

布隆过滤器是否好用,得看哈希函数写成啥样 - 小傅哥
source link: https://www.cnblogs.com/xiaofuge/p/16783301.html
Go to the source link to view the article. You can view the picture content, updated content and better typesetting reading experience. If the link is broken, please click the button below to view the snapshot at that time.
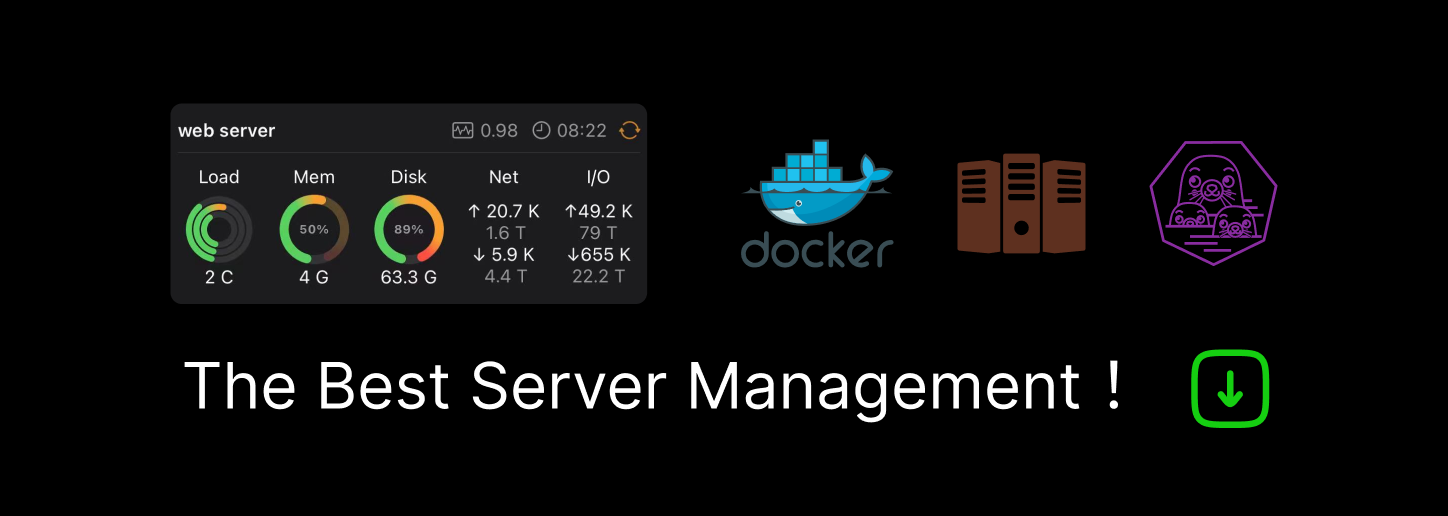
作者:小傅哥
博客:https://bugstack.cn
沉淀、分享、成长,让自己和他人都能有所收获!😄
布隆过滤器的历史
布隆过滤器由 Burton Howard Bloom 于 1970 年提出,它是一种节省空间的概率数据结构,包括一个很长的二进制向量和一些列随机映射函数。
二、布隆过滤器结构
布隆过滤器是一个基于数组和哈希函数散列元素的结构,很像HashMap的哈希桶。布隆过滤器可以用于检测一个元素是否在集合中。它的优点是空间效率和查询时间比一般算法要好很多,但也有一定概率的误判性。如HashMap出现哈希碰撞💥
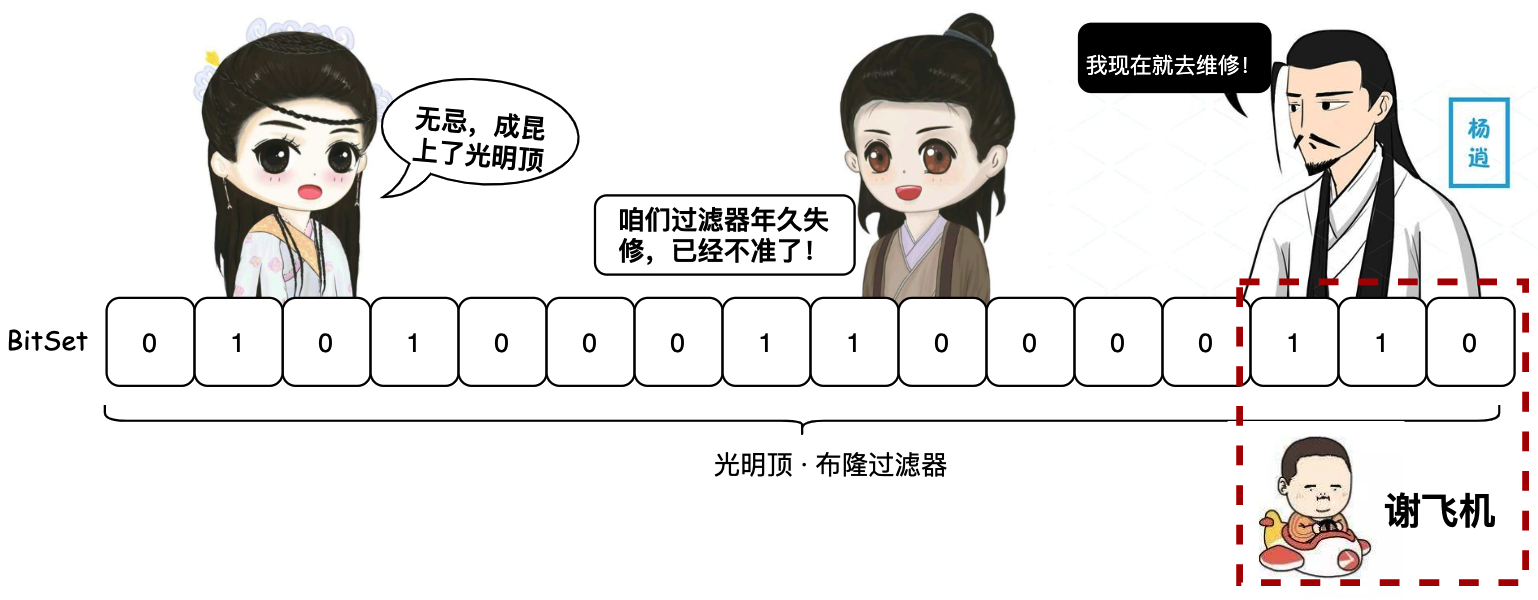
- 赵敏:无忌,成昆上了光明顶!
- 张无忌:咱们过滤器年久失修,已经不准了!
- 张无忌:布隆过滤器的长度太小,哈希计算单一。导致谢飞机、拎瓢冲、成昆,三个人的哈希值都是相同的,所以没法判断成昆是否上了光明顶。咱们只能快些上山了,沿途小心。
- 杨左使:老大,我现在就去维修一下。布隆过滤器的优化方式可以通过增加长度和多样新计算哈希解决。
三、布隆过滤器实现
布隆过滤器的实现条件包括可以存放二进制元素的 BitSet 以及多样性的哈希计算函数。
public class BloomFilter {
private static final HashGenerator.HashGroup[] GROUPS = new HashGenerator.HashGroup[]{HashGenerator.HashGroup.G1, HashGenerator.HashGroup.G2, HashGenerator.HashGroup.G3, HashGenerator.HashGroup.G4};
private final BitSet bits;
private HashGenerator[] generators;
}
所有的元素存放都经过多样的哈希计算存放到 BitSet 中,这样可以尽可能的分散元素,减少误判性。
1. 哈希函数
private int hashG1(String value) {
int hash = 0;
for (int idx = 0; idx < value.length(); idx++) {
char c = value.charAt(idx);
hash = (hash << 5) + hash + c;
hash &= hash;
hash = Math.abs(hash);
