

What is vector search?
source link: https://www.algolia.com/blog/ai/what-is-vector-search/
Go to the source link to view the article. You can view the picture content, updated content and better typesetting reading experience. If the link is broken, please click the button below to view the snapshot at that time.
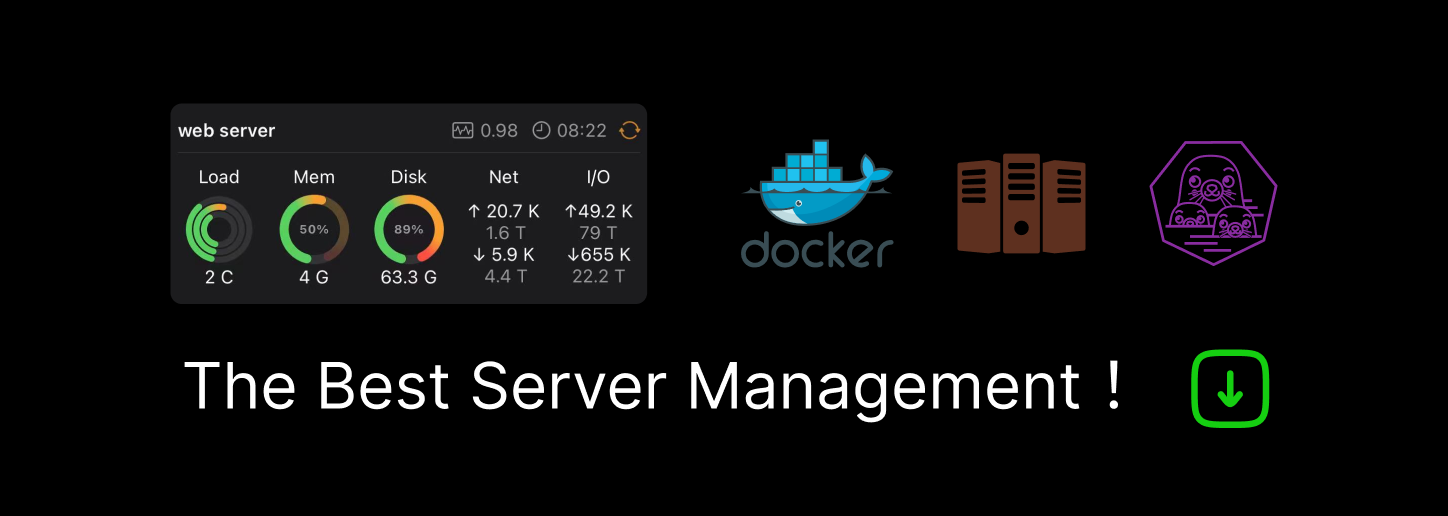
What is vector search?
Nov 17th 2022 ai
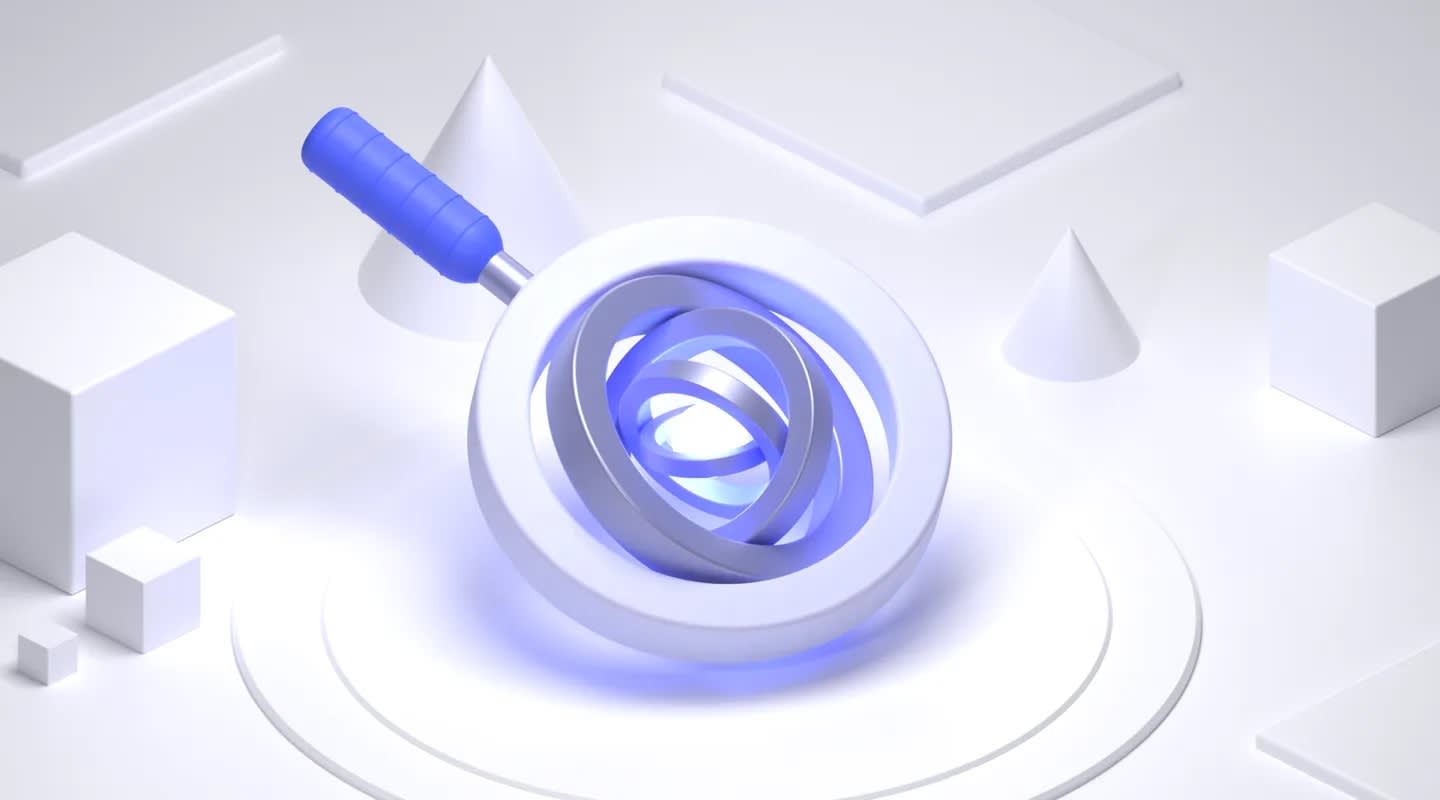
Vector search is a way to find related objects that have similar characteristics using machine learning models that detect semantic relationships between objects in an index.
Solutions for vector search and recommendation are becoming more and more common. If you want to add a natural language text search on your site, create image search, or build a powerful recommendation system, you’ll want to look into using vectors.
The research behind it has been decades in the making, but up until now building and scaling vector search has only been available to the largest of companies like Google, Amazon, and Netflix. These companies have hired thousands of engineers and data scientists, and some have even developed their own computer chips to offer faster machine learning.
Today, just about any company can deploy vector-powered search and recommendations in a fraction of the time and price. Vector technologies unleash a whole new era for developers to build solutions that enable better search, recommendation, and prediction solutions.
This blog offers an introduction to vector search and some of the technology behind it such as vector embeddings and neural networks. And, I’ll briefly describe neural hashing, a new technique that enables vectors to be delivered even faster and more efficiently.
The problem with language
Language is often ambiguous and fuzzy. Two words can mean the same thing (synonyms) or the same word can have multiple meanings (polysems). In English for example, “fantastic” and “awesome” can sometimes be synonymous, but “awesome” can also mean many different things — inspiring, daunting, divine, or even plentiful.
Vector embeddings (also known as word embeddings, or just vectors) along with different machine learning techniques such as spelling correction, language processing, category matching, and more can be used to structure and make sense of language.
What are vector embeddings?
Vectorization is the process of converting words into vectors (numbers) which allows their meaning to be encoded and processed mathematically. You can think of vectors as groups of numbers that represent something. In practice, vectors are used for automating synonyms, clustering documents, detecting specific meanings and intents in queries, and ranking results. Embeddings are very versatile and other objects — like entire documents, images, video, audio, and more — can be embedded too.
