有输入、没有输出的是root node。
个人理解,如果把weight、bias、data视为计算图的一部分,这些节点就是叶子节点(op不是叶子节点)。尤其是从反向计算图的视角(https://discuss.pytorch.org/t/what-is-the-purpose-of-is-leaf/87000/9)看,这些节点的grad_fn是空,反向传播到这些节点就会停止。
is_leaf和requires_grad有比较密切的关系,但二者又是独立的。PyTorch是这样解释的:(https://pytorch.org/docs/stable/generated/torch.Tensor.is_leaf.html#torch.Tensor.is_leaf)
-
requires_grad=false的节点都是叶子节点。比如data。
-
requires_grad=true的节点如果是用户创建的,也是叶子节点。比如weight和bias。
-
在梯度的反向计算过程中,只有叶子节点的梯度才会被填充。对于非叶子节点,如果要填充梯度信息,需要显式设置retain_grad=true。
-
requires_grad=true才会计算、填充梯度。比如y = relu(x),y是op创建的、不是叶子节点。但如果x需要计算梯度,则y.requires_grad==true。但不需要为y填充梯度。
关于叶子节点这个概念,目前找到的主要是直观描述,还没看到严格、清晰的定义。也可能是因为用户一般不会直接使用is_leaf(https://discuss.pytorch.org/t/what-is-the-purpose-of-is-leaf/87000/9),这个概念只是在阅读代码的时候才会涉及到。
下面的资料可以供进一步参考:
-
What is the purpose of `is_leaf`? (https://discuss.pytorch.org/t/what-is-the-purpose-of-is-leaf/87000)
-
叶子节点和tensor的requires_grad参数(https://zhuanlan.zhihu.com/p/85506092)
2.2 tensor detach
Tensor的detach方法(https://github.com/Oneflow-Inc/oneflow/blob/48e511e40e09551408c96722c09bd061ce320687/oneflow/core/framework/tensor_impl.cpp#L155)会创建一个新的tensor,新tensor的属性中
-
requires_grad = false
-
is_leaf = true
detach的意思是从grad的反向计算图中把tensor分离出来。新的tensor与原来的对象共享存储,但不参与反向图的拓扑构造。原有对象的requires_grad属性不变。
比如下面的代码,修改一个对象的数据,另一个对象的数据也会改变。
3
示例代码
本文通过如下代码来观察OneFlow的autograd机制。
y.backward方法有两种接口:
-
如果y是一个标量(比如loss),不需要传递任何参数。
-
如果y是一个向量,需要传入一个与y的shape一致的向量作为参数。
为什么会有这种区别呢?下面几篇参考资料中对这个问题做了比较详细的解释。简单的说:
-
如果函数的输出是向量,在反向传播的过程中会造成梯度tensor shape的维度膨胀,实现复杂、性能差。
-
如果函数的输出是标量,反向传播梯度tensor的shape与参数变量的shape一致,不会出现维度膨胀,更容易实现。
-
对于向量版本的backward,可以假想存在某个loss函数,backward的参数是loss传播到y这里的梯度。因为前后节点间的梯度是乘积关系,所以用ones替代这个假想的梯度,这样计算结果x.grad就是y对x的梯度。
后续将以y.backward(flow.Tensor([1, 1]))为例观察一下autograd的机制。其反向图只有x <- y这一步。
-
自动求梯度 (https://tangshusen.me/Dive-into-DL-PyTorch/#/chapter02_prerequisite/2.3_autograd?id=_233-梯度)
-
PyTorch 的 backward 为什么有一个 grad_variables 参数?(https://zhuanlan.zhihu.com/p/29923090)
3.1 梯度结果的存储
