

Good Design in ML Applications With Konrad Piercey
source link: https://neptune.ai/blog/good-design-machine-learning
Go to the source link to view the article. You can view the picture content, updated content and better typesetting reading experience. If the link is broken, please click the button below to view the snapshot at that time.
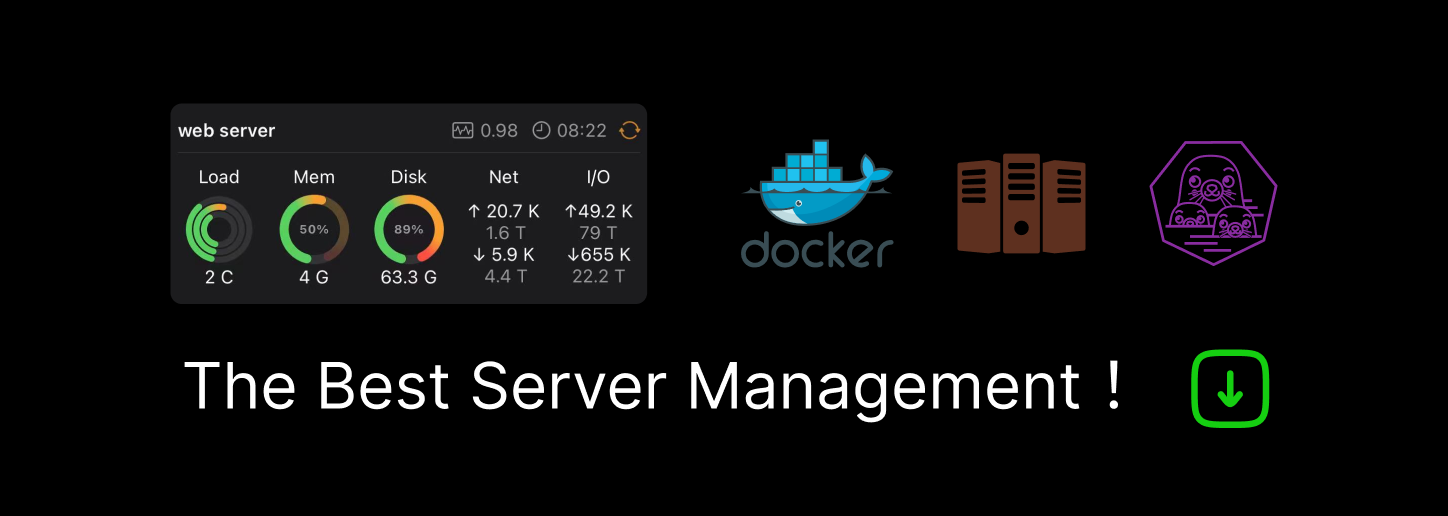
Good Design in ML Applications With Konrad Piercey
Some time ago, Konrad Piercey, a Lead Product Designer at Delivery Hero, was one of the speakers on the MLOps Community meetup in Berlin. He talked about good design in ML applications – why it’s a growing topic, what it actually means and how to start implementing it.
And we thought that it’s a very interesting topic that we definitely want to learn more about. So we sat down with Konrad and asked him a few(ish) questions. Here’s the effect of our conversation!
By the way, MLOps Meetups happen more and more often in different cities around the world. If you’re interested, you should check the MLOps Community schedule here. Maybe there’s a meeting happening in your city soon! You can also organize one – join the MLOps Community slack channel if you’re interested!
Okay, now, we can really talk about design in ML.
What is (good) design/UX for ML?
Patrycja: Let’s start from the basics. What is design/UX for ML? Is it anything specific for ML, or is it just the usual design? And why do you think it’s important to talk about it in the context of ML?
Konrad: The idea here is that a design for machine learning isn’t really an existing field. There are no machine learning designers, there are machine learning and data science engineers out there. Then there are product designers and UX/UI designers and then may contribute in some way to some elements of machine learning. But if they do, it is a very small part of the larger initiative of how machine learning is integrated into a product.
The main goal of the machine learning design is to help create a better experience and a better relationship between the machine and the user.
How do we do it? By revealing what’s behind the curtain and how the system is operating.
Because machine learning, from its early principles, is something that happens in the background. You don’t see what’s happening when it’s doing a number of calculations, building some models, and producing some output.
- You don’t see how that’s working.