}
return hash % (seed * size - 1);
}
private int hashG2(String value) {
int hash = 7397;
for (int idx = 0; idx < value.length(); idx++) {
char c = value.charAt(idx);
hash = (hash << 5) + hash + c;
}
return Math.abs(hash % seed * (size - 1));
}
private int hashG3(String value) {
int hash = 0;
for (int idx = 0; idx < value.length(); idx++) {
char c = value.charAt(idx);
hash = (hash << 5) + hash + c;
hash += c;
hash &= hash;
}
return Math.abs(hash % (seed * size - 1));
}
private int hashG4(String value) {
int h;
return (value == null) ? 0 : Math.abs(seed * (size - 1) & ((h = value.hashCode()) ^ (h >>> 16)));
}
- 这里提供了四种哈希计算的方式,相当于每一个哈希计算都是一次扰动处理。一个元素的存放可以经过四次哈希,尽量让元素值做到散列。
2. 构建容器
public BloomFilter(int size, int[] seeds) {
bits = new BitSet(size);
generators = new HashGenerator[seeds.length];
for (int i = 0; i < seeds.length; i++) {
generators[i] = new HashGenerator(size, seeds[i], GROUPS[i % GROUPS.length]);
}
}
- 构造函数根据所需创建的容器大小和哈希种子来初始化布隆过滤器。
3. 添加元素
public void add(String value) {
for (HashGenerator generator : generators) {
int hash = generator.doHash(value);
bits.set(hash, true);
}
}
- 添加元素时按照元素初始化时的哈希计算种类,获取哈希并存放。
4. 比对元素
public boolean contains(String value) {
boolean ret = true;
for (HashGenerator generator : generators) {
ret = ret && bits.get(generator.doHash(value));
}
return ret;
}
- 比对元素时用的是同一类哈希计算方式,并且把这些哈希值
&&
计算。用N个比特位置记录一个值更准确
四、布隆过滤器测试
单元测试
@Test
public void test() {
String val00 = "小傅哥";