Tensor的grad属性(https://github.com/Oneflow-Inc/oneflow/blob/48e511e40e09551408c96722c09bd061ce320687/oneflow/api/python/framework/tensor.cpp#L611),在读取值时调用的是acc_grad()方法(acc应该是accumulate的缩写)。这样就知道梯度实际存储在哪里,读代码时可以重点关注相关部分。
调用流程如下:
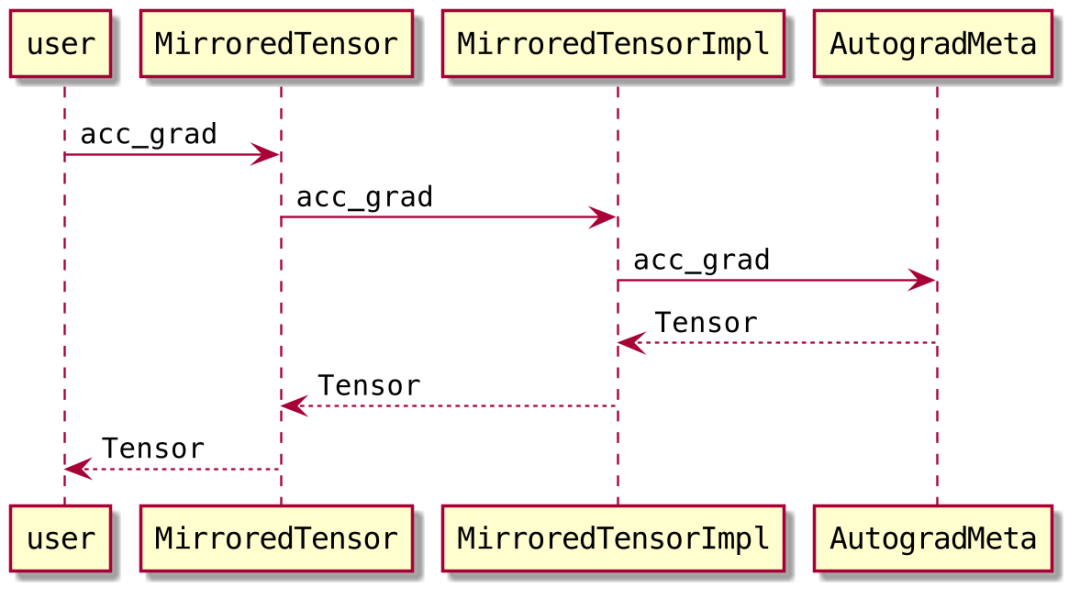
注:图片中的MirroredTensor在最新源码中,已经更名为LocalTensor,其实是一样的。
4
autograd相关的类图关系
下图展示了autograd相关类的关系
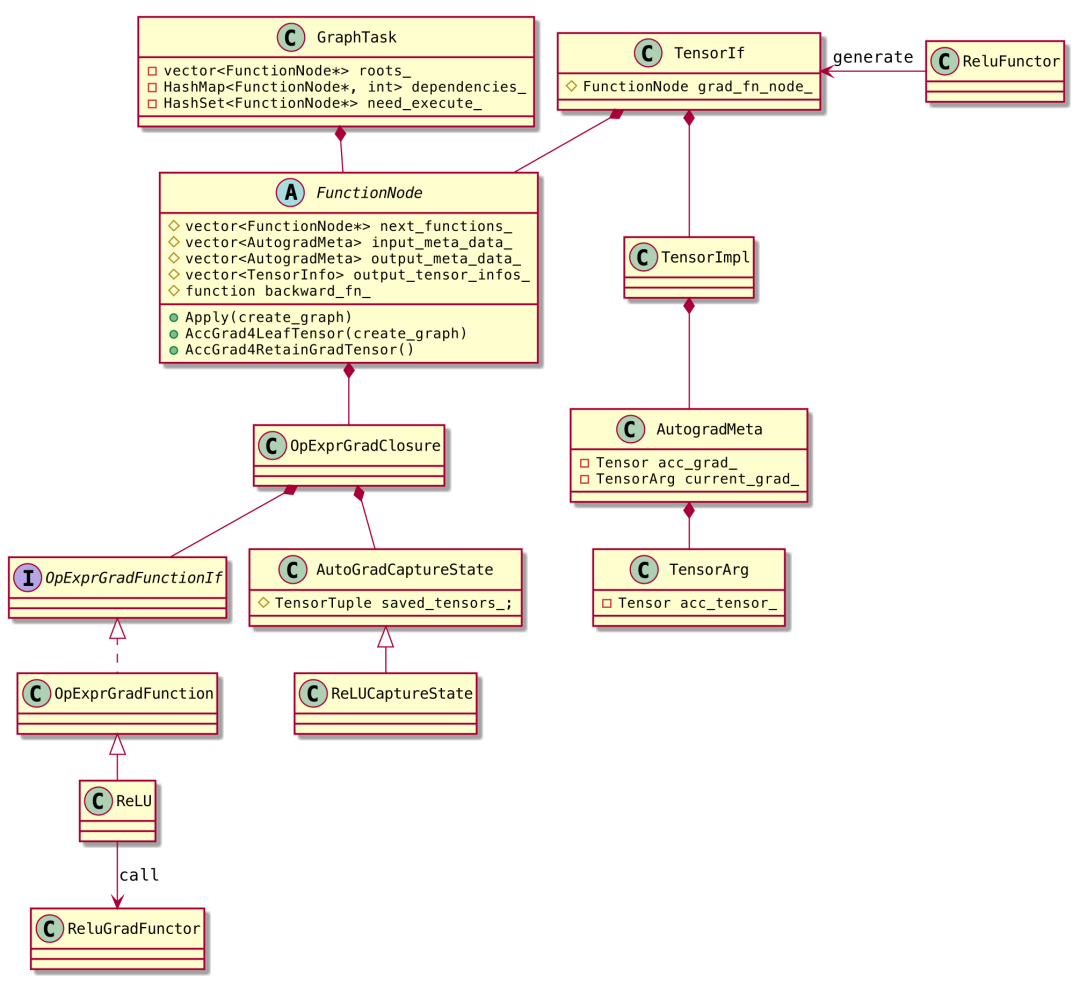
在看autograd代码之前,可以参照这个类图,了解其中的结构和关系,有助于理解代码中各个部分的作用。
在eager模式下,用户通过op的组合逐步构建出前向计算图。在执行前向计算的过程中,引擎会为autograd需要的反向计算图记录必要的信息,在调用backward方法时执行这个反向计算图。
对照上面的类图
-
站在tensor的视角
-
-
前向op输出一个tensor y,即TensorIf <- ReluFunctor这部分。
-
从y可以找到反向计算图实际执行梯度计算的类,即TensorIf -> FunctionNode -> ReLU这个链路。
-
FunctionNode的backward_fn_包含了OpExprGradClosure。它只负责计算当前节点的梯度。
-
ReLU是执行梯度计算的类,它会调用ReluGradFunctor这个op来执行梯度计算。
-
-
站在反向图存储的视角
-
-
反向图相关的信息在FunctionNode中保存。
-
反向计算图的root是tensor(比如y或loss)的grad_fn_node_变量。
-
FunctionNode的next_functions_表示反向图的下游节点,当前节点把梯度结果传给这些下游节点。这些FunctionNode的连接就构成了反向图的拓扑结构。
-
tensor的梯度存储路径是TensorImpl.AutogradMeta.acc_grad_
-
AutogradMeta.current_grad_是反向图上游传递到当前节点的梯度合计。如果tensor t输入给op u和v,那么u和v反传的梯度会累加到current_grad_。current应该表示截至当前正在计算时的累加和。
-
FunctionNode虽然并不持有tensor实例,但它持有tensor的AutogradMeta成员变量指针。
-
基于上述relu的例子中的节点y
-
output_meta_data_即y.autograd_meta_
-
input_meta_data_即x.autograd_meta_
-
所以FunctionNode能获取到上下游的梯度数据并进行读写
-
AutoGradCaptureState可以存储一些梯度计算需要的状态信息,比如计算relu的梯度时需要用到它的前向输出结果y。
-
-
站在反向图执行的视角
-
-
GraphTask负责反向图的执行。
-
FunctionNode只保存必要的数据。
-
GraphTask基于这些数据,自己构造遍历需要的数据结构,遍历所有节点、执行梯度计算。
-
5
前向计算过程中为autograd所做的准备
反向图的执行过程是数据驱动的,数据的存储结构和内容决定了执行的具体动作。
以下讨论只针对eager模式。lazy模式下,反向图的构建是多轮优化passes的一部分(https://github.com/Oneflow-Inc/oneflow/blob/48e511e40e09551408c96722c09bd061ce320687/oneflow/core/framework/op_interpreter/op_interpreter.cpp#L98)。
之前在讨论Op、Kernel与解释器时已经了解Interpreter的作用。只是当时重点关注op的执行,忽略了grad相关的内容。
GetInterpreter(https://github.com/Oneflow-Inc/oneflow/blob/48e511e40e09551408c96722c09bd061ce320687/oneflow/core/framework/op_interpreter/op_interpreter_util.cpp#L67)返回的其实是一个AutogradInterpreter对象(https://github.com/Oneflow-Inc/oneflow/blob/48e511e40e09551408c96722c09bd061ce320687/oneflow/core/framework/op_interpreter/op_interpreter_util.cpp#L42),在它的Apply方法中(https://github.com/Oneflow-Inc/oneflow/blob/48e511e40e09551408c96722c09bd061ce320687/oneflow/core/framework/op_interpreter/op_interpreter.cpp#L86),调用内嵌Interpreter的同时,也会记录grad计算需要的信息。
