

Handling personal identifiable information in your data
source link: https://xebia.com/blog/handling-personal-identifiable-information-in-your-data/
Go to the source link to view the article. You can view the picture content, updated content and better typesetting reading experience. If the link is broken, please click the button below to view the snapshot at that time.
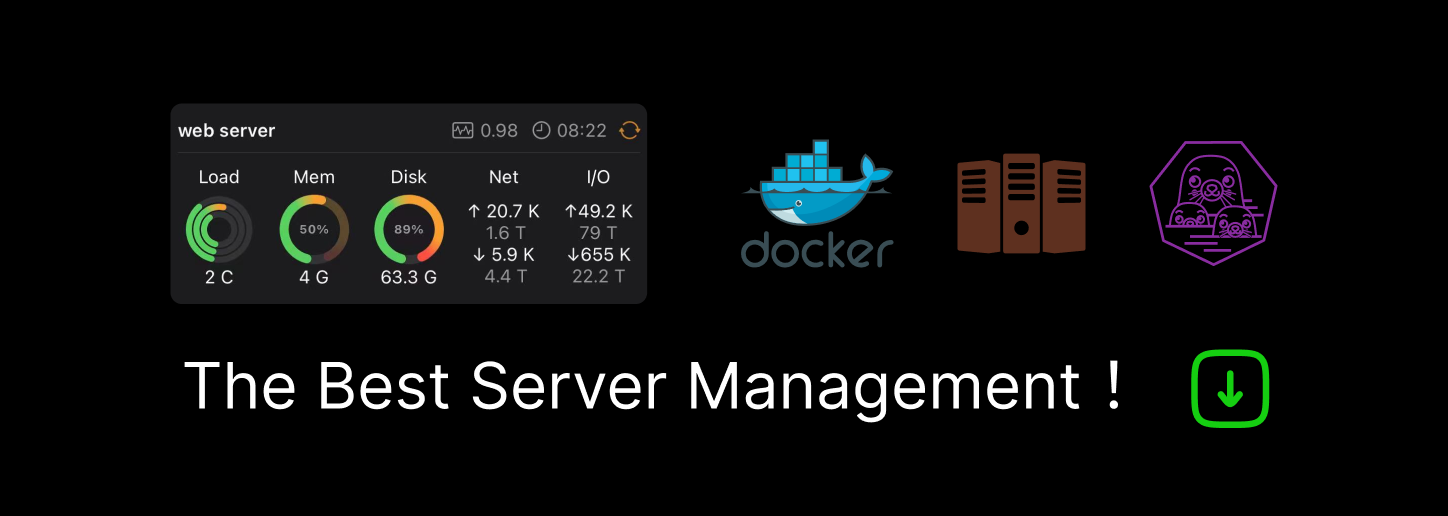
In one of my previous blog posts we discussed a number of data and security-related topics. One of the topics that were discussed was about handling personal identifiable information (PII) data and complying with regulations like GDPR. In this blog post, I want to dive a bit deeper into that topic and give a few examples of services that can be used for handling Personal Identifiable Information in your data with AWS.
Handling Personal Identifiable Information in your data pipelines
Data is often considered to be the new oil or gold, depending on whether you prefer sticky or shiny things. Because of that, applications tend to collect as much data as they can in order to get the maximum value of it. Collecting and storing all that data will likely result in storing some personal identifiable information.
The definition of what is considered PII data differs between regulators, e.g. specific data points like a credit card number or name versus collecting enough contextual data to be able to point to a specific person. In this blog post, the focus will be on how to deal with specific data points, although it can be a basis for dealing with contextual data as well.
However, it might not always be evident if your data contains specific information about people. You might for instance allow users to upload documents. Those documents could possibly contain sensitive data without you actually knowing it. Luckily AWS has a few services that can help with detecting these types of data.
Amazon Comprehend
Amazon Comprehend is a service that uses natural language processing to analyze texts and return all kinds of insights about them like sentiment, topics, and entities. However, Amazon Comprehend also detects PII in your text. As it currently stands Comprehend only supports PII-detection in English. If you are using any of the other supported languages by Comprehend you could try and train your own custom classifier to sidestep the missing functionality.
