

以寡治众各个击破,超大文件分片上传之构建基于Vue.js3.0+Ant-desgin+Tornado6纯异步I...
source link: https://v3u.cn/a_id_218
Go to the source link to view the article. You can view the picture content, updated content and better typesetting reading experience. If the link is broken, please click the button below to view the snapshot at that time.
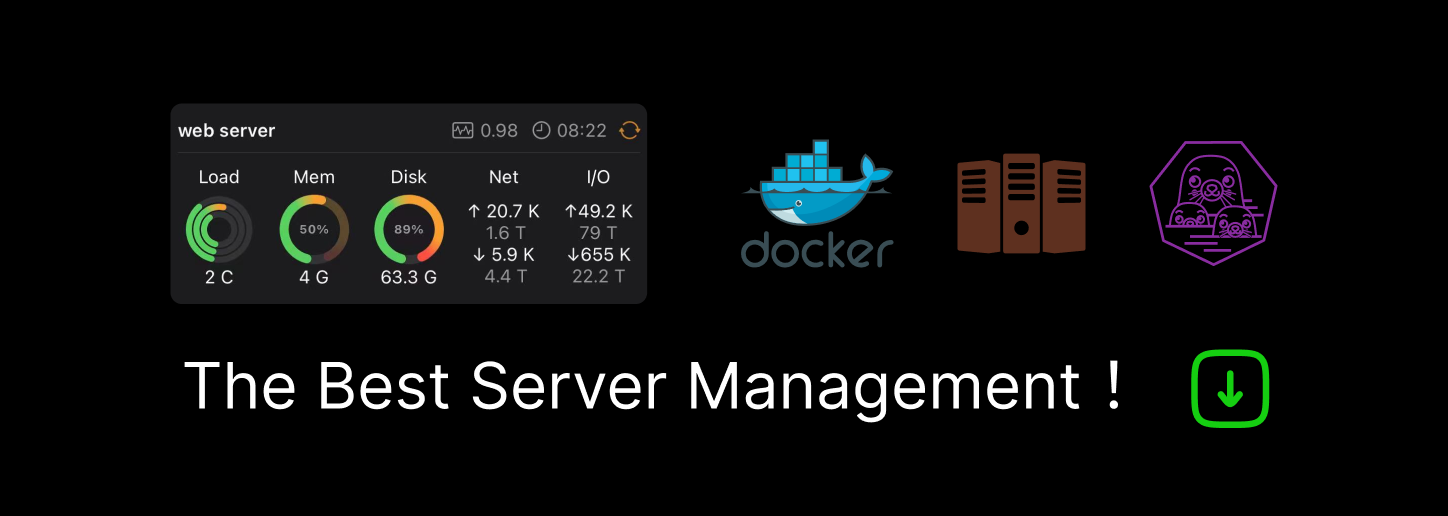
以寡治众各个击破,超大文件分片上传之构建基于Vue.js3.0+Ant-desgin+Tornado6纯异步IO高效写入服务
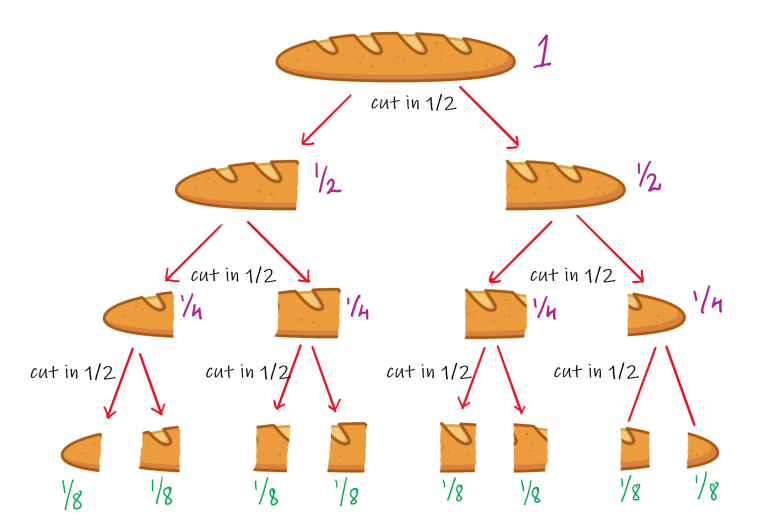
分治算法是一种很古老但很务实的方法。本意即使将一个较大的整体打碎分成小的局部,这样每个小的局部都不足以对抗大的整体。战国时期,秦国破坏合纵的连横即是一种分而治之的手段;十九世纪,比利时殖民者占领卢旺达, 将卢旺达的种族分为胡图族与图西族,以图进行分裂控制,莫不如是。
21世纪,人们往往会在Leetcode平台上刷分治算法题,但事实上,从工业角度上来看,算法如果不和实际业务场景相结合,算法就永远是虚无缥缈的存在,它只会出现在开发者的某一次不经意的面试中,而真实的算法,并不是虚空的,它应该能帮助我们解决实际问题,是的,它应该落地成为实体。
大文件分片上传就是这样一个契合分治算法的场景,现而今,视频文件的体积越来越大,高清视频体积大概2-4g不等,但4K视频的分辨率是标准高清的四倍,需要四倍的存储空间——只需两到三分钟的未压缩4K 电影,或者电影预告片的长度,就可以达到500GB。 8K视频文件更是大得难以想象,而现在12K正在出现,如此巨大的文件,该怎样设计一套合理的数据传输方案?这里我们以前后端分离项目为例,前端使用Vue.js3.0配合ui库Ant-desgin,后端采用并发异步框架Tornado实现大文件的分片无阻塞传输与异步IO写入服务。
首先,安装Vue3.0以上版本:
npm install -g @vue/cli
安装异步请求库axios:
npm install axios --save
随后,安装Ant-desgin:
npm i --save ant-design-vue@next -S
Ant-desgin虽然因为曾经的圣诞节“彩蛋门”事件而声名狼藉,但客观地说,它依然是业界不可多得的优秀UI框架之一。
接着在项目程序入口文件引入使用:
import { createApp } from 'vue'
import App from './App.vue'
import { router } from './router/index'
import axios from 'axios'
import qs from 'qs'
import Antd from 'ant-design-vue';
import 'ant-design-vue/dist/antd.css';
const app = createApp(App)
app.config.globalProperties.axios = axios;
app.config.globalProperties.upload_dir = "https://localhost/static/";