AutogradInterpreter::Apply的主要流程如下:
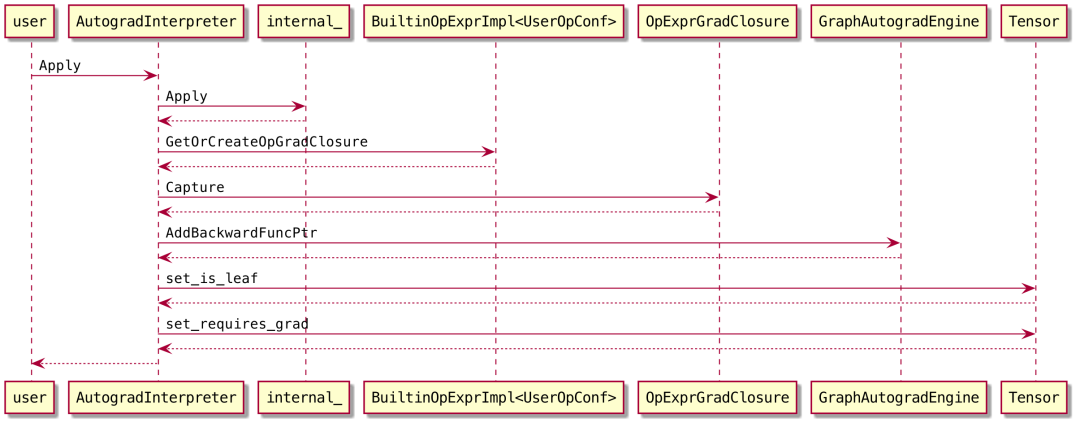
Apply的第一步会先计算requires_grad。只要op的任一输入的requires_grad为true,op的输出的requires_grad也为true(https://github.com/Oneflow-Inc/oneflow/blob/48e511e40e09551408c96722c09bd061ce320687/oneflow/core/framework/op_interpreter/op_interpreter.cpp#L151-L152)(前提是输出的数据类型支持梯度)。y的requires_grad就是在这里决定的。
比如y = relu(x),如果数据类型支持梯度,y.requires_grad就等于x.requires_grad。
然后会调用内嵌的解释器internal_执行相关计算。在调用内嵌解释器期间,会临时禁止梯度模式,比如有些op可能会嵌套、多次调用解释器(ReluGradFunctor也会通过解释器执行),这些都不需要梯度逻辑。
需要说明的是,构造x时不会执行grad相关的逻辑,因为inputs的requires_grad都是false,x的requires_grad是在构造的最后才设置的(https://github.com/Oneflow-Inc/oneflow/blob/48e511e40e09551408c96722c09bd061ce320687/oneflow/api/python/utils/tensor_utils.cpp#L187)。
下面重点看一下几个核心函数的逻辑细节。
5.1 梯度闭包的构建
前面对类图的说明中已经提到,OpExprGradClosure只负责当前节点的梯度计算。
GetOrCreateOpGradClosure函数(https://github.com/Oneflow-Inc/oneflow/blob/48e511e40e09551408c96722c09bd061ce320687/oneflow/core/framework/op_expr.cpp#L146)的核心代码如下:
NewObj会调用AutoRegistrationFactory(https://github.com/Oneflow-Inc/oneflow/blob/48e511e40e09551408c96722c09bd061ce320687/oneflow/core/common/auto_registration_factory.h#L94)获取预先注册的工厂、创建对象。之前在讨论Op指令在虚拟机中的执行时也看到过类似的注册机制。
这里op_type_name的值是relu,在代码中搜索"relu",可以找到注册ReLU的宏(https://github.com/Oneflow-Inc/oneflow/blob/48e511e40e09551408c96722c09bd061ce320687/oneflow/core/autograd/gradient_funcs/activation.cpp#L562)。宏展开后的代码如下:
static AutoRegistrationFactory<std::string, OpExprGradFunctionIf>::CreatorRegisterTypeg_registry_var4("relu", ([]() { return new ReLU; }));
所以实际返回的对象是ReLU(https://github.com/Oneflow-Inc/oneflow/blob/48e511e40e09551408c96722c09bd061ce320687/oneflow/core/autograd/gradient_funcs/activation.cpp#L200)。其Init函数是个空操作。
OpExprGradClosure只是简单的把ReLU存下来供backward执行时调用。整个调用流程如下:
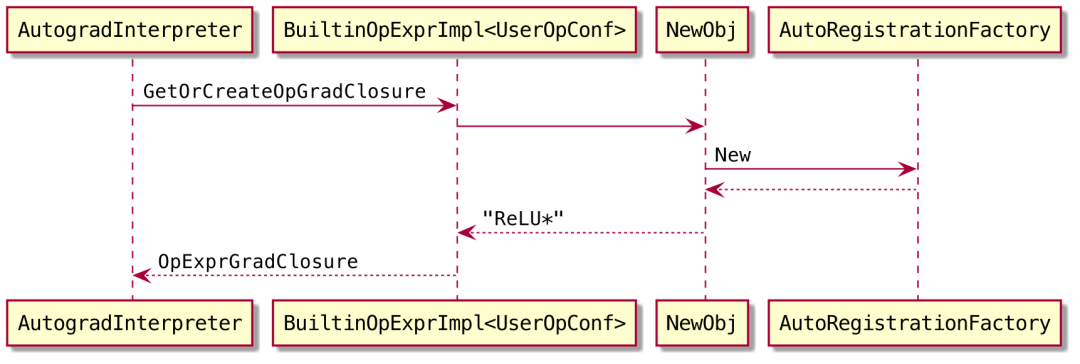
5.2 捕获梯度计算需要的数据