We can visualize vectors using a simple 3-dimensional diagram:

Image via Medium showing vector space dimensions. Similarity is often measured using Euclidean distance or cosine similarity.
You and I can understand the meaning and relationship of terms such as “king,” “queen,” “ruler,” “monarchy,” and “royalty.” With vectors, computers can make sense of these terms by clustering them together in n-dimensional space. In the 3-dimensional examples above, each term can be located with coordinates (x, y, z), and similarity can be calculated using distance and angles.
In practice, there can be billions of points and thousands of dimensions. Machine learning models can then be applied to understand that words which are close together in vector space — like “king” and “queen” — are related, and words that are even closer — “queen” and “ruler” — may be synonymous.
Vectors can also be added, subtracted, or multiplied to find meaning and build relationships. One of the most popular examples is king – man + woman = queen. Machines might use this kind of relationship to determine gender or understand gender relationship. Search engines could use this capability to determine the largest mountain ranges in an area, find “the best” vacation itinerary, or identify diet cola alternatives. Those are just three examples, but there are thousands more!
How vector embeddings are created
Some of the earliest models and attempts to represent words as vectors go back to the 1950s with roots in computational linguistics. In the 1960s, research on semantic differentials attempted to measure the semantics, or meaning, of words. Natural language processing (NLP), a way to analyze text to infer meaning and structure, began with complex sets of handwritten rules, but turned to new machine learning models in the 1980s. NLP is still used today in search engines to help structure queries.
It was in the late 1980s that a new statistical model, latent semantic analysis (LSA), also called latent semantic indexing (LSI), was developed for creating vectors and performing information retrieval. LSA is very good at understanding document relatedness by analyzing what terms are frequently used together to build a model of semantic relatedness (e.g., “royalty” and “queen”).
It is a good approach for handling certain kinds of problems — such as synonyms and polysems, and measuring distance (or similarity) between objects — however, it has difficulty scaling. LSA can be computationally expensive especially as the number of vectors increases or as the underlying data changes — for example, every time you update your catalog.
In 2013, Word2Vec was introduced as a new model to understand word similarity using neural networks. Like LSA, Word2Vec can be used to create the word embeddings and then be trained to find text that is semantically similar.

Image via IBM
As the name suggests, neural networks are machine learning networks that resemble the neurons in a brain. Underlying neural networks is a type of machine learning known as deep learning. Every “neuron” in a neural network is essentially just a mathematical function. The weighted total of each neuron’s inputs is calculated; the more significant an input’s weight, the more it influences the neuron’s output.
You can find deep learning used in voice assistants, facial recognition, self-driving cars, and many other applications. Deep learning can be trained on enormous datasets and is able to recognize a large number of complex patterns.
Examples of vector search results
Nowadays, there is a wide diversity of vector embedding models to process different data such as images, videos, and audio. There are also many freely available vector databases with vector embeddings and distance metrics that represent nearness or similarity between vectors.
There are also various algorithms which can be used to search a vector database to find similarity. These include:
- ANN (approximate nearest neighbor): an algorithm that uses distance algorithms to locate nearby vectors.
- kNN: an algorithm that uses proximity to make predictions about grouping.
- (SPTAG) Space partition tree and graph: a library for large scale approximate nearest neighbors.
- Faiss: Facebook’s similarity search algorithm.
- HNSW (hierarchical navigable small world): a multilayered graph approach for determining similarity.
There are tradeoffs between these different techniques and often you’ll see multiple techniques being used to deliver results faster and with greater accuracy. These various techniques will deliver better results even for hard-to-process queries. We will write a future blog about these different techniques and tradeoffs.

For example, when searching an electronics catalog, people sometimes type “usbc”, “usb-c”, or “usb c”. Do these mean the same thing, or is it for three different items? Keyword engines can struggle with this kind of formatting, and typically you might need to create if/then rules to teach the search engine how to manage this query. However, with vector search, this isn’t a problem. Vector search engines will know to deliver similar results.