app.config.globalProperties.weburl = "http://localhost:8000";
app.use(router);
app.use(Antd);
app.mount('#app')
随后,参照Ant-desgin官方文档:https://antdv.com/components/overview-cn 构建上传控件:
<a-upload
@change="fileupload"
:before-upload="beforeUpload"
>
<a-button>
<upload-outlined></upload-outlined>
上传文件
</a-button>
</a-upload>
注意这里需要将绑定的before-upload强制返回false,设置为手动上传:
beforeUpload:function(file){
return false;
}
接着声明分片方法:
fileupload:function(file){
var size = file.file.size;//总大小
var shardSize = 200 * 1024; //分片大小
this.shardCount = Math.ceil(size / shardSize); //总片数
console.log(this.shardCount);
for (var i = 0; i < this.shardCount; ++i) {
//计算每一片的起始与结束位置
var start = i * shardSize;
var end = Math.min(size, start + shardSize);
var tinyfile = file.file.slice(start, end);
let data = new FormData();
data.append('file', tinyfile);
data.append('count',i);
data.append('filename',file.file.name);
const axiosInstance = this.axios.create({withCredentials: false});
axiosInstance({
method: 'POST',
url:'http://localhost:8000/upload/', //上传地址
data:data
}).then(data =>{
this.finished += 1;
console.log(this.finished);
if(this.finished == this.shardCount){
this.mergeupload(file.file.name);
}
}).catch(function(err) {
//上传失败
});
}
}
具体分片逻辑是,大文件总体积按照单片体积的大小做除法并向上取整,获取到文件的分片个数,这里为了测试方便,将单片体积设置为200kb,可以随时做修改。
随后,分片过程中使用Math.min方法计算每一片的起始和结束位置,再通过slice方法进行切片操作,最后将分片的下标、文件名、以及分片本体异步发送到后台。
当所有的分片请求都发送完毕后,封装分片合并方法,请求后端发起合并分片操作:
mergeupload:function(filename){
this.myaxios(this.weburl+"/upload/","put",{"filename":filename}).then(data =>{
console.log(data);
});
}
至此,前端分片逻辑就完成了。
