

AIOps: What, Why, and How?
source link: https://dzone.com/articles/aiops-what-why-and-how
Go to the source link to view the article. You can view the picture content, updated content and better typesetting reading experience. If the link is broken, please click the button below to view the snapshot at that time.
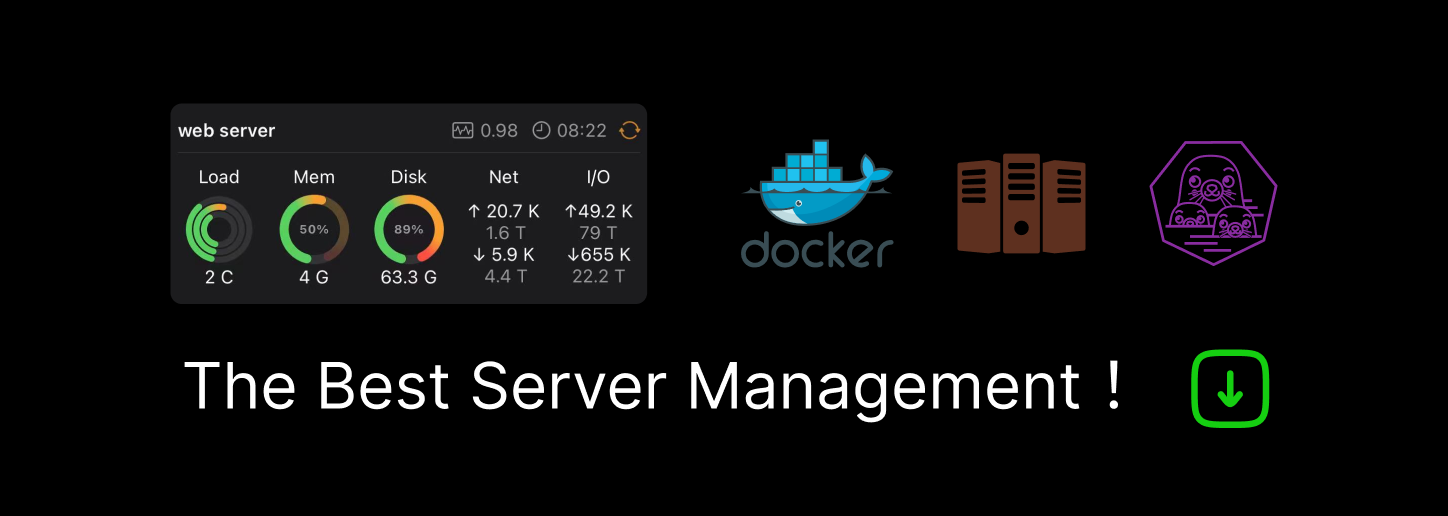
AIOps: What, Why, and How?
A Guide To Everything About AIOps: Use cases, benefits, challenges, core elements, AIOps architecture, and future.
Since Gartner coined the term AIOps in 2016, artificial intelligence has become a buzzword in the advanced technological world. The goal of AIOps is to automate complex IT systems resolution while simplifying their operations.
Simply put, AIOps is the transformational approach that uses machine learning and AI technologies to run operations such as event correlation, monitoring, service management, observability, and automation.
With AIOps, you can collect and aggregate ever-increasing data generated from observability and monitoring systems, different applications, or infrastructure, filter the noise to identify events and patterns for system performance and availability issues, and determine root causes and often resolve them automatically or send the alert to the IT team.
If you aren’t using AIOps to complete the process, then it will become difficult to run alongside technology innovation taking place at a rapid pace. Besides, if you depend on traditional knowledge and old systems, your IT operations are more likely to become unpredictable and unscalable.
As predicted by Gartner, 40% of the DevOps team is likely to implement AIOps in their applications and infrastructure monitoring tools for better platform performance and capabilities by 2023.
AIOps Architecture
The AIOps architecture provides methods and technologies that help in seamless integration for enterprise monitoring, service management, and automation to provide a complete AIOps solution.
AIOps Architecture Enabling Insights Across Operation Monitoring.
As shown in the image above, AIOps has three key areas when it comes to IT operations, namely Monitor (Observe), Engage, and Act.
Unlike traditional event management and monitoring tools, in observability, machine-learning-based functions are used to ensure there aren’t gaps or blindspots left while serving the organizations’ monitoring needs regardless of their architecture.
In the observability stage, primary processes that take place include data ingestion, data integration, event suppression, event deduplication, rule-based correlation, machine learning correlation (including anomaly detection, event correlation, root cause analysis, and predictive analytics), visualization, collaboration, and feedback.
The Engage section of AIOps architecture is related to IT Service Management (ITSM) and its functions that deal with processes and their execution through different metrics and functions.
As the Engage part deals with the data of service management, it acts as a repository for all the activities or actions occurring in ITSM, including problem management, configuration management, incident management, change management, capacity management, availability, and service-level agreements.
While in Observability events, metrics, traces, and logs act as the primary data; in Engage, the primary data remains around the execution of actions in different processes where the data is a blend of on-demand and real-time analytics.
The major phases in Engage consist of Incident Creation, Task Assignment, Task Analytics, Agent Analytics, Change Analytics, Process Analytics, Visualization, Collaboration, and Feedback.
Finally, in the Act stage, the actual technical task execution takes place. The act is the final phase that executes all the technical tasks such as change execution, incident resolution, service request fulfillment, etc. It is here that all the incidents discovered are resolved, and the system gets back to its normal condition.
How AIOps Works?
