

UX Research: How Google's LaMDA Outperforms | UX Collective
source link: https://uxdesign.cc/google-wants-you-to-test-lamda-how-ux-research-can-help-it-outperform-1a1f0f973389
Go to the source link to view the article. You can view the picture content, updated content and better typesetting reading experience. If the link is broken, please click the button below to view the snapshot at that time.
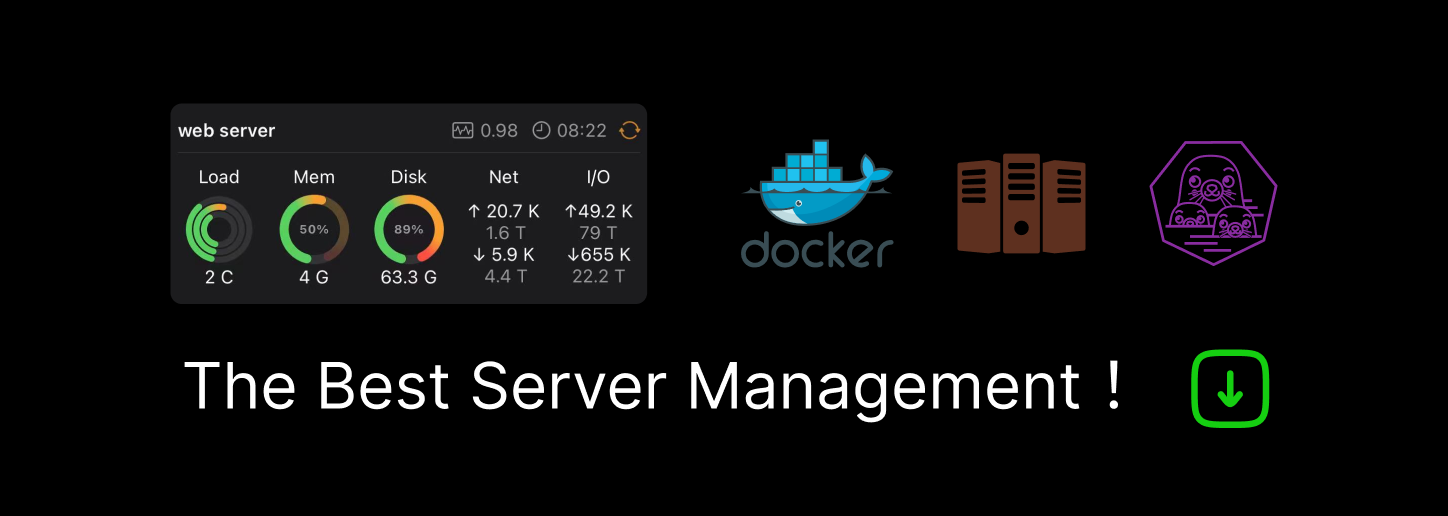
Google wants you to test LaMDA; how UX research can help it outperform
UX research solved the puzzle of how to interview and understand users; it can have an influential role in building the future of LaMDA.

Created by the Author using Midjourney
If LaMDA is going to make its way into the open market, it needs to give users the ability to conduct targeted tests before doing so.
They are really considering carrying out their plans to do just this.
Right now is the perfect time for Google to integrate end-to-end UX research approaches to build LaMDA, what it is today and its future.
Before I delve further, here are the instructions on how you can join the waitlist to test LaMDA: visit this site.

At this link
As of today, you can only “register your interest,” get on the waiting list, and wait. Interestingly, the idea behind the testing phase for LaMDA is to provide you with three use cases: (1) LaMDA will orient you around a physical location; (2) it can potentially give you an outline of topics and idea for you to further decompose; and (3) you can simply have a conversation (an “open-ended” chat) with it.
Background
First, we were told that LaMDA has consciousness. The public was led to think that it had reached the level of human abilities whereby it not only understood its own existence, it also felt happiness and sadness.
Google moved quickly to take action. While we will never have access to complete information on what transpired within the organization, the fact that they fired Blake Lemoine for claiming that LaMDA had gained sentience was something that became public knowledge.
LaMDA theory and its brain, simplified
Google has developed a new neural model called LaMDA that can generate responses to questions in natural language. This is different from traditional methods like computers and people because these models are specifically designed for dialog, meaning they can understand multiple speakers at once and make decisions based on their knowledge. Google studied the benefits of using LaMDA across three key metrics: quality, safety, and groundedness:
— Quality: how sensible, specific, and interesting?
— Safety: how responsible as an AI capability?
— Groundedness: how authoritative and officially factual are its responses?