String val01 = "https://bugstack.cn";
String val02 = "https://github.com/fuzhengwei/CodeGuide";
String val03 = "https://github.com/fuzhengwei";
BloomFilter filter = new BloomFilter(Integer.MAX_VALUE, new int[]{7, 19, 43, 77});
filter.add(val00);
filter.add(val01);
filter.add(val02);
logger.info("测试结果 val00:{} 布隆过滤器:{}", val00, filter.contains(val00));
logger.info("测试结果 val01:{} 布隆过滤器:{}", val01, filter.contains(val01));
logger.info("测试结果 val02:{} 布隆过滤器:{}", val02, filter.contains(val02));
logger.info("测试结果 val02:{} 布隆过滤器:{}", val03, filter.contains(val03));
}
- 可以看到这里初始化了一个比较大的布隆过滤器,并且提供了4个随机种子;
7, 19, 43, 77
计算哈希值。
测试结果
21:33:22.790 [main] INFO bloom_filter.__test__.BloomFilterTest - 测试结果 val00:小傅哥 布隆过滤器:true
21:33:22.794 [main] INFO bloom_filter.__test__.BloomFilterTest - 测试结果 val01:https://bugstack.cn 布隆过滤器:true
21:33:22.794 [main] INFO bloom_filter.__test__.BloomFilterTest - 测试结果 val02:https://github.com/fuzhengwei/CodeGuide 布隆过滤器:true
21:33:22.795 [main] INFO bloom_filter.__test__.BloomFilterTest - 测试结果 val02:https://github.com/fuzhengwei 布隆过滤器:false
Process finished with exit code 0
- 通过测试可以看到,存放的val00、val01、val02 分别可以验证出 true 没有存放的 val03 验证为fasle
五、常见面试题
- 布隆过滤器的使用场景?
- 布隆过滤器的实现原理和方式?
- 如何提高布隆过滤器的准确性?
- 有哪些中哈希计算方式?
- 都有哪些类型的布隆过滤器实现?Google 开源的 Guava 中自带的布隆过滤器、Redis 中的布隆过滤器(https://github.com/RedisBloom/RedisBloom)
Recommend
About Joyk
Aggregate valuable and interesting links.
Joyk means Joy of geeK