You can simply understand the working of AIOps by looking at the technology components supporting its processes — machine learning, big data, and automation. AIOps work best when deployed independently and provide a centralized system to collaborate for collecting and analyzing data from multiple monitoring sources.
Note: The data can consist of streaming real-time events, network data, historical performance events, system logs, and metrics, incident-related or ticketing.
After collecting the data, AIOps implement machine learning and analytics capabilities to:
- Identifying and separating significant abnormal event alerts from tons of data.
- Detects the root cause of the abnormal events and proposes solutions.
- Automates alerts to the operation analysts along with the proposed solution.
- Create remedies for abnormal events based on the nature of the problem and address problems in real time.
Finally, based on the analytics results, AIOps’ machine learning helps adapt algorithms and even creates new ones to determine problems at earlier stages and propose highly impactful solutions. Simply put, the AIOps model continues to improve, given the previous results.
Core Elements of AIOps
By now, you must know that the core elements behind AIOps are Big Data and Machine Learning.
To understand these two terms, we will take a better look at each of them here.
1. Big Data
As AIOps ingests data from numerous resources, it is essential to build the AIOps platform on Big Data technology. Big data refers to complex and large data sets that cannot be dealt with using traditional software for data processing. The data it contains comes in greater variety, increasing volumes, and high velocity, also known as the three V’s of Big Data.
As AIOps integrate large, complex, variant data sets from different sources into a data warehouse, the velocity of processing so much data volume can become unmanageable in case one doesn’t use Big Data platforms.
2. Machine Learning
The second yet most important part of AIOps is machine learning, a pivotal aspect of artificial intelligence. Machine Learning is centered on studying human behavior to replicate them using algorithms and data. When ML is implemented after gaining the information to solve a task, it can provide better accuracy in results than humans themselves.
Similarly, ML helps AIOps platforms to leverage their power to analyze data and detect patterns and anomalies while monitoring events and entities. The analyzed data is then used to offer insights and reach the root-cause alerts.
Benefits and Challenges of AIOps
The Major Benefits of AIOps Are as Follows:
- Higher System Availability: As AIOps ensures maximum application availability for the modern hybrid infrastructure, It has become a potential game changer.
- Better SLA compliance in the meantime to repair: Integrated with IT Service Management functionalities, AIOps can find patterns in events, identify useful insights, and allow automation solutions. All of that reduces the mean time to repair while exceeding the SLA compliance.
- Minimum Human Errors: As AIOps automates most of the mundane and iterative tasks of the operations handled by IT teams, it reduces human errors simultaneously.
- Better Automated Incident Detection: A lot of time is saved by AIOps as it reduces the noise created due to pseudo incidents by leading through event analysis to verify the incident.
- Prediction and Outrage Prevention: AIOps use essential KPIs to measure the performance of operations, creating intelligent suggestions to help IT operations complete their goal.
- Cost Optimization: A matured AIOps system can impactfully bring down the costs of operations by offloading tasks from humans to algorithms, leading human resources to spend their time on other important tasks.
- Better Environment Visibility: Using AIOps, businesses can identify opportunities, make strategic decisions, and identify inefficiencies in IT operations.
Some of the Challenges That AIOps Entail Are:
- Difficult Organizational Change Management.
- Mismatched Expectations.
- Rigid Processes.
- Difficulty in Data Availability and Monitoring.
- Lack of Domain Inputs.
- Inaccurate Predictive Analysis.
- Minimum Accuracy on Historical Data due to Data Drift.
- Difficulty in Understanding Machine Learning.
Use Cases of AIOps
As we know, AIOps is designed to gather and analyze IT operational data. Some of the popular use cases of AIOps are:
- Anomaly Detection
AIOps continuously analyze and compare data to its historical events that help in detecting potential problems.
- Incident Event Correlation
You can use AIOps for incident event correlation as it quickly processes and analyzes incident data while giving solutions to the problem before it gets out of control.
- Predictive Analytics
Apart from early error detection, AIOps with data gathering and analyzing features can help machine learning algorithms understand current and historical data trends while offering actionable insights into future outcomes.
- Digital Transformation
As AIOps removes the complexity of new technologies from ITOps, a new space for unrestricted transformation is created. It helps organizations to leverage flexibility to new advancements to deal with their strategic goals.
- Root Cause Analysis
One can also use AIOps in analyzing root causes by correlating numerous data points, tracking patterns of events, and more. The root cause analysis of AIOps helps businesses as well as their users in identifying and resolving issues more effectively, making the customer experience better.
- Cloud Adoption/Migration
With AIOps comes a clear understanding of cloud adoption and migrations’ transforming interdependence, minimizing the risks related to such shifting.
Future of AIOps
Given the advancements in technologies, most organizations are moving from traditional infrastructure to a dynamic one running on virtualized environments that can be reconfigured and scaled as required.
But, as we know, these systems tend to generate an enormous volume of data endlessly. Even Gartner has suggested that IT infrastructures are more likely to create two to three times more operational data every year.
Needless to say, traditional solutions can’t keep up with such data volume, sort events from the surrounding environment, or correlate data to provide real-time analysis and insights on IT operations to meet customer needs.
However, with AIOps providing visibility into dependencies and performance throughout the infrastructure while analyzing data, extracting abnormal events, or automating alerts to the IT team, it becomes the best solution for modern organizations.
Undoubtedly, AIOps are platforms utilizing modern machine learning and big data along with other advanced analytics technologies to improve IT operations with dynamic, proactive, and personalized insights by finding the root cause of problems and providing recommended solutions.
Recommend
About Joyk
Aggregate valuable and interesting links.
Joyk means Joy of geeK