- You also don’t see how you’re affecting the model generally.
And machine learning design is taking some fundamentals of UX/UI and applying that to the bigger product strategy behind how a company or product wants to integrate machine learning.
So you take UX/UI and machine learning and add those two together.
And I think if you were to ask a general person in tech what is machine learning design, they’re probably not going to mention many things that relate to the user interface, and that’s where I’m hoping to change the current industry outlook.
Why good design in ML apps is important?
Patrycja: And why do you think it’s important? Why do you think users have to have this good experience with ML apps instead of just getting the results of the model?
Konrad: Well, first, I think we should state the obvious, that machine learning is fascinating and it’s amazing. Some of the greatest machine learning integrations people don’t even realize. Forget the future where machine learning is going to be most of our digital products.
- Take driverless cars, for instance. Driverless cars won’t ever exist without machine learning.
- But even more basic machine learning at the consumer level is your auto-correct on your phone. That uses ML to ensure that it’s suggesting the proper correction or proper next word.
- My favorite example, though, is the email spam filter. Your email spam filter would never exist without machine learning. All those lovely emails saying you’ve won the lottery would go right to the top of your email inbox without machine learning.
So there are different levels of integration of these. Why it’s exciting and why we need to look at it now is because machine learning is starting to integrate into more personalized experiences that really define cooperation with a product or service. You can take most large consumer applications, and they use machine learning in some way.
Whether that’s Amazon, Facebook, YouTube, or Netflix, all of these use machine learning in order to either drive engagement or sales. And so, it’s important for users to understand how their interaction with the system is affecting their product experience.
Is “good design for ML” a new thing?
Patrycja: Do you see this need being noticed by the business? Is it something completely new, or do people already think, “okay, we need to design it in a way that people have this good experience”?
Konrad: I think it’s not totally new that data scientists and product designers try to be cautious with how they integrate and launch ML properties into their products or application. But it’s definitely not thought of at the depth at which it needs to be considered because the implications of machine learning now can affect things at an immense scale, like the critical infrastructure of electoral voting systems or moving the needle on nationwide obesity epidemics.
This is stuff that machine learning now affects. We’re not just talking about your email inbox spam filter anymore. We’re talking about things that fundamentally change societies or human nature. And this is only growing with time.
It’s also important to think about how quickly it moves forward. By the time it already has an impact, it can often be too late. So you can’t start to think of machine learning design after the fact because once you put a model in place, the impact it’s producing can already be widespread.
That can be both good and bad. The widespread success of a model then can have both positive and negative outcomes.
The biggest challenges when designing ML products
Patrycja: What do you think are the biggest challenges in designing ML applications in a way that they provide a good experience to people?
Konrad: The first step really starts with bringing in the team to understand how the model is working. What are the data points which you’re using to drive the model, and how are those being used to push the product forward?
And normally, speaking from my experience, data science and machine learning isn’t something that often has designers (people who are user-centered) coming in and speaking on behalf of the user, on behalf of their values. So I’d say that’s part of the growing problem, but also the growing opportunity.
Patrycja: Talking about your experience, could mention some specific challenges for the projects that you’re working on in Delivery Hero?
Konrad: Sure. So in Delivery Hero, we operate more than 12 different food delivery apps. And those 12 food delivery apps are present in over 70 countries. That’s a ton of people that we’re affecting.
In just one day, for example, more than half a million people order on our platform. So talking about scale and the impact of a product, by the time our product does its job, we’ve already affected so many users:
- what they’ve ingested and eaten,
- potential habit changes,
- how they think about and perceive food and their diet,
- And the rest of their day (if you ate a really heavy meal, you might not want to exercise later, right?)
So eating is really intricate part of our human nature, metabolic functions, and mood.
So at Delivery Hero, we’re looking to integrate machine learning more and more intensively because of the growing size of the organization and how many people we are affecting.
And at Delivery Hero, we’re looking at machine learning in a way where we can guide users to find better products, better food, or other items which they want to order. It’s similar to any other eCommerce platform. Obviously, we are trying to make a sale, but hopefully, not doing it with any negative intentions or built-in biases programmed into the system. That’s the main goal.
At Delivery Hero, we’re not trying to make people more unhealthy. We want people to be healthy, but we also want people to find things that they’re hungry for and to find something delicious… to feed the beast haha.