Amazon Macie
Amazon Macie is described by AWS as a fully managed data security and data privacy service. It has a couple of features that help you improve your security and privacy. One of them is detecting sensitive and PII data in your objects stored in S3 buckets on AWS. It can scan documents like pdf and docx to detect any number of data types that can be considered sensitive or personal. The number of data types that it can detect is quite extensive but might not cover all your use cases. One of the nice things about Amazon Macie is that you can register custom data identifiers. Adding an identifier for a dutch personal identification number could be done by specifying the regular expression and optionally some keywords to improve accuracy.
AWS Glue
The third option that I want to share with you is using AWS Glue to detect PII in your data pipelines. Although AWS Glue is probably used mostly for its ETL capabilities, it can also do some neat little tricks. One of them is detecting PII in the data that goes through your AWS data pipelines. Just as with Comprehend and Macie it mostly focuses on US data types like US passport numbers or social security numbers along with some generic types like names, email addresses, and IP addresses. However, as with Macie you can extend the detection capabilities of AWS Glue by creating your own custom identifier.
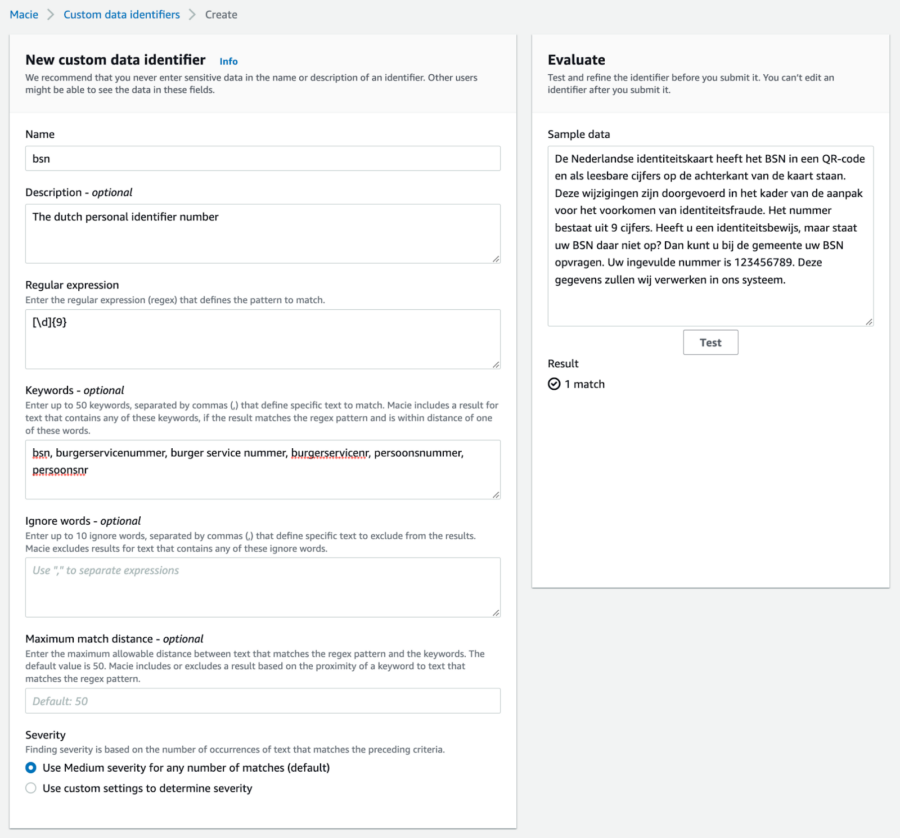
Now that we know where we actually store the personal information we can act on it. Depending on your use case you might start with redacting those parts of the data or by simply deleting it. In the next section, I want to walk you through the steps of redacting PII in your AWS Glue data pipelines.
Removing PII from your data with AWS Glue
To show you how you can detect and optionally remove the data in your Glue data pipeline I will take you through a few steps on how you can add a detect PII transformation and how you can add your custom detection patterns.
We start by creating a new visual job with both the source and target specified as S3. I already have a bucket where I can place the input and output of the pipeline. In the input location, I uploaded a CSV file with some mock data to test the pipeline. In the data source settings, I specify the bucket and prefix and Glue automatically tries to detect the files in the bucket.