调用流程如下:
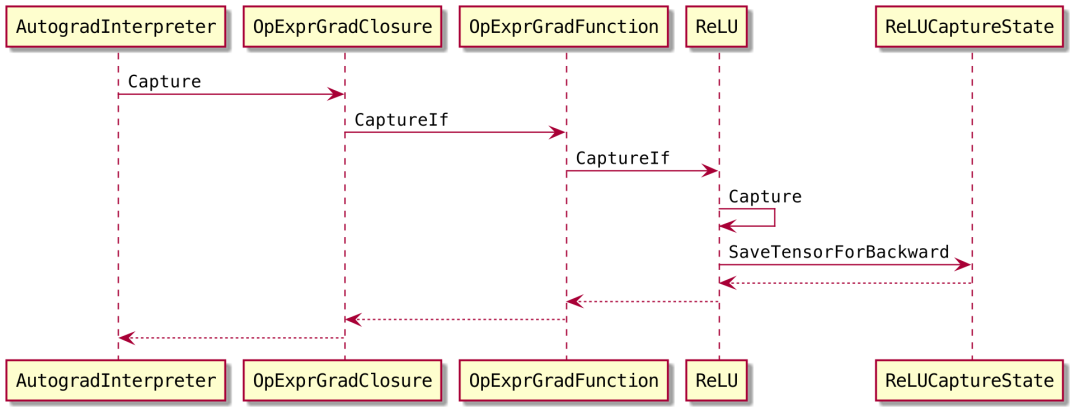
Capture函数(https://github.com/Oneflow-Inc/oneflow/blob/48e511e40e09551408c96722c09bd061ce320687/oneflow/core/framework/op_interpreter/op_interpreter.cpp#L122)的作用就是为后续的梯度计算保存必要的数据。
需要注意的是,OpExprGradFunction::CaptureIf(https://github.com/Oneflow-Inc/oneflow/blob/48e511e40e09551408c96722c09bd061ce320687/oneflow/core/framework/op_expr_grad_function.h#L93)中保存的是detach的tensor。这些tensor与原来的tensor共享数据;可以读写梯度数据,但不会参与反向图的拓扑构造。这个函数把Interpreter传过来的op的detached outputs传给ReLU::Capture(https://github.com/Oneflow-Inc/oneflow/blob/48e511e40e09551408c96722c09bd061ce320687/oneflow/core/framework/op_expr_grad_function.h#L128)(就是relu的前向输出y),ReLU::Capture就把output[0]存到ReLUCaptureState的saved_tensors_中(https://github.com/Oneflow-Inc/oneflow/blob/48e511e40e09551408c96722c09bd061ce320687/oneflow/core/autograd/gradient_funcs/activation.cpp#L209)。因为对于relu来说,根据y就可以计算梯度。
5.3 保存反向图结构信息
AutogradInterpreter::Apply中会构造一个lambada表达式backward_fn(https://github.com/Oneflow-Inc/oneflow/blob/48e511e40e09551408c96722c09bd061ce320687/oneflow/core/framework/op_interpreter/op_interpreter.cpp#L103-L110),其核心逻辑只有一行grad_closure->Apply。
这个lambda的主要作用就是捕获grad_closure这个智能指针。lambda表达式最终会作为FunctionNode的backward_fn_变量。这样才有类图中FunctionNode到OpExprGradClosure这条线,才能从FunctionNode找到closue、执行节点的梯度计算。
GetThreadLocalAutogradEngine()->AddNode这个函数(https://github.com/Oneflow-Inc/oneflow/blob/48e511e40e09551408c96722c09bd061ce320687/oneflow/core/framework/op_interpreter/op_interpreter.cpp#L113)很关键,AddNode的主要任务(https://github.com/Oneflow-Inc/oneflow/blob/48e511e40e09551408c96722c09bd061ce320687/oneflow/core/autograd/autograd_engine.cpp#L478)是为inputs和outputs创建FunctionNode、并保存反向图遍历需要的数据。其输入参数中的inputs/outputs,是前向计算的op的inputs/outputs。对于relu来说,inputs就是x,outputs就是y。
在上述示例代码中,对于x,因为它是叶子节点、也需要梯度,在AddAccumulateFunctionNode会将grad_fn_node设置为一个空操作的函数(https://github.com/Oneflow-Inc/oneflow/blob/48e511e40e09551408c96722c09bd061ce320687/oneflow/core/autograd/autograd_engine.cpp#L508)。之所以是空操作,是因为叶子节点只需要存储梯度、不需要自己计算梯度;它所需要的梯度计算结果会由反向图的上游节点保存到x.autograd_meta_中。