So, it’s now a challenge for Delivery Hero to find the best ways to move forward with machine learning that:
- Helps the business grow,
- Helps people find what they’re looking for regarding the products and foods,
- And also create a system that doesn’t undermine the morals and values that those users have when using our platform.
How to align good user experience with business goals?
Prince: This is really interesting. You’re seeing Delivery Hero aligning its goals with the user goals. But what is the business incentive there?
For example, the users won’t be buying as much if they’re having the right foods which don’t make them binge eat. That means perhaps fewer sales.
So how are you guys aligning those two things with your design?
Konrad: A lot of good questions. I think we can break that down into a few areas. I think there’s a section that you touched on – a user perception of the brand, loyalty brand perception.
And within these different fields of balancing is what I call: the biggest balance. That’s balancing shareholder profitability and big tech with what is morally the right thing to do.
Because in any large-scale business, you’re there to grow the business, to make the business profitable. And that is still ongoing. Even in Delivery Hero, I am a shareholder, and most of the staff is, and that’s what is hard.
There’s no easy answer to balancing shareholder profitability with the best product experience for users. That’s why it’s a conversation, an ongoing conversation that we have all the time.
But I think most businesses and organizations don’t fully realize the scale at which their technology is constantly moving and morphing (for us in Delivery Hero, it’s around our growing industry of logistics and food). Users are becoming more aware of their interaction with these large-scale businesses, whether it’s Facebook, Uber, ride-sharing, or food delivery. With the growing technology, users understand the product that they’re using (maybe a bit slower than the people making the product itself). But users understand:
- “Okay, these things are getting smarter”,
- “The experience, my feed, it has been uniquely crafted for me.”
The users, including you and me, we’re not dumb, but we don’t always know how the technology is working. Once users get an idea of how the service may be potentially misusing them, that can have a huge detractor and effect on brand loyalty and brand perception.
So if I realize:
- 1One service is really pushing sales no matter what the outcome,
- 2The other suggests items to buy, but it also says, “hey, you can set your budget to make sure you don’t overreach each month. We know not everybody’s just made of money, so let’s help you spend mindfully.”
I think this type of value to product design is something that’s growing quite fast because users are seeing what’s happening to their digital services and how they’re often being misused against them.
You can see right now the churn against Facebook, for instance. There are many competitors to it, so it’s not the main reason why Facebook is losing a large swath of younger audiences. But in large part, it’s because of brand perception and the product experience.
Facebook isn’t the newest kid on the block when it comes to apps that have a large footprint. But with that footprint, there comes a heavy lingering shadow behind the product:
- What it means,
- What it does to people,
- How it’s used.
And businesses get ahead of that by being a bit more honest and open with users, saying, “look, we’re here to make a profit, but we also want to provide the best value, the best experience for you”.
How are they doing that? How are they voicing this approach to product design through the app experience, through the UI, through the UX? That is being crafted now. That is a new field. How to engage the audience in an honest and open way while not undermining the core business values of:
- 1Growing the business,
- 2Growing sales,
- 3Growing engagement.
Prince: I would like to understand some of the metrics you guys are aiming at. Are you using any metrics, or did you see any difference between when you started and now?
Konrad: I can’t dig into those small details about the intricacies of how Delivery Hero is using machine learning today. A lot of it is still intellectual property, stuff that we’re building or testing, and we want to keep private for the time being.
What I can say is the general outlook. Some of the things we’re hoping to build and we’re testing today are suggested content based on how you’re using the system and your surrounding elements (the region, the city you’re in.)
All these factors are taken into account, some of them we can use more accurately, and some of them less. But these data points would be suggestions based on your previous purchases or your previous search history.
We take these in, we look at these, and then we suggest material based on those criteria. This is very similar and not unlike the other digital products you’re using today.
At its most basic principle, this is how Google search works. Based on how everybody else is searching for similar words, in your similar country, at a similar time of day. These are the data points that later produce your search results. And your search results are refined very heavily.
When you look at a product experience that’s consumer-driven (Netflix or YouTube). These features are heavily personalized just for you. We’re all gonna have different feeds and content. And that’s a good thing, that’s helpful. That’s exactly what machine learning is supposed to do.
