

手把手教你使用LabVIEW OpenCV dnn实现图像分类(含源码) - virobotics
source link: https://www.cnblogs.com/virobotics/p/16768570.html
Go to the source link to view the article. You can view the picture content, updated content and better typesetting reading experience. If the link is broken, please click the button below to view the snapshot at that time.
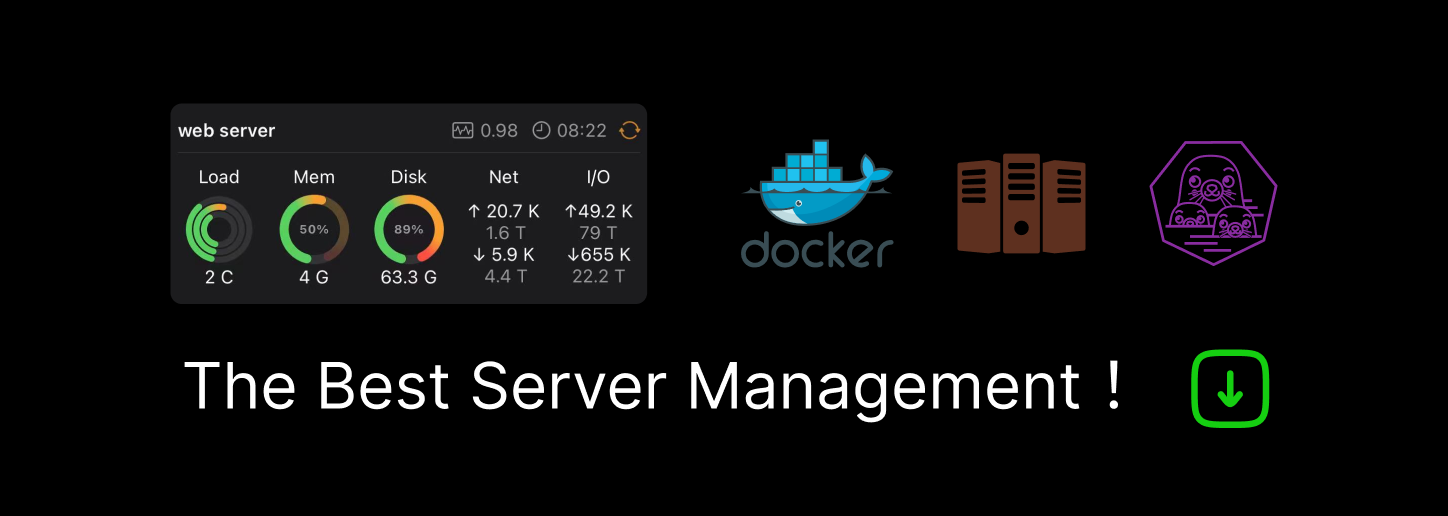
上一篇和大家一起分享了如何使用LabVIEW OpenCV dnn实现手写数字识别,今天我们一起来看一下如何使用LabVIEW OpenCV dnn实现图像分类。
一、什么是图像分类?
1、图像分类的概念
图像分类,核心是从给定的分类集合中给图像分配一个标签的任务。实际上,这意味着我们的任务是分析一个输入图像并返回一个将图像分类的标签。标签总是来自预定义的可能类别集。
示例:我们假定一个可能的类别集categories = {dog, cat, eagle},之后我们提供一张图片(下图)给分类系统。这里的目标是根据输入图像,从类别集中分配一个类别,这里为eagle,我们的分类系统也可以根据概率给图像分配多个标签,如eagle:95%,cat:4%,panda:1%
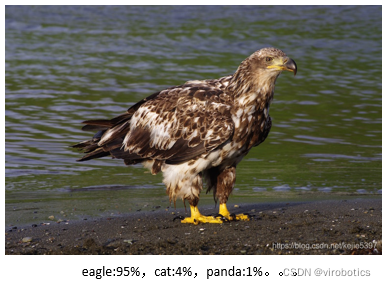
2、MobileNet简介
MobileNet:基本单元是深度级可分离卷积(depthwise separable convolution),其实这种结构之前已经被使用在Inception模型中。深度级可分离卷积其实是一种可分解卷积操作(factorized convolutions),其可以分解为两个更小的操作:depthwise convolution和pointwise convolution,如图1所示。Depthwise convolution和标准卷积不同,对于标准卷积其卷积核是用在所有的输入通道上(input channels),而depthwise convolution针对每个输入通道采用不同的卷积核,就是说一个卷积核对应一个输入通道,所以说depthwise convolution是depth级别的操作。而pointwise convolution其实就是普通的卷积,只不过其采用1x1的卷积核。图2中更清晰地展示了两种操作。对于depthwise separable convolution,其首先是采用depthwise convolution对不同输入通道分别进行卷积,然后采用pointwise convolution将上面的输出再进行结合,这样其实整体效果和一个标准卷积是差不多的,但是会大大减少计算量和模型参数量。
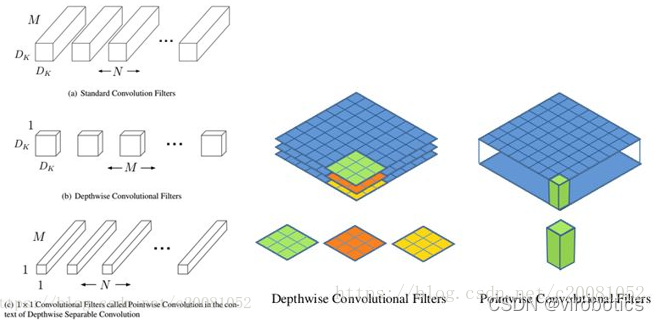
MobileNet的网络结构如表所示。首先是一个3x3的标准卷积,然后后面就是堆积depthwise separable convolution,并且可以看到其中的部分depthwise convolution会通过strides=2进行down sampling。然后采用average pooling将feature变成1x1,根据预测类别大小加上全连接层,最后是一个softmax层。如果单独计算depthwise convolution和pointwise convolution,整个网络有28层(这里Avg Pool和Softmax不计算在内)。