之后会为y构造GraphFunctionNode并形成节点连接(https://github.com/Oneflow-Inc/oneflow/blob/48e511e40e09551408c96722c09bd061ce320687/oneflow/core/autograd/autograd_engine.cpp#L491)、并保存到grad_fn_node(https://github.com/Oneflow-Inc/oneflow/blob/48e511e40e09551408c96722c09bd061ce320687/oneflow/core/autograd/autograd_engine.cpp#L495)。需要注意的是,这里的backward_fn就是AutogradInterpreter::Apply中的lambda表达式(https://github.com/Oneflow-Inc/oneflow/blob/48e511e40e09551408c96722c09bd061ce320687/oneflow/core/framework/op_interpreter/op_interpreter.cpp#L103-L109)。
需要注意的是,AddBackwardFuncPtr中的inputs/outputs是针对op而言,GraphFunctionNode构造函数中同名变量的是针对FunctionNode而言,二者的含义和指向的对象是不一样的。
构造完成后,x和y的grad_fn_node_字段数据内容如下:
x.grad_fn_node_
y.grad_fn_node_
backward就是根据这些数据,从roots出发,完成反向图的遍历。
6
backward的入口
在《OneFlow源码阅读4:tensor类型体系与local tensor》(https://segmentfault.com/a/1190000041989895)中提到过,Tensor类在Python端经过一层包装,通过Python机制为Tensor类注册一些方法,backward就是包装的方法之一。
相关的源代码文件如下
-
python/oneflow/framework/tensor.py
-
python/oneflow/autograd/__init__.py
-
oneflow/python/oneflow/autograd/autograd.py
-
oneflow/api/python/autograd/autograd.cpp
C++的调用流程如下:
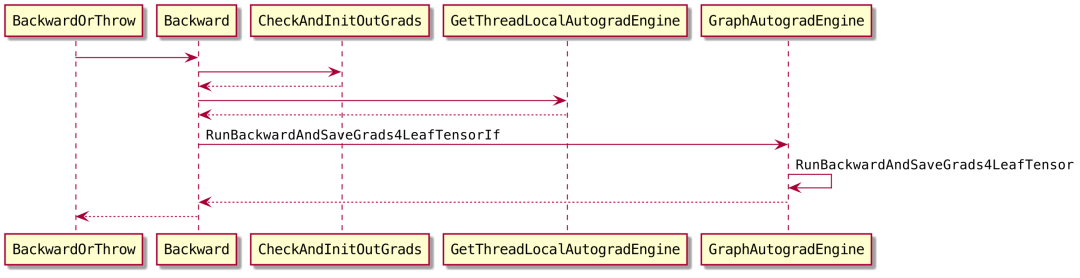
这里重复一下本文使用的示例代码:
上述示例代码执行时,Backward(https://github.com/Oneflow-Inc/oneflow/blob/48e511e40e09551408c96722c09bd061ce320687/oneflow/api/python/autograd/autograd.cpp#L90)的主要参数的值如下:
-
outputs: y, relu输出的tensor
-
out_grads: [1, 1]
CheckAndInitOutGrads(https://github.com/Oneflow-Inc/oneflow/blob/48e511e40e09551408c96722c09bd061ce320687/oneflow/api/python/autograd/autograd.cpp#L49)返回的是loss通过当前op、传到当前节点的梯度。其部分逻辑就是第3节讨论的
-
如果y是一个向量,backward必须传入一个与y的shape一致的向量(https://github.com/Oneflow-Inc/oneflow/blob/48e511e40e09551408c96722c09bd061ce320687/oneflow/api/python/autograd/autograd.cpp#L72-L81)。
-
如果y是一个标量,backward不要参数,框架会自动构造一个全1的tensor(https://github.com/Oneflow-Inc/oneflow/blob/48e511e40e09551408c96722c09bd061ce320687/oneflow/api/python/autograd/autograd.cpp#L70)。
7
反向计算中GraphAutogradEngine的调用流程
反向图计算的流程分析可以结合3类信息
-
上述x和y的grad_fn_node_的值
-
类图以及类之间的关系