Unfortunately, machine learning can also show you content that is overwhelming or content that is leading you down into an echo chamber. From the food side, we want to suggest the content you’re looking for. But if you constantly eat burgers on our platform, all we ever suggest to you is burgers. That’s not a healthy lifestyle, and we don’t want to do that.
So that’s where this balance comes in. We take the data points that can be helpful to craft an experience that is personalized to you, but also we balance and know the other aspects which contribute to overall physical health and well-being.

Patrycja: Maybe coming back to your specific project. In the context of understanding the ML or this demand to know what’s under the hood. Is there a difference in how people want this in different parts of the world?
Konrad: Well, I wouldn’t say that our integrations with ML are going to change drastically from one region to the next. I can only speak a little bit on behalf of my ML team, as I’m not directly a data scientist or machine learning engineer. I’m on the design side. I can talk about our cooperation and our goal vision setting. With the details of exactly which models we’re testing in each region, I can’t specify that.
But, I would say it is not the larger goal to have some sort of radically different model for each country. You can think about it from a user experience side, the eating habits of somebody in the city center of Singapore are going to be different eating habits of somebody in Stockholm, Sweden.
- 1The things to eat
- 2The time of day
- 3The amount to eat
- 4Who do they eat with,
- 5How often are they doing meals together.
These are the type of things that are more interesting from the design side. They probably do have some effect on the machine learning models (which we would use).
Examples of good and bad UX practices in ML apps
Patrycja: So you talked a bit about those bad patterns in the UX for ML apps, like suggesting too much unhealthy food or trying to keep you watching something forever.
I’m wondering what you think could be done to make it a more positive experience for people. What are the good UX practices for ML applications?
Konrad: Good examples are a little bit hard to come by because, honestly, we don’t have many great ones. But I can share some examples that your audience may have already seen and already engaged with, but they hadn’t realized this is machine learning design.
Some poor examples would be where you have engagement on a level that is unhealthy. If you look at content-driven services (whether that’s social media or news), you don’t often get a sense of your path of consumption.
But right now, on your phone, you can see and set individual app limits (“I only want to use this app for this long”). This is a new feature for smartphones. This is not something that has existed for a very long time. You have to ask yourself – why does this exist now? This exists because of a growing need for it to exist. This is something users want because of our unhealthy technological habits.
You can also see this happening on YouTube. YT has a timer you can set that alerts you, “You’ve been watching for an hour. Do you still want to engage with the service?”.
TikTok actually has something that you’re not able to turn on or off. For some users, TikTok tested videos directly in the feed. As you were transitioning between content, a personality came on and said, “Hey, you’ve been watching a lot of content. Why don’t you take a break and step outside to get some fresh air”. This is great, but it also goes against the principle of the business to push users to engage more. Very interesting to see how industries are pushing these concepts forward of healthier habits.
So at this point, you’re seeing what happens if the model has become so effective that it’s now detrimental to the user. The machine learning model is, in a way, almost too good.
This is not something that people often talk about, but that’s what’s happening. This is the concept of very progressive machine learning modeling. AI and ML are at the point when models almost become too efficient. Now people (not machines) have to put these sorts of break times, like “Hey, it’s time to take a break”, and “Hey, you might have been consuming too much”.

But as Prince said, it goes against the business model to purchase more, to spend more time. Yes, it does. But that is the judgment of moral values that must occur on the design, on the product side, and on the experience side.
The process of designing and building ML applications
Patrycja: I see. So now, I’m wondering what the process looks like on your side when the designer is this extra person on the team. What does the workflow look like? When do you start cooperating with the ML team and the others?
Konrad: That’s an interesting topic because on my teams at Delivery Hero, we have the menu where you’re actually browsing products of a specific vendor, and you want to add stuff to your cart.
We’re also making suggestions on that screen. And the idea here is that as the menu grows, we will make more and more types of suggestions. The menus presented will be more personalized. There will be more input from the model in order to reorganize what’s presented to you.
This is the inevitable path of actually any food delivery app. Even outside of Delivery Hero, the apps will start suggesting and personalizing more and more content. They’re already doing this. But this will become a larger part of the product experience.
What’s important is that on my team, we started asking questions:
- 1Where exactly is machine learning gonna come in and make an effect on users?
- 2How would they be affected by this, in both positive and negative ways?
You don’t just look at machine learning and say, okay, what can I do for users in order to help them find a product at the price point they are looking for. You have to ask, are there any downsides to just adding more products to the basket?