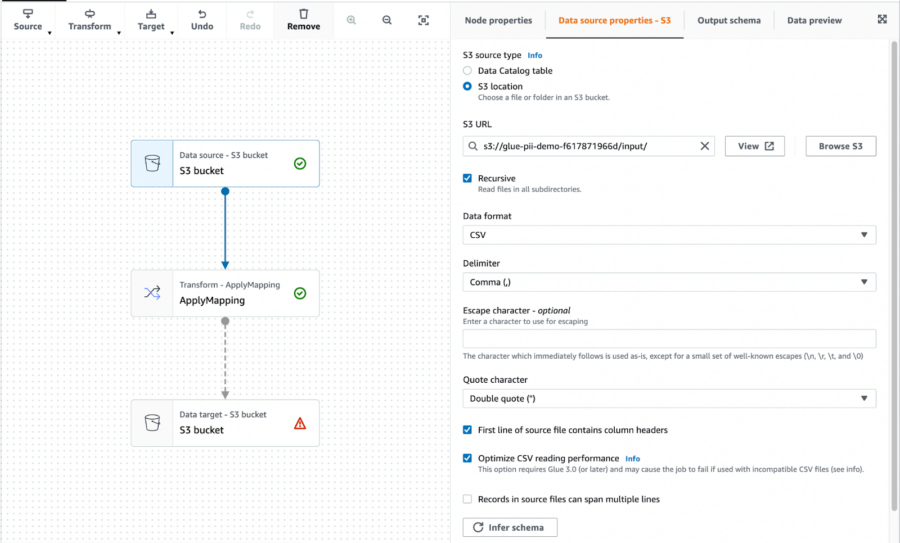
You can let Glue infer the schema automatically by clicking “Infer schema” and checking the result in the “Output schema” tab. In the data target node, I configured the same S3 bucket but with a different prefix. In my case “output/”.
The next step is to add a new transform step that will detect the PII. Select the ApplyMapping node and add the Detect PII from the Transform menu. After setting the new transform node as the parent for the Data target it should look like this:
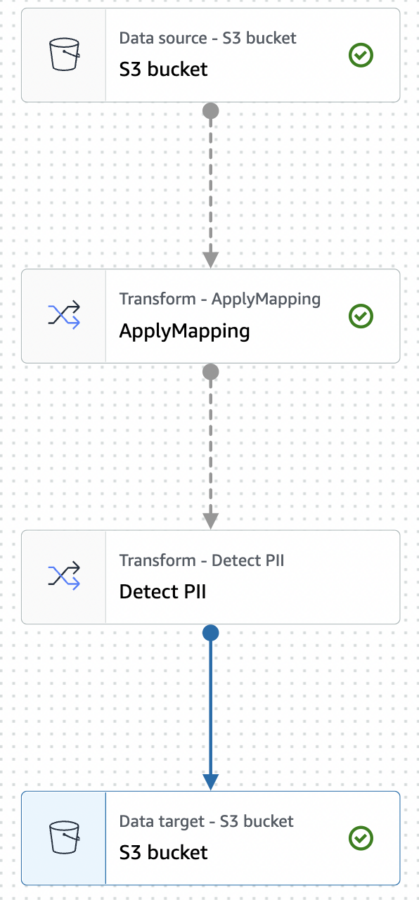
Now we need to define what types of PII to detect and what to do with them. We do this by selecting the Detect PII transform and selecting the option “Select specific patterns”. From the list, we pick Person’s name, Email Address, and IP Address. However, I also want to detect some specific fields like the Dutch citizen service number (BSN) and any bank account numbers (IBAN). To allow Glue to detect them we can create custom detection patterns, you can do this by creating a new detection pattern and filling in the details.