RunBackwardAndSaveGrads4LeafTensor(https://github.com/Oneflow-Inc/oneflow/blob/48e511e40e09551408c96722c09bd061ce320687/oneflow/core/autograd/autograd_engine.cpp#L445)函数的几个参数是:
-
outputs: relu的输出y
-
out_grads: 用户自己构造的ones [1, 1]
7.1 反向传递过来的梯度的累加
RunBackwardAndSaveGrads4LeafTensor(https://github.com/Oneflow-Inc/oneflow/blob/release/0.7.0/oneflow/core/autograd/autograd_engine.cpp#L447)函数中,PushPartialTensor(https://github.com/Oneflow-Inc/oneflow/blob/48e511e40e09551408c96722c09bd061ce320687/oneflow/core/autograd/autograd_engine.cpp#L450)的作用就是将loss传过来的梯度累加到autograd_meta_.current_grad_.acc_tensor_。第4节中提到,TensorArg.acc_tensor_存储的就是loss传过来的梯度的合计。这就是roots(即y)接收到的梯度,要么是框架自动创建的ones,要么是用户提供的梯度(通常也是ones)。
这行代码的逻辑可以用如下伪码表示
outputs[i].impl_.autograd_meta_.current_grad_.acc_tensor_ += out_grads[i]
7.2 反向图计算任务的构造与执行
FunctionNode只是记录了反向图的基础信息。RunBackwardAndSaveGrads4LeafTensor中会再构造一个GraphTask对象来表示一次反向计算任务。
-
GraphTask的构造函数(https://github.com/Oneflow-Inc/oneflow/blob/48e511e40e09551408c96722c09bd061ce320687/oneflow/core/autograd/autograd_engine.cpp#L452)主要是初始化反向图的roots_节点,并将图中各个节点的依赖计数dependencies_置为0。根据示例代码,roots_就是y(通常是loss)。
-
ComputeDependencies(https://github.com/Oneflow-Inc/oneflow/blob/48e511e40e09551408c96722c09bd061ce320687/oneflow/core/autograd/autograd_engine.cpp#L321)会对反向图进行深度优先遍历、统计图中各个节点的依赖计数。
-
GraphTask::Apply(https://github.com/Oneflow-Inc/oneflow/blob/48e511e40e09551408c96722c09bd061ce320687/oneflow/core/autograd/autograd_engine.cpp#L405)中实现了反向图的遍历逻辑(传入的save_grad_for_leaf参数是true)。当FunctionNode的依赖为0时,节点才会被放入执行队列(https://github.com/Oneflow-Inc/oneflow/blob/48e511e40e09551408c96722c09bd061ce320687/oneflow/core/autograd/autograd_engine.cpp#L439),后续会对反向图执行按拓扑序遍历。FunctionNode::Apply执行时,它的依赖都执行完毕了。GraphTack::Apply这个函数中,涉及梯度计算逻辑主要包括两部分:
-
-
调用node->Apply执行单个节点的梯度计算(https://github.com/Oneflow-Inc/oneflow/blob/48e511e40e09551408c96722c09bd061ce320687/oneflow/core/autograd/autograd_engine.cpp#L421)
-
调用node->AccGrad4LeafTensor存储算好的梯度(https://github.com/Oneflow-Inc/oneflow/blob/48e511e40e09551408c96722c09bd061ce320687/oneflow/core/autograd/autograd_engine.cpp#L430)
-