We have to ask those questions simultaneously as we integrate this service.
Integrating machine learning isn’t all positive impact. You do have to weigh the outcomes. On our side, I think what we’re trying to do, is to educate both inside the company and outside to other designers.
If you know machine learning is being used in your team, the first thing is to educate yourself on:
- How that model works,
- What data points it’s using,
- And then what it produces.
So, what is the input, and what is the output. That’s what machine learning is, input and output. So educate yourself and your product team on those criteria.
From there, you can better understand how the model can be integrated and what it can or can’t do. I’m not saying make all the designers machine learning experts or take a course in machine learning, but understand the basic principles of how your model works and is being applied.
From there, there are actually three steps. Three super important steps to Good Design in Machine Learning (as I like to call it) GDML:
- 1Educational
- 2Simple
With these three principles or pillars, that’s how we define and approach integration with machine learning. So, if we want to integrate machine learning on the menu, we look at how we can bring something educational to the user by telling them what the machine is suggesting or maybe why we’ve suggested that. Speaking a little bit more in detail on how we change their feed or that what we suggest to them is having an effect on their habits. Because, again, that’s a two-way relationship between the machine and the user.
My interaction with the system changes the system. Also, what the system is displaying to me, what it’s giving me, changes me. In return, depending on what I interact with and order, this will change the system. And so that’s a symbiotic relationship that machine learning starts to build between users and the product.
But again, educational, simple, and fun:
- Educate – try to show something to users they didn’t know before. Try to teach them something new and valuable which is not naturally understood. That’s educational.
- Keep it simple – if we want to educate the user on something relating to ML or the model in the background, we’re not gonna teach them the data attributes of how things are going in or how things are coming out. We’re not trying to write a book or have them read a book on these complex mathematics.
Show them a simple graphic. That’s generally the approach we wanna take. We know that the phrase: pictures speak 1000 words. UX copy is super important here. We don’t want to lecture the user, we want to keep the language simple.
- And the last one is fun – we needed to add this one because, at the end of the day, machine learning, AI, and deep learning can be really complicated topics. And I think for a lot of people, it may be naturally uninteresting if it’s not easy to digest.
So trying to find a way to create some visual language or graphic that uses fun colors or fun animations to be a bit more humanistic. We want to try to put a smile on some users’ faces (I think the world could generally use a few more smiles in it). So trying to create a fun and engaging experience is also part of that.
Prince: How do you make people want to learn?
Konrad: Again, back to our values. Those three values or principles can be generally summarized in two words.
- 1Transparency
- 2Disclosure
Transparency and disclosure are sorts of our leading lights whenever we approach ML integrations. I think the design community also is approaching ML/AI/DL in this manner. We want to be transparent and disclose when there is a machine making decisions on behalf of people.
Transparency and disclosure are actually often legally binding rules in countries’ laws. This is a huge facet of UX principles that many large organizations are not aware of, and it could potentially put them at legal risk. If we look at UNESCO (where 200+ countries are member states), they actually adopted the first-ever AI ethics guideline, which states, “people should be fully informed when a decision is informed by or is made on the basis of AI algorithms”.
So we’re not talking about machine learning and design in some abstract way. As in, “we can maybe cut some corners here and do some things there,” that may not be the best for users, but it’s going to help the business. Or even not thinking about the user at all – that’s a scary thought. Let’s just only think about increasing engagement and let that be the first priority, this type of strategy isn’t going to get you very far, in fact, it might be endangering your business.
Well, I think you’re gonna find that very soon within a lot of countries, and even businesses (sometimes they have their own internal guidelines for AI and ML) release newly updated rules for ethics and guidelines.
So, as I said, people should be fully informed when a decision is made on the basis of AI algorithms.
Most of the products we’ve already talked about today are doing this. They are suggesting content or showing you content, not because you necessarily want to see that content, but because it’s making an assumption you want to see that. And this assumption is being done on behalf of the machine.
And when you don’t like the content, you do have some options. There is this little hamburger menu, or three-dot menu, where you can choose “hey, I’m not interested in this” or “don’t show me this anymore”.
But generally speaking, those algorithms are hidden behind a deeper menu, a deeper layer, and it’s usually on a per-content basis. That means that I can decide whether or not I want to see this video or post, but it’s not giving me any analytical data as a whole. I don’t know how I’m consuming content there or what path I’m moving forward down.