For demonstration purposes I created to simple patterns for the BSN and IBAN numbers like this:
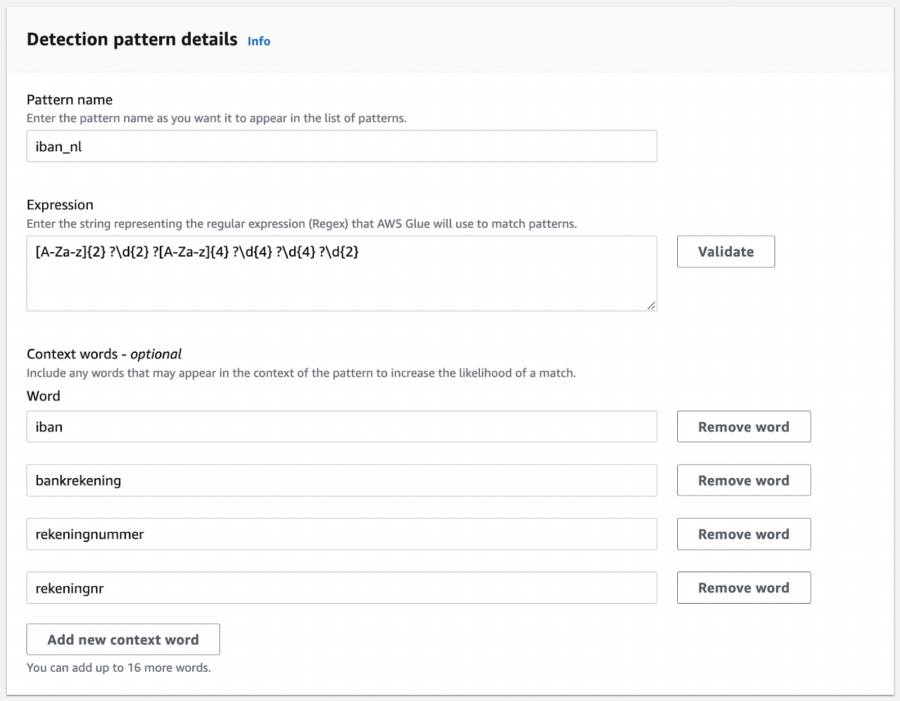
After having created your custom patterns you should be able to select them from the list in the transform step. The last step is to define what to do with the detection results. Here you have two options. Either enrich the data with the detection results or redact the input by masking or deleting it. In case you want to apply different actions for different types of PII you can simply add a separate Transform step with a different action.
Testing the pipeline
To test the data pipeline I generated some fake data with names, email addresses, a subject line, and a body with paragraphs from Lorem Ipsum. In the body, I inserted some generated fake bank account numbers and fake personal identification numbers. For the input, I generated a CSV file with 1000 lines. 197 lines contained a bank account number, 39 contained a personal identification number and 6 contained both.
After running the job Glue added an extra column with detected entities like this:
{ "DetectedEntities": { "first_name": [{ "entityType": "PERSON_NAME", "start": 0, "end": 8 }], "body": [{ "entityType": "iban_nl", "start": 124, "end": 146 }], "email": [{ "entityType": "EMAIL", "start": 0, "end": 22 }], "last_name": [{ "entityType": "PERSON_NAME", "start": 0, "end": 6 }], "ip_address": [{ "entityType": "IP_ADDRESS", "start": 0, "end": 15 }] } }
As you can see it specifies which entities are found in which fields and their position inside the field. In this small test setup, it was able to detect all the bank account numbers and personal numbers. In reality you will probably come across cases where spelling is different or include no context words at all, which will make detecting those entities harder.
Given the detection results above you can decide on your next steps like masking, tagging or anonymizing the data in another way. In the case of masking, you can do that directly in the PII transformation step above instead for simplicity.
Wrapping up
Handling Personal Identifiable Information in your data can be challenging, especially if you are not sure what data you are processing in the first place. By utilizing AWS capabilities in your data pipelines and AWS environment you can get a better understanding of the types of data that you store and it can help you process them correctly.
If you want to know more about the services mentioned in this blog post you can follow the links below. If you want to discuss how this can be integrated into your own data pipelines then don’t hesitate to contact us.
Discover and protect your sensitive data at scale with Amazon Macie
Detecting PII entities with Amazon Comprehend
Detect and Process sensitive data with AWS Glue
Recommend
About Joyk
Aggregate valuable and interesting links.
Joyk means Joy of geeK