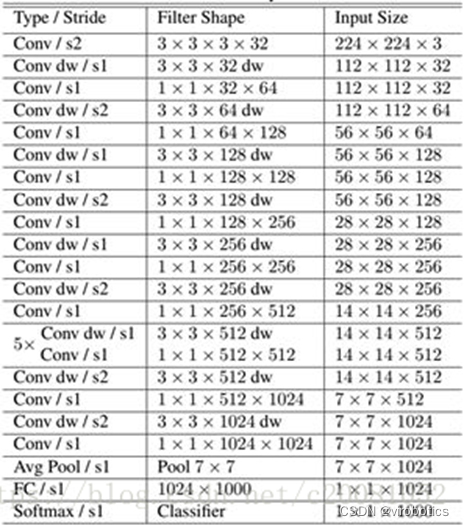
二、使用python实现图像分类(py_to_py_ssd_mobilenet.py)
1、获取预训练模型
- 使用tensorflow.keras.applications获取模型(以mobilenet为例);
from tensorflow.keras.applications import MobileNet
original_tf_model = MobileNet(
include_top=True,
weights="imagenet"
)
- 把original_tf_model打包成pb
def get_tf_model_proto(tf_model):
# define the directory for .pb model
pb_model_path = "models"
# define the name of .pb model
pb_model_name = "mobilenet.pb"
# create directory for further converted model
os.makedirs(pb_model_path, exist_ok=True)
# get model TF graph
tf_model_graph = tf.function(lambda x: tf_model(x))
# get concrete function
tf_model_graph = tf_model_graph.get_concrete_function(
tf.TensorSpec(tf_model.inputs[0].shape, tf_model.inputs[0].dtype))
# obtain frozen concrete function
frozen_tf_func = convert_variables_to_constants_v2(tf_model_graph)
# get frozen graph
frozen_tf_func.graph.as_graph_def()
# save full tf model
tf.io.write_graph(graph_or_graph_def=frozen_tf_func.graph,
logdir=pb_model_path,
name=pb_model_name,
as_text=False)
return os.path.join(pb_model_path, pb_model_name)
2、使用opencv_dnn进行推理
- 图像预处理(blob)
def get_preprocessed_img(img_path):
# read the image
input_img = cv2.imread(img_path, cv2.IMREAD_COLOR)
input_img = input_img.astype(np.float32)
# define preprocess parameters
mean = np.array([1.0, 1.0, 1.0]) * 127.5
scale = 1 / 127.5
# prepare input blob to fit the model input:
# 1. subtract mean
# 2. scale to set pixel values from 0 to 1
input_blob = cv2.dnn.blobFromImage(
image=input_img,
scalefactor=scale,
size=(224, 224), # img target size
mean=mean,
swapRB=True, # BGR -> RGB
crop=True # center crop