In the food delivery space, it’s actually much easier. We have a much easier guiding light for us compared to social media, news, music, or movies. Well, the human body has very easy guidelines on what is and what isn’t good for us. Of course, this changes from person to person throughout the world.
But as an example, the WHO has a standard for public health. One of these numbers is for the average adult, they should consume at least 400 grams of fruit and vegetables per day (this is not an exact number for every single person. It depends on your weight, your height, your region, and other factors. . So local standards have to be taken into effect).
So whether it’s these AI and ML ethics guidelines, or clear health standards from the WHO, users need to be aware of how their product is affecting them and how the machine is making decisions on their behalf. As we’ve said, even suggesting on behalf of them – this also counts because they’re consuming, they’re engaging.
And as Prince said, that can be hard sometimes because you have to balance that with the growth of the business. You don’t want to put people off by potentially limiting their choices. That’s not what Delivery Hero wants to do. We don’t want to limit people’s choices. Nor would Netflix or Instagram want to stop people from watching a movie or from reading a post.
That’s not the idea to limit the content, to draw a hard line. It’s just to inform the user. And machine learning design, or GDML, is all about that. Not about saying what’s right or wrong but about guiding the user to more information so that they can be better informed. We only want to inform.
What’s next for a good design in ML?
Prince: You talked about limits in services that are based on machine learning. I am wondering, in terms of good design ML, or probably the next few steps, do you see imposing or adding limits or summary statistics to applications in ML becoming a standard?
Konrad: If the question is whether we want to give users the ability to turn off the algorithm – I don’t think that’s gonna happen any time soon. Nor do I think it’s the best thing to do. I think giving the user options to reset their algorithm, or to totally turn it off, may not be that beneficial.
For instance, if you take your YouTube account, I don’t know how helpful that would be for the users to just turn off their algorithms. That’s gonna be very strange. Of course, if they really want to do that, I could just make a new account and start from scratch. But there is no button on YouTube that says “reset my algorithm”. It’s always just constantly feeding into what you consume, whether that’s like your Google search history or what you’re actually watching on YouTube.
I’d say probably the growing trend is more looking at the broader awareness of what we’re going to give to users, e.g., the statistics you mentioned. Bringing a broader awareness of what the machine has learned about them and giving users some insight into it. Again, coming back to the first principle of GDML. Education, showing some of those statistics, is gonna be a large fundamental part of it.
One of my favorite examples is if you use Spotify, perhaps you’ve seen your end-of-year summary, they call it “Wrapped”. Have you guys seen that? That is GDML. However, it’s only a surface-level implementation of what we’ve been talking about so far for good design machine learning. Your end-of-year summary Wrapped on Spotify shows you how you have affected the system and how the system affected you. It shows you how many songs you consume and how many hours you listen to. They can even break that down into morning, afternoon, and evening. They’re showing you how your data is unique in the system. Also, based on its suggestions to you, it shows you, “hey, you’ve discovered x number of artists, and you’re in the x percentile of this genre for people who listen to similar music as you. ?”. That is the start of GDML.
However, I want to make an important note that even though this is a nice example of modern GDML, Spotify summary wrap doesn’t actually show any deeper level value to the user. It’s showing very surface-level numbers, stuff that wouldn’t necessarily help the user in any way. It’s just somewhat interesting. A key factor to good design in machine learning is to educate the user on something actually useful, not just frivolous data.
The mock visual examples I’ve shared with you (Food Delivery app, Facebook, Amazon) show how we can bring a deeper level of meaning using GDML by showing statistics and relevant data to the user. In these draft examples, users go away learning something that has some deeper level of meaning or value to their life, habits, consumption, or purchases.


Spotify is a nice example because it shows what it means to peek behind the curtain of the algorithm. These statistics of how much you consume that song in the year versus everybody else etc., you would never know that by default. They show you these because it’s interesting, it’s about you personally. And that’s why people like it. It’s about me. It shows why I’m special. But it doesn’t go beyond that really surface-level projection of information.
How to measure if design improvements affect users?
Patrycja: After implementing some good design practices in ML, do you somehow test or check if users start to use the application differently or they limit the usage? Or the opposite, maybe they use it even more now that they are informed. Do you have this data?
