
1

记一次 Sedona(GeoSpark) 空间计算优化 - 明月照江江
source link: https://www.cnblogs.com/gradyblog/p/16663184.html
Go to the source link to view the article. You can view the picture content, updated content and better typesetting reading experience. If the link is broken, please click the button below to view the snapshot at that time.
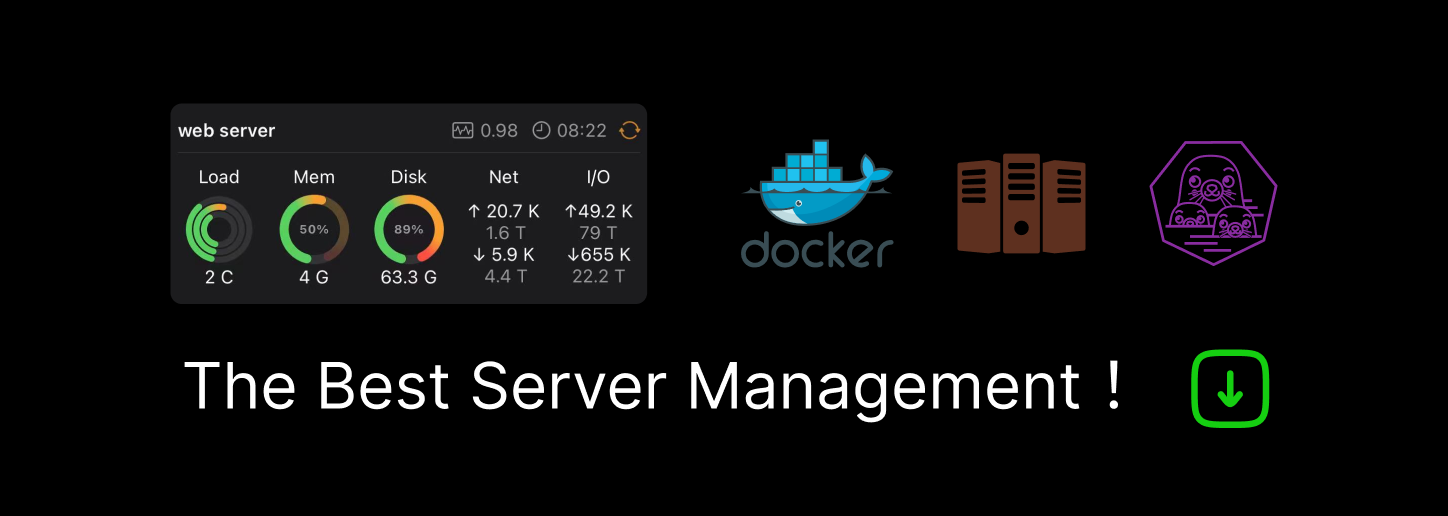
项目需求需要空间计算能力,开始选型Sedona(GeoSpark)来完成,
需求需要每一条数据在满足某条件的情况下,去查找某张表进行空间匹配,找到离这个点(point)最近的一条道路(lineString)
第一个方案: 使用sedona来使用临近道路的判断
由于sedona本质还是使用spark的能力,所以遵循spark的开发规则,不能在rdd.map
里面干活,sedona也不支持批量查,只能一条一条匹配。 伪代码如下
val spatial_sql =
"""
| select
| ST_GeomFromWKT(geom) geom, name, adcode
| from ods.ods_third_party_road_data
|""".stripMargin
val third_party_road_df = spark.sql(spatial_sql).toDF()
aoi_day_s_df.rdd.collect().par.map(row => {
val tmp_location = row.getAs[String]("poi_location")
val near_street = spatialQueryStreet(third_party_road_df, city_code, tmp_location)
println(near_street)
...
)
def spatialQueryStreet(third_party_road_df:DataFrame, city_code:String, location: String): String = {
val frame = third_party_road_df.where("adcode = '%s'".format(city_code)).toDF()
val tp_road_spatial_rdd = Adapter.toSpatialRdd(frame, "geom")
tp_road_spatial_rdd.buildIndex(IndexType.RTREE, false)
val geometryFactory = new GeometryFactory()
val x = location.substring(location.indexOf("(") + 1, location.indexOf(" "))
val y = location.substring(location.indexOf(" ") + 1, location.indexOf(")"))
val pointObject = geometryFactory.createPoint(new Coordinate(x.toDouble, y.toDouble))
val usingIndex = true
val result = KNNQuery.SpatialKnnQuery(tp_road_spatial_rdd, pointObject, 1, usingIndex)
if (result.isEmpty) {
return ""
} else {
val dst = result.get(0)
//System.out.println("==== dst.getUserData: " + dst.getUserData.toString)
val strings = dst.getUserData.toString.split("\t")
val near_street = strings(0)
//System.out.println("==== near_street: " + near_street)
near_street
}
结果效率不高,因为每条数据都要匹配,sedona又不能在rdd.map
中使用,所以必须先collect().map
,这就不能利用到spark多节点并行的特性; 2. 每条数据都基于third_party_road_df
创建了空间索引来查,效率更低了(如果只有一条数据还勉强可以接受)
方案2: 改sedona为JTS来处理,jts直接创建rtree,可以在rdd.map
中处理,而且创建速度也更快一些,效率更高了
伪代码如下
poi_build_aoi_aoi_day_s_df.rdd.map(row => {
val tmp_location = row.getAs[String]("poi_location")
val rtree = createRtree(model_list)
near_street = spatialQueryStreet(rtree, tmp_location)
println(near_street)
...
)
def createRtree(third_party_road_list: Array[ThirdPartyModel]): STRtree = {
val rtree = new STRtree()
for (model <- third_party_road_list) {
val geom = model.geometry
geom.setUserData(model.name)
rtree.insert(geom.getEnvelopeInternal, model.geometry)
}
rtree.build()
rtree
}
def spatialQueryStreet(rtree: STRtree, location: String): String = {
if (rtree == null) {
""
}
val geometryFactory = new GeometryFactory()
val x = location.substring(location.indexOf("(") + 1, location.indexOf(" "))
val y = location.substring(location.indexOf(" ") + 1, location.indexOf(")"))
val pointObject = geometryFactory.createPoint(new Coordinate(x.toDouble, y.toDouble))
val result = rtree.nearestNeighbour(pointObject.getEnvelopeInternal, pointObject, new GeometryItemDistance())
val name = result.asInstanceOf[Geometry].getUserData.asInstanceOf[String]
println(s"nearestNeighbour name: $name")
name
}
通过这次修改,由原来跑3个小时(甚至更多)的任务在15分钟内就跑完了
PS: 经尝试rtree
不能通过广播变量发送出去,会报序列化异常
其实还可以再优化一下,上面每条数据还是创建了一次rtree
, 可以改为mapPartition
,然后只建一次rtree
, 数据量大时效果更佳
aoi_day_s_df.rdd.mapPartitions(iterator => {
// rtree 放到iterator.map 外面创建,搞一次就ok了,更快(不过我没有试验,应该是百分百可行的)
val rtree = createRtree(model_list)
val seq = iterator.map(row => {
val tmp_location = row.getAs[String]("poi_location")
near_street = spatialQueryStreet(rtree, tmp_location)
println(near_street)
...
)
seq
)
Recommend
About Joyk
Aggregate valuable and interesting links.
Joyk means Joy of geeK