7.3 节点的梯度计算
FunctionNode::Apply(https://github.com/Oneflow-Inc/oneflow/blob/48e511e40e09551408c96722c09bd061ce320687/oneflow/core/autograd/autograd_engine.cpp#L187)中,处理output_meta_data_的for循环(https://github.com/Oneflow-Inc/oneflow/blob/48e511e40e09551408c96722c09bd061ce320687/oneflow/core/autograd/autograd_engine.cpp#L195-L205)的核心逻辑可以用如下伪码表示:
从中可以看出来,output_grads的作用就是拷贝上游传过来的梯度数据(指针),作为backward_fn_的参数。
后面可以看到,backward_fn(https://github.com/Oneflow-Inc/oneflow/blob/48e511e40e09551408c96722c09bd061ce320687/oneflow/core/autograd/autograd_engine.cpp#L206)的核心逻辑是:
input_grads就是当前节点传给下游节点的梯度,调用backward_fn时会对它进行赋值。
处理input_meta_data的for循环的核心逻辑(https://github.com/Oneflow-Inc/oneflow/blob/48e511e40e09551408c96722c09bd061ce320687/oneflow/core/autograd/autograd_engine.cpp#L214)可以用如下伪码表示。实质就是将当前节点传给下游节点的梯度,累加到下游节点的current_grad上,从而实现梯度的传播。如果tensor输入给多个op,每个op的梯度会加起来。
input_meta_data_[i].current_grad_.acc_tensor_ += input_grads[i]
7.3.1 梯度计算的执行:backward_fn
以下只考虑前述示例的root节点的执行。也就是y对应的FunctionNode。对于y来说,backward_fn就是AutogradInterpreter::Apply中定义的lambda表达式(https://github.com/Oneflow-Inc/oneflow/blob/48e511e40e09551408c96722c09bd061ce320687/oneflow/core/framework/op_interpreter/op_interpreter.cpp#L103-L110)。
对于relu来说,执行过程如下:
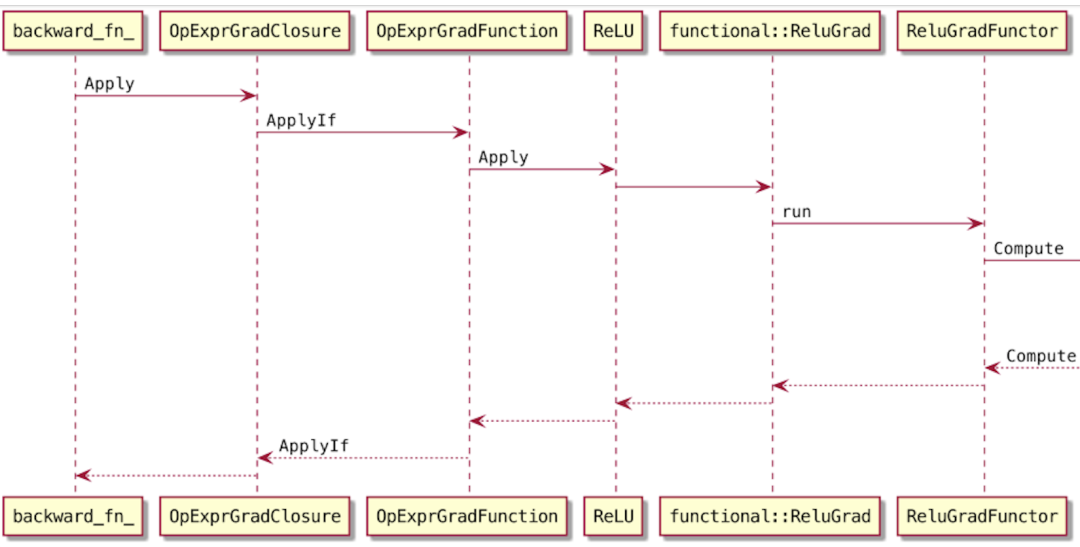
之前在5.1节已经确认,OpExprGradClosure::impl_就是ReLU(https://github.com/Oneflow-Inc/oneflow/blob/48e511e40e09551408c96722c09bd061ce320687/oneflow/core/autograd/gradient_funcs/activation.cpp#L200)。
如前所述,backward_fn的参数中,output_grads是上游传过来的梯度数据,backward_fn需要计算relu的梯度,二者的乘积赋值给in_grads。这些参数会一直传递到ReLU::Apply(https://github.com/Oneflow-Inc/oneflow/blob/48e511e40e09551408c96722c09bd061ce320687/oneflow/core/autograd/gradient_funcs/activation.cpp#L213)。
functional::ReluGrad(https://github.com/Oneflow-Inc/oneflow/blob/48e511e40e09551408c96722c09bd061ce320687/oneflow/core/autograd/gradient_funcs/activation.cpp#L219)的Functor名字是ReluGrad。对应的Functor是ReluGradFunctor(https://github.com/Oneflow-Inc/oneflow/blob/48e511e40e09551408c96722c09bd061ce320687/oneflow/core/functional/impl/activation_functor.cpp#L61)(命名空间是oneflow::one::functional::impl)。