Konrad: Inside Delivery Hero and our family of food apps, we’re not gonna know the real results for some time, but we do know:
- what users are asking for,
- the solutions that we’re building,
- and the process that I’ve shown to you today (educate, keep it simple, try to make it fun).
As well as those principles of trying to be transparent and disclose how the model is working. These are our best efforts to provide what’s right for the customer.
But on the part of impact and validation, how do we know what we’ve built is meaningful? Well, there are some ways to find out, and there are some processes and standards which are helpful.
You need to contrast soft qualitative metrics against hard quantitative metrics.
What we’re talking about here is user interviews vs. live data analytics. So things like whether people use the product more after they’ve viewed some bar graph showing usage of the system or whether they’re eating more noodles and pizza at night versus in the afternoon.
So checking, after a user has engaged/seen content related to their machine learning algorithm, how they’re going to continue engaging with the product. You can tell some of that by data analytics. But you also have to contrast that against user interviews because some of that won’t be able to be told by just the base numbers. You need to do interviews and studies. So spending some time on research and user research is never a waste of time.
- 1Eye tracking,
- 2Dwell time,
- 3Click-through rates,
All this stuff is super helpful. So all that can help you validate the impact of your GDML.
I will make a specific statement about using click-through rates. Let’s say you have some GDML component that helps educate the user on the algorithm and the product experience: how it’s changing, how they’re changing it, and how it’s changing them. If you do have some specific component that people can click on and learn more about their data, you should have that CTA very non-intrusive. So it really shouldn’t be visually screaming at the user, but it should be there if they want to click on it and learn more. But it shouldn’t be yelling at them like,” you need to take a look at this now! You should investigate your data, that would just sound scary.
Again. We’re not trying to scare the user or make this a critical part of the user flow or user experience. But we’re giving them, what’s most important, the opportunity to learn more if they’d like to learn more. We’re not hiding this stuff. We don’t wanna make it to seem like we’re hiding anything that is potentially affecting their mental or physical health.
Also, it’s worth mentioning that, in many cases, companies don’t hide that information intentionally. They often don’t even necessarily know what the system is doing or what effect it has on the user.
Let’s take Facebook in the U. S. – the fake news propagation. They didn’t know fake news was a problem till it already was a problem and wrecked havoc for a critical US election. So that shows that once machine learning at scale is applied, the effects are much faster than what you might imagine because that’s the nature of machine learning and product consumption on a user’s part.
If it’s propagating information or propagating sales or making suggestions, we should know:
- What is that doing to the user?
- How can we inform them before we know, as a product, what is good or bad for them?
That’s why GDML is so powerful, it keeps the user informed so that they can make a decision on what they think is healthy for them.
Patrycja: It is also touching what you said before that before educating users about the product, people also have to educate themselves. They need to understand the tool and what the algorithm is doing before they even can share this info with others.
Konrad: Yeah, it’s something new that designers and product people will have to start implementing into their own internal processes. Just five years ago, I didn’t have to worry about ML or deep learning or any of this algorithmic learning. It wasn’t part of my process or methodology as a designer.
But now, when machine learning is integrated into almost any digital consumer experience, designers really have to take a step forward and start participating in those conversations. Both learn about ML, in general (how it works), but also specifically inside their teams (how they’re planning to use it, how they want to apply it). That will be part of the growing portfolio of needs from new people in the product and design space.
How can Data Scientists and ML Engineers help UX designers?
Patrycja: Following up on what you said, let’s go to the other side of this process. Is there anything machine learning people, like data scientists and ML engineers, can do to make it easier for you to design this product in a better way?
Konrad: I would say, if you’re a machine learning engineer or data scientist, strike up a random conversation with one of your fellow product or design people. Make new friends there.
The interaction between machine and user, between data science and UX, is becoming so intricate now that it’s hard for us to understand how best to move forward. These things are just being defined now. Designing machine learning is not an industry that currently exists. It is a burgeoning field.
So, anybody who’s super interested in it, if you are going to get ahead on that curve, you have to know how to speak about the information in a way that’s personal, that is humanistic, and you can only speak about it if you know what you’re talking about. This is not a call out for every designer to become a machine learning engineer or every machine learning engineer to take a boot camp on UX.
It’s about sort of linking arms and moving forward together to create product experiences that we’re proud of. To create products for the people who come after us, for our kids, for your grandparents, and for the people who are long after once, we’re dead.
