

Mature questions around organizational data use - IDC study takes a fresh look a...
source link: https://diginomica.com/mature-questions-around-organizational-data-use-idc-study-takes-fresh-look-some-longstanding-issues
Go to the source link to view the article. You can view the picture content, updated content and better typesetting reading experience. If the link is broken, please click the button below to view the snapshot at that time.
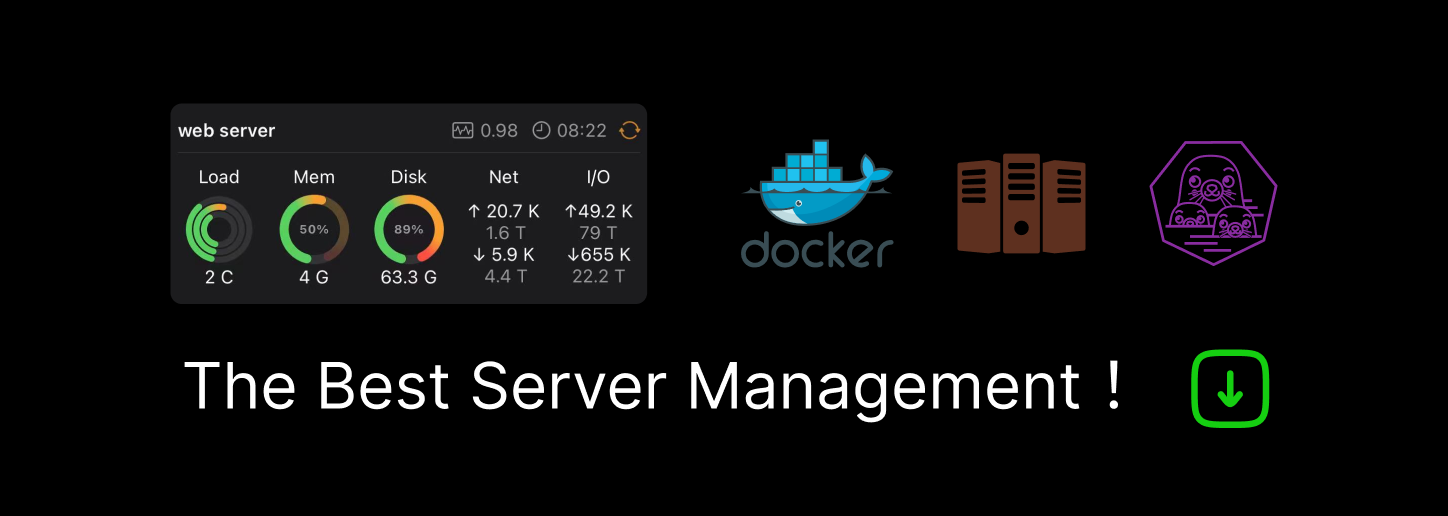
Mature questions around organizational data use - IDC study takes a fresh look at some longstanding issues

Rachel Obstler
Do you want to see over 3X improvement in revenue with shorter time to market, greater profit, and exceptional customer loyalty? Then it’s time to take a long, hard look at how data mature your organization is.
That’s one of the underlying conclusions of a new white paper from IDC - How Data Maturity and Product Analytics Improve Digital Experiences and Business Outcomes - that concludes that data maturity - AKA how well an organization is able to use data in its decision making - can provide up to 2.5X increase in overall business outcomes for leading teams versus lagging teams. Revenue generation is particularly improved, some 3.2X greater for the most mature companies, who also saw 2.4X times greater profit.
Some context - the findings are based on a survey of 626 product builders and data scientists from organizations across the US, UK and Canada that sell a digital product or service, sell products or services via their own branded e-commerce site, or both. The study was commissioned by analytics firm Heap.
As per Heap’s official release, top level findings include:
- 98% of leaders have a good-to-excellent understanding of customer journey friction points, while only 29% of the least data mature companies can make the same claim.
- 84% of leader teams get answers in minutes or hours compared to only three percent of lagging.
- 76% of leaders have a single source of truth for data compared to only three percent of lagging firms.
- 89% of leading teams agree or strongly agree their organization celebrates learning from experimentation while only 23% of lagging teams have the same level of conviction.
- Over 80% of leaders have fully-automated data validation, have clearly-defined policies on who can access data, and have the ability to control data management.
- 62% of leaders have “complete” or “strong” access to data, while only 38% of lagging organizations have this same.
- 73% of leading companies believe that they can do more with the data that is available to them.
People/Process/Technology
Prior to the release of the study results today, I caught up with Rachel Obstler, EVP of Product at Heap, to get her perspective on the main takeaways, as well as some thoughts on wider data analytics topics. The study used a people/process/technology format, she told me:
The idea was, looking at all of those things, what were respondents behaviors around the technology that they had in place? There were questions we asked like, 'How long does it take you to answer a question?'. A more mature organization has a situation where their users can answer questions in minutes or hours, not days or weeks or months. Then there's process. We asked questions like, 'Do you have a single source of truth?' and we found that in the more mature organizations, 76% of them had a single source of truth.
We asked a bunch of questions about culture. An example of that is, 'How often is a decision made by the 'hippo', the highest paid person in the room. We found that 44% of decisions are made by the 'hippo', but if you look at the low maturity bucket, it went up to like 69%. We basically took a set of questions and said, 'Hey, these are mature behaviors, these indicate mature behaviors', and so bucketed them into the different maturity levels and then you can see all these differences in these ways.