ReluGradFunctor之后,是基于Primitive kernel实现的计算逻辑。ReluGradFunctor中对应op名字是"relu_grad",这个relu_grad的注册被包在一个宏定义(https://github.com/Oneflow-Inc/oneflow/blob/48e511e40e09551408c96722c09bd061ce320687/oneflow/user/kernels/activation_kernels.cpp#L331)中,实际上会返回一个BinaryPrimitiveKernel,这是一种稍显特殊的基于Primitive的kernel,其具体为ep::primitive
下的一种BroadcastElementwiseBinary工厂(https://github.com/Oneflow-Inc/oneflow/blob/48e511e40e09551408c96722c09bd061ce320687/oneflow/user/kernels/activation_kernels.cpp#L337-L339),其对应的cpu和cuda注册分别位于:
-
oneflow/core/ep/cpu/primitive/broadcast_elementwise_binary.cpp
-
oneflow/core/ep/cuda/primitive/broadcast_elementwise_binary.cu
最终实现位于binary_functor.h
(https://github.com/Oneflow-Inc/oneflow/blob/48e511e40e09551408c96722c09bd061ce320687/oneflow/core/ep/common/primitive/binary_functor.h#L354):
至此,完成了梯度计算的逻辑。
7.4 梯度的存储
FunctionNode::Apply执行完毕后,GraphTask::Apply调用FunctionNode::AccGrad4LeafTensor(https://github.com/Oneflow-Inc/oneflow/blob/48e511e40e09551408c96722c09bd061ce320687/oneflow/core/autograd/autograd_engine.cpp#L430)为叶子节点拷贝梯度数据。
在上述例子中,因为y不是叶子节点,处理到y.grad_fn_node_时不会进行实质处理。对于x,会调用CopyOrAccGrad(https://github.com/Oneflow-Inc/oneflow/blob/48e511e40e09551408c96722c09bd061ce320687/oneflow/core/autograd/autograd_engine.cpp#L84),这个函数逻辑的伪码形式如下
autograd_meta.acc_grad_ += autograd_meta.current_grad_
autograd_meta.acc_grad_就是Python端读到的x的梯度。
-
https://github.com/Oneflow-Inc/oneflow/tree/48e511e40e09551408c96722c09bd061ce320687
-
自动求梯度 (https://tangshusen.me/Dive-into-DL-PyTorch/#/chapter02_prerequisite/2.3_autograd?id=_233-梯度)
-
PyTorch 的 backward 为什么有一个 grad_variables 参数?(https://zhuanlan.zhihu.com/p/29923090)
-
PyTorch 101, Part 1: Understanding Graphs, Automatic Differentiation and Autograd (https://blog.paperspace.com/pytorch-101-understanding-graphs-and-automatic-differentiation/)
其他人都在看
欢迎Star、试用OneFlow最新版本:https://github.com/Oneflow-Inc/oneflow/https://github.com/Oneflow-Inc/oneflow/
Recommend
About Joyk
Aggregate valuable and interesting links.
Joyk means Joy of geeK