Here’s a more interesting example:

In our test database with more than 20,000 products — which includes only product titles and brand names — we performed a search for “coffee gift card” (above). The term “coffee” is not in Starbucks gift card description, however, the vector engine can make the connection between “coffee” and “starbucks” to return good results!
Vector search challenges
Vector embeddings help us to find similarity between documents. When it comes to relevance, vector search is superior to keyword search for many types of queries. If they’re so great, why don’t we use vector search for everything? In fact, for many query types, keyword search still provides better relevance. Additionally, vector search is not very efficient and historically can’t scale without a significant investment in computer processing. With new, recently introduced neural hashing capabilities, vector search is finally able to scale. More on this below.
Accuracy vs keyword search
Vector search is terrific for fuzzy or broad searches, but keyword search still rules the roost for precise queries. As the name suggests, keyword search tries to match exact keywords. Other features such as autocomplete, instant search, and filters have also made keyword search popular.
For example, when you query for “Adidas” on a keyword engine, by default you will only see the Adidas brand. The default behavior in a vector engine is to return similar results — Nike, Puma, Adidas, etc.. They are all in the same conceptual space. Keyword search still provides better results for short queries with specific intention.
Speed and scale
Bottlenecks are more likely with vector search because queries must do complex vector calculations to predict relationships as opposed to just reading column based indexes. Machines divide CPU time between various inbound processes. In fact, much of the embedding requires GPU inference also, which includes the queries, so this is even more complicated in some ways.
To cope, search engines either need more compute power or must instead process the same queries faster. Vector search companies have been pushing the benefits of vector AI for years, but the cost and performance issues have impeded its progress and engendered concerns about its viability.
Some companies that offer vector search module add-ons will attempt to skirt the problem by only running the vector search if the keyword search result is poor. The message is that you can have one or the other — keywords or vectors, speed or quality — but not both running at the same time.
Some have suggested that caching is a good way around this problem. The argument goes that by caching results you can virtually eliminate costs and provide results instantly. In practice, search queries vary considerably and the cost benefit for caches is often questionable. The cache rate of search can be extremely low, especially for sites with massive longtail content (using our own customer data we have seen, on average, 50% of the traffic are longtail queries that are not frequent enough to be cached).
One fix to all of these problems — accuracy, speed, scalability, and cost — is called neural hashing. We’ll explain briefly how it works.
Binary vectors
Vectors work, but as mentioned above, have speed and scale limitations that affect performance and cost. We took a different approach, called neural hashing, that leverages vectors without tradeoffs.

Vector search engines use neural networks and deep learning models to deliver semantic search capabilities.
Neural hashing makes vector-based search as fast as keyword search and this is done without the need for GPUs or specialized hardware. Neural hashing uses neural networks to hash vectors — compressing the vectors into binary hashes (or binary vectors). You may have heard of hashes; cryptographic hashing is a commonly used technique in security for producing a tiny, unique output for protected password comparisons.
Performance-wise, these hashed vectors can be run on commodity hardware, retain 96% (or more!) of the vector information, and can be calculated hundreds of times faster than vectors alone.
Now, if there was only some way to get keyword search and neural hashing into the same query….
Hybrid search
Hybrid search is a new method to combine a full-text keyword search engine and a vector search engine into a single API to get the best of both worlds.
There is tremendous complexity in running both keyword and vector engines at the same time for the same query. Some companies have opted to go around the complexity by running these processes sequentially — they run a keyword search and then, if a certain relevance threshold isn’t met, run a vector search. There are many poor tradeoffs for this such as speed, accuracy, filtering, and heap sorting. These so-called dual systems suffer because the vector databases often don’t have the same (or any) filtering capabilities so they return massive amounts of information that’s unnecessary.
True hybrid search is different. By combining full-text keyword search and vector search into a single query, customers can get more accurate results fast. For Algolia, we’ve combined neural hashes with our world-class and blazingly fast keyword search technology into a single API call. It scales to meet the needs of any size dataset — even for indexes that have a lot of changes with frequent updates and deletions — without any additional overhead.
We will post more information on hybrid search soon. Hopefully this has provided you with a good overview of vector search and how it can radically improve your site’s search results!
Recommend
About Joyk
Aggregate valuable and interesting links.
Joyk means Joy of geeK