We have to set an industry standard for what is right here because we’re no longer creating static product experiences, these products are, in a way, sort of growing organisms themselves.
The product experiences we now craft are moving, flexing, and vicious in their nature. And the machines, our newest friends, are part of that. So we have to learn how to integrate with that properly.
Most important design principles
Prince: Yes, that makes sense! Before we finish, I wanted to ask you, what are your top three things, principles, or must-haves, when designing UX/UI design before releasing any machine learning application or your first iteration of the product?
Konrad: Wow, I would say:
Get to know your audience and become your audience. As you’re only able to build a great experience if you know and can connect empathetically with your users and with your customers. So that’s the most important.
And that’s what being a product designer is.
- 1Try to break the product,
- 2Talk to the people you’re affecting,
- 3And if possible, use what you’re building. That’s a super important part.
I think even big businesses today don’t do that enough. And it’s sometimes difficult to ensure that everybody working on the product really engages with that product, uses it, and tries to be a part of the experience instead of just designing or building something which you don’t really have the perspective on what is in the end experience.
Good Desing Machine Learning (GDML) community
Patrycja: Okay, the final question is about the GDML community or the movement…
Konrad: The movement… hah, sounds like I’m starting an army here, “If you want to join the ranks of GDML, I’m gonna post a signup sheet”.
Patrycja: Not yet, but who knows?
Konrad: So GDML, it’s the phrase we found that best summarizes our attitude toward creating a better user experience where ML, deep learning, and AI are involved, and that stands for Good Design Machine Learning. Learn more about GDML
We want to be good. We want to do good. We want to design good. And with GDML, we’re just trying to find other people who are interested in this topic. That’s the main thing. People who are interested to learn more, who are interested in progressing in this field.
Currently, I’m acting as a pseudomoderator in this group and in my own specific areas, but we’re eagerly looking for engineers, data scientists, designers, and anybody else who wants to help communicate GDML in their own company or maybe start a meet up in their own community.
As a side gig, I’m also working on completing my first book, on GDML, as you might have assumed. We will hopefully see that coming out sometime soon, but I won’t remark on it too much until it’s on the shelves.
Patrycja: Okay, perfect. Thank you for sharing your experience!

Konrad Piercey
Konrad leads founders and their teams to understand successful product & experience design methodologies, merging business development with user experience and product design.
Konrad is a product steward and IOT guru, interested in the way consumers connect with changing digital landscapes. With 10+ years in UX/UI design and launches in the US, EU, and Asia, he loves building unusual products. Mentoring teams to best understand their potential and be industry leaders. Konrad guides organizations on how to achieve their goals and create impactful products.
- Follow me on
Recommend
-
64
We have S.O.L.I.D principles of object-oriented programming, and we also have Software design patterns...
-
20
T raditionally, databases are used t...
-
6
Is Rails a good fit for writing rich frontend applications? This is a question I’ve asked myself months ago, working as a consultant for a client with a quite unique app. Does it make sense to stick with Rails? Maybe it’s...
-
10
Blocks Courtesy of Konrad ZuseSeptember 28, 2014Apparently, I've got a theme going of Weird Syntax. Let's run with it. Konrad Zuse was an early pioneer in computer science, although his name is perhaps somewhat less well...
-
4
The Other First Computer: Konrad Zuse And The Z3 Bavarian Alps, Dec. 1945: Since 1935, Berlin engineer Konrad Zuse has spent his entire career developing a series of automati...
-
3
Upcoming Talk on How to Achieve Flow With the Right Batch Size? by Konrad Swistelnicki of mbition We will be hosting an industry talk on “How to achieve flow with the right batch size?” The talk is free and open to the publi...
-
7
Zero-Fuss Docker Database Backup A simple tool to create backup of all databases on a host. Supports postgres and mysql/mariadb. Successor to this script.
-
5
The No Title® Tech BlogTales of an exploration on antigravity and other potentially unrelated matters There are new applicat...
-
5
Apply Now Android Developer at Konrad Toronto ...
-
6
[赛贝24-748] Konrad律所代理金属钻头附件起诉,涉及专利侵权!未提出TRO![24-cv-748]赛贝知识产权平台赛贝知识产权,云端知识产权大平台
About Joyk
Aggregate valuable and interesting links.
Joyk means Joy of geeK