There are certain questions that are always begged in this sort of study. For example, can we be certain that those who claim to have a single source of truth have indeed captured this particular ‘Holy Grail’? There’s inevitably a degree of self-validation that comes into play at this point. But leaving that to one side, one aspect of any research into organizational data use/leverage is the cynical view that people will always want more data, even when having that isn’t always the best thing for them. We’ve long talked about the data deficit that many organizations operate under, but there’s also the danger of data deluge.
So how do organizations know when ‘enough is enough’ and be able to strike the optimum balance? Obstler points to a couple of questions in the study that approach that aspect:
One of them is we asked if respondents were leveraging the data that they had effectively? 73% of the leading mature companies felt like they could do more with the data that they already had. That gets at that drowning in data issue. The other thing that we asked about is we said, 'Do you have access to all the data that you need and what types of data do you wish you had?'. Some of the feedback on that was that 65% of the lagging companies felt like they didn't have access to the likes of session replay data, or the ability to identify the specific points of friction.
Frictionless
Talk of friction brings us into the orbit of Phil Wainewright’s ongoing work on the Frictionless Enterprise which Obstler cited as “very related”:
You have to have data access. This is part of the data culture piece. It's about making sure that you do provide access to everyone, you provide the right tooling, the self-service tooling, so that any user can answer a question that they need to answer within minutes.
But there’s a but here:
There's a lot of different tools and it's very hard for users to to get the answers that they want when they have some data in this tool over here and some data in this tool over there. Also, the idea of analytics is so divorced from the product and the experience itself. That shouldn't be. So, one of the things that Heap is doing is putting together these things, so that you find a point of friction and use quantitative analysis, but then be able to immediately watch this experience.
There's just a lot of broken workflows. The fix to this is to be able to enable the individual users that are responsible for these feature teams..They need to have access to this data that's sitting in an executive’s computer that's useless. As a product leader, I'm always thinking about how do I enable my team? The most important thing I can do is enable my team and give them access to the data that they need to do their jobs.
That said, it’s interesting to see from the study that over 45% of all companies only use data to measure success or failure on major initiatives. Is that a high enough percentage? Obstler argued:
There's always exceptions where a call to action is below the fold and you probably don't need data to tell you to move up the fold. But it's still nice to have it and it's also still nice to see how big of an impact a change made because you always have to prioritize changes, so you really should be measuring the impact of everything that you do.
Empowering
In fact, nearly three-quarters of leading companies believe they could do more with the data available to them. How then do they improve their situations? In response, Obstler pointed to her former role at another tech firm, PagerDuty, a company that was on the forefront of the shift to a DevOps operating style and the idea that engineers should build and operate their code, with feature teams that are really self-actuated and empowered:
What really got it to happen is, I think three things. Number one, some people did it and they were hugely successful and so it was measurable. Number two, the tools were in place. Just taking the example of PagerDuty, if you tell an engineer they have to own and operate their code, but there's nothing that makes it easy for them to know when there's a problem or not a problem, that's not tenable. So, the tooling that is necessary needs to be in place. And then the third one is the culture. But you can't force the culture if those other two things aren't also in place, so they all have to happen at the same time and together.
On the culture front, while the accepted wisdom is that everyone wants to be able to access data, is there in reality a possibility that employees who are not data scientists can be nervous when presented with data responsibilities? There’s certainly a huge data trust issue to be tackled, said Obstler:
Just talking to customers, seeing what's going on in the market, seeing what the challenges are, it is very difficult when you are, let's say, a product manager and you want to measure something and you have to say to someone, 'I want to measure this stuff'. Then an engineer has to go implement the measurement and you get back a bunch of data and you have no idea if it really is doing what you intended or not. How do you even validate to begin with? There's just too many points of contact, there's too much in there.
The idea of data trust at least partially needs to be solved by the user being able to viscerally understand the metric that they're looking at. I can see what this represents in the product. I can play it and watch it happening. I see exactly what this data represents. I can visualize it. What is needed is for people to be able to trust the data. You can spend all the time you want validating it. You can have an army of people who are data scientists or data analysts who are going through validating data, but the reality is, that takes time. It's probably not worthwhile for every single data point.
When you're a product manager, you may be just wanting to fix one little thing. You only need to look at it once, measure it, fix it, see if it worked and then you're done with that data point. You may not need to look at that precise thing. Having an army of people constantly validating data, it's just very inefficient. So, the user needs to be able to viscerally understand and trust the data they're working with.
There also needs to be a willingness to experiment and be ready to fail as well as win. This is an area where a lot of organizations fall short, concluded Obstler:
Do you celebrate learnings from experiments, whether they're successful or failed? That's the whole point of an experiment - it may not work. 89% of leading teams celebrate these learnings. 77% of the lagging teams do not celebrate. But if you expect every experiment to work, it's not an experiment. The whole idea is you need to try things. You don't always know which outcome is going to work. This is a really hard thing for feature teams to do. They have to plan their work and plan their outcomes. Let's say the quarterly goal is, let's move this metric by five percent, but they don't know 100% how they're going to do it. They need to make sure that they plan for, let's say, time for five experiments during the quarter, three of which they think are going to work and the two that don't work are things that they should learn from so that they don't make those mistakes.
My take
The IDC study is a wide-ranging white paper that takes in a lot of important topics. Heap’s latest piece for diginomica tees up a lot of those questions, but there’s a lot of meat on the bones of the full report also to digest. As I commented to Obstler during our conversation, some of these topics feel as though we’ve been talking about them for decades. The difference now is the evolution of data analytics tech to the point where we might finally find it easier to put our houses in order.
Recommend
About Joyk
Aggregate valuable and interesting links.
Joyk means Joy of geeK