)
print("Input blob shape: {}\n".format(input_blob.shape))
return input_blob
- 调用pb模型进行推理
def get_tf_dnn_prediction(original_net, preproc_img, imagenet_labels):
# inference
preproc_img = preproc_img.transpose(0, 2, 3, 1)
print("TF input blob shape: {}\n".format(preproc_img.shape))
out = original_net(preproc_img)
print("\nTensorFlow model prediction: \n")
print("* shape: ", out.shape)
# get the predicted class ID
imagenet_class_id = np.argmax(out)
print("* class ID: {}, label: {}".format(imagenet_class_id, imagenet_labels[imagenet_class_id]))
# get confidence
confidence = out[0][imagenet_class_id]
print("* confidence: {:.4f}".format(confidence))
3、实现图像分类 (代码汇总)
import os
import cv2
import numpy as np
import tensorflow as tf
from tensorflow.keras.applications import MobileNet
from tensorflow.python.framework.convert_to_constants import convert_variables_to_constants_v2
def get_tf_model_proto(tf_model):
# define the directory for .pb model
pb_model_path = "models"
# define the name of .pb model
pb_model_name = "mobilenet.pb"
# create directory for further converted model
os.makedirs(pb_model_path, exist_ok=True)
# get model TF graph
tf_model_graph = tf.function(lambda x: tf_model(x))
# get concrete function
tf_model_graph = tf_model_graph.get_concrete_function(
tf.TensorSpec(tf_model.inputs[0].shape, tf_model.inputs[0].dtype))
# obtain frozen concrete function
frozen_tf_func = convert_variables_to_constants_v2(tf_model_graph)
# get frozen graph
frozen_tf_func.graph.as_graph_def()
# save full tf model
tf.io.write_graph(graph_or_graph_def=frozen_tf_func.graph,
logdir=pb_model_path,
name=pb_model_name,
as_text=False)