Visual rendering of its scaling and fine-tuning metrics
Google found that scaling LaMDA improved the quality of outputs, but its improvements on safety and groundedness were far behind human performance. However, combining scaling with fine-tuning significantly improved LaMDAs overall performance although the model still falls below human levels.
Our words, our phrase patterns, and the tone we try to accentuate through our verbal arguments are all received by machine learning algorithms and used to build their entire ecosystem.
This ecosystem entails not just an endeavor to understand the user’s inquiries, but also an attempt to provide suitable answers. Notice here that I did not state the best answers, the most appropriate answers, or the most accurate answers. The ecosystem is trained to adapt to its environment; as such, what it learns is how it responds (generally speaking).
Few-shot learning and zero-shot learning approaches are the ultra-technical areas of AI where question answering is being researched extensively, should you have curiosity to delve deeply into their interworking.
The interplay between UX and LaMDA
The intersection between the type of data used to train LaMDA and how LaMDA should evaluate that data is the decisive factor in what becomes of LAMDA in the future.
UX research has many of the answers on how to train these engines, not only in how LaMDA can analyze user questions, but also how it can problem-solve with the user with follow-up inquiries.
As an illustration, if LaMDA does not understand a question posed by the user or wants the user to reframe their response it already received, it would know how to follow up if it is trained on how to ask questions.
Knowing the relevant methods to apply for asking those questions would support the development and actual tuning of LaMDA. Simple enough. But, this is where it gets complicated.
I recently wrote about framing an approach to interviewing Meta’s BlenderBot 3 where I presented the questions that I used to “interview” BlenderBot 3.
Engineers think in highly logical constructs. When they build a product, they apply networks of reasoning to integrate across its engine. In the case with LaMDA, its potential responses to user enquiries will be informed by the data utilized in its “fine-tuning” stage, the problem space involving the data used to build LaMDA’s question-answering logic.

From the Author: OpenAI assisted with the development of this visual. This is a depiction of “an AI humanoid having an intense debate with a human.”
Teaching LaMDA how to ask questions
My proposal is to educate LaMDA now on how to socialize, follow up with further questions, and comprehend the deeper human language interconnections with just as much rigor and academic discipline. This is all possible, thanks to large language model (LLM) advancements. What is new is integrating different or specific approaches in teaching LaMDA how to socialize.
Especially in design thinking, varied approaches are employed when conducting user research to understand user needs through interviews. Numerous publications on the subject of user experience research have been developed to help with the problem of conducting interviews.
I have built AI models from ground up in the past. I am not talking about simply taking a fine-tuned or pre-trained AI model and use it for some open-ended, free-text use case. Literally from ground up, and end-to-end.
I have a very good understanding of the kinds of outcomes I am capable of generating, which, as a result of the way my algorithmic implementations have been developed, allow for extremely particular responses.
My algorithmic (for my AI program) implementation is able to answer in a manner that is consistent with those fundamental rules I set forth, easily seen based on the approach it takes through which it presents queries. Even though it is using zero-shot learning procedures to respond in conversational chat, which means that it is seeing text in a structure and syntax it was not necessarily (directly) trained on, it is still able to probe further, follow-up accordingly, and find a way to respond.
The naturalness of an AI algorithm’s discussions is determined not just by the data used to train it, but also by how it is taught to think.
Parting Thoughts
If you have any recommendations for this post or suggestions for broadening the subject, I would appreciate hearing from you.
Also, here is my newsletter; I hope you will kindly consider subscribing.
All references are baked into the article.
Recommend
About Joyk
Aggregate valuable and interesting links.
Joyk means Joy of geeK