return os.path.join(pb_model_path, pb_model_name)
def get_preprocessed_img(img_path):
# read the image
input_img = cv2.imread(img_path, cv2.IMREAD_COLOR)
input_img = input_img.astype(np.float32)
# define preprocess parameters
mean = np.array([1.0, 1.0, 1.0]) * 127.5
scale = 1 / 127.5
# prepare input blob to fit the model input:
# 1. subtract mean
# 2. scale to set pixel values from 0 to 1
input_blob = cv2.dnn.blobFromImage(
image=input_img,
scalefactor=scale,
size=(224, 224), # img target size
mean=mean,
swapRB=True, # BGR -> RGB
crop=True # center crop
)
print("Input blob shape: {}\n".format(input_blob.shape))
return input_blob
def get_imagenet_labels(labels_path):
with open(labels_path) as f:
imagenet_labels = [line.strip() for line in f.readlines()]
return imagenet_labels
def get_opencv_dnn_prediction(opencv_net, preproc_img, imagenet_labels):
# set OpenCV DNN input
opencv_net.setInput(preproc_img)
# OpenCV DNN inference
out = opencv_net.forward()
print("OpenCV DNN prediction: \n")
print("* shape: ", out.shape)
# get the predicted class ID
imagenet_class_id = np.argmax(out)
# get confidence
confidence = out[0][imagenet_class_id]
print("* class ID: {}, label: {}".format(imagenet_class_id, imagenet_labels[imagenet_class_id]))
print("* confidence: {:.4f}\n".format(confidence))
def get_tf_dnn_prediction(original_net, preproc_img, imagenet_labels):
# inference
preproc_img = preproc_img.transpose(0, 2, 3, 1)
print("TF input blob shape: {}\n".format(preproc_img.shape))
out = original_net(preproc_img)
print("\nTensorFlow model prediction: \n")
print("* shape: ", out.shape)
# get the predicted class ID
imagenet_class_id = np.argmax(out)
print("* class ID: {}, label: {}".format(imagenet_class_id, imagenet_labels[imagenet_class_id]))
# get confidence
confidence = out[0][imagenet_class_id]
print("* confidence: {:.4f}".format(confidence))
def main():
# configure TF launching
#set_tf_env()
# initialize TF MobileNet model
original_tf_model = MobileNet(
include_top=True,
weights="imagenet"
)
# get TF frozen graph path
full_pb_path = get_tf_model_proto(original_tf_model)
print(full_pb_path)
# read frozen graph with OpenCV API
opencv_net = cv2.dnn.readNetFromTensorflow(full_pb_path)
print("OpenCV model was successfully read. Model layers: \n", opencv_net.getLayerNames())
# get preprocessed image
input_img = get_preprocessed_img("yaopin.png")
# get ImageNet labels
imagenet_labels = get_imagenet_labels("classification_classes.txt")
# obtain OpenCV DNN predictions
get_opencv_dnn_prediction(opencv_net, input_img, imagenet_labels)
# obtain TF model predictions
get_tf_dnn_prediction(original_tf_model, input_img, imagenet_labels)
if __name__ == "__main__":
main()
三、使用LabVIEW dnn实现图像分类(callpb_photo.vi)
本博客中所用实例基于LabVIEW2018版本,调用mobilenet pb模型
1、读取待分类的图片和pb模型
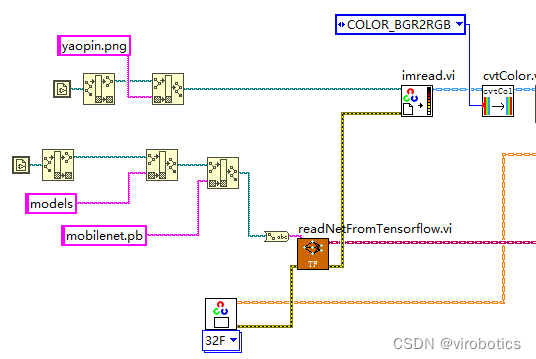
2、将待分类的图片进行预处理
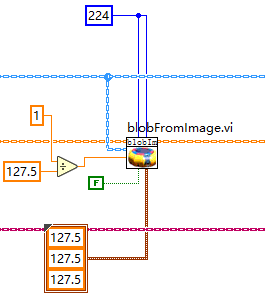
3、将图像输入至神经网络中并进行推理
4、实现图像分类

5、总体程序源码:
按照如下图所示程序进行编码,实现图像分类,本范例中使用了一分类,分类出置信度最高的物体。
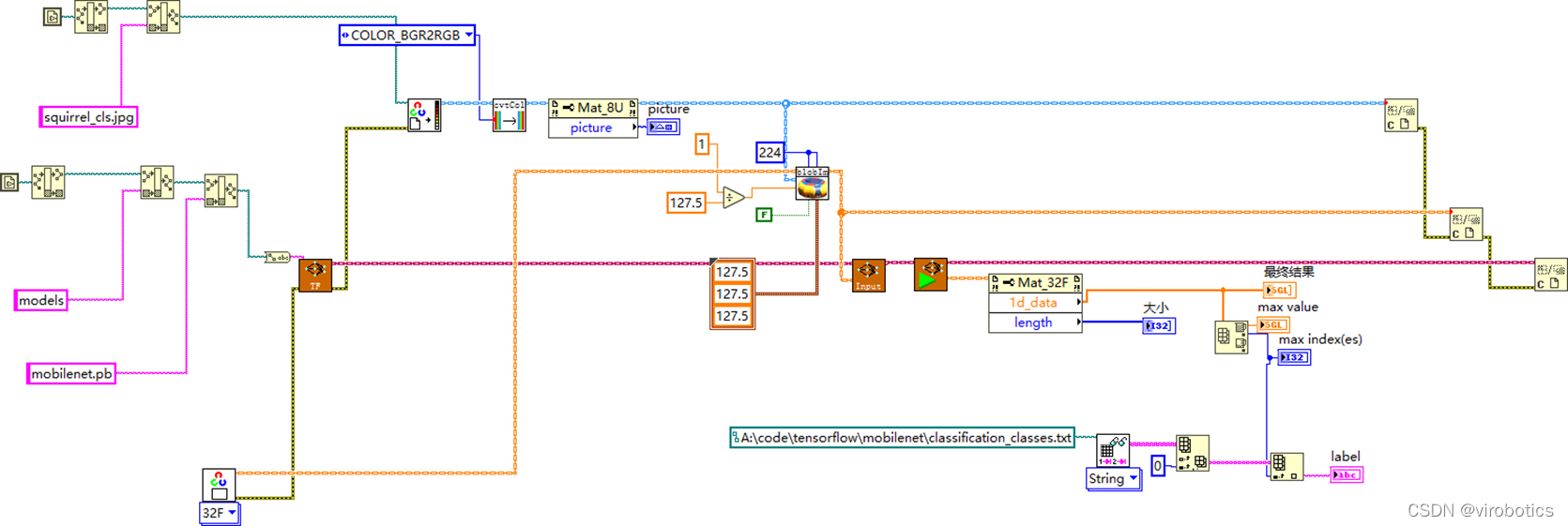
如下图所示为加载药瓶图片得到的分类结果,在前面板可以看到图片和label:
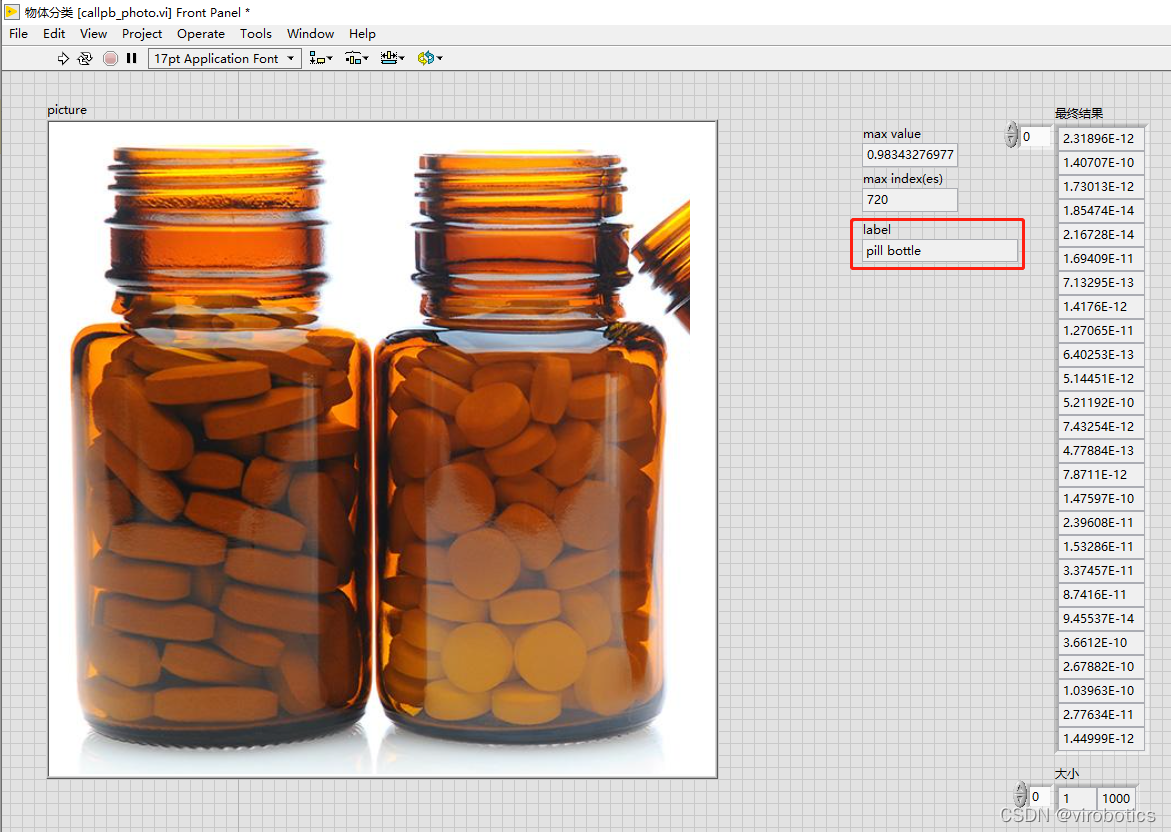
四、源码下载
链接:https://pan.baidu.com/s/10yO72ewfGjxAg_f07wjx0A?pwd=8888
提取码:8888
更多关于LabVIEW与人工智能技术,可添加技术交流群进一步探讨。qq群号:705637299,请备注暗号:LabVIEW 机器学习
Recommend
About Joyk
Aggregate valuable and interesting links.
Joyk means Joy of geeK