后端异步IO写入
为了避免同步写入引起的阻塞,安装aiofiles库:
pip3 install aiofiles
aiofiles用于处理asyncio应用程序中的本地磁盘文件,配合Tornado的异步非阻塞机制,可以有效的提升文件写入效率:
import aiofiles
# 分片上传
class SliceUploadHandler(BaseHandler):
async def post(self):
file = self.request.files["file"][0]
filename = self.get_argument("filename")
count = self.get_argument("count")
filename = '%s_%s' % (filename,count) # 构成该分片唯一标识符
contents = file['body'] #异步读取文件
async with aiofiles.open('./static/uploads/%s' % filename, "wb") as f:
await f.write(contents)
return {"filename": file.filename,"errcode":0}
这里后端获取到分片实体、文件名、以及分片标识后,将分片文件以文件名_分片标识的格式异步写入到系统目录中,以一张378kb大小的png图片为例,分片文件应该顺序为200kb和178kb,如图所示:
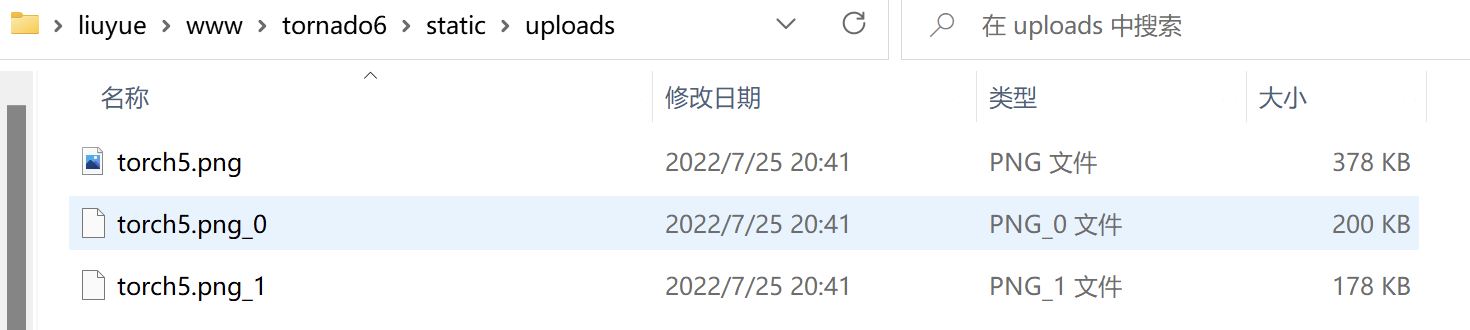
当分片文件都写入成功后,触发分片合并接口:
import aiofiles
# 分片上传
class SliceUploadHandler(BaseHandler):
async def post(self):
file = self.request.files["file"][0]
filename = self.get_argument("filename")
count = self.get_argument("count")
filename = '%s_%s' % (filename,count) # 构成该分片唯一标识符
contents = file['body'] #异步读取文件
async with aiofiles.open('./static/uploads/%s' % filename, "wb") as f:
await f.write(contents)
return {"filename": file.filename,"errcode":0}
async def put(self):
filename = self.get_argument("filename")
chunk = 0
async with aiofiles.open('./static/uploads/%s' % filename,'ab') as target_file:
while True:
try:
source_file = open('./static/uploads/%s_%s' % (filename,chunk), 'rb')
await target_file.write(source_file.read())
source_file.close()
except Exception as e:
print(str(e))
break
chunk = chunk + 1
self.finish({"msg":"ok","errcode":0})
这里通过文件名进行寻址,随后遍历合并,注意句柄写入模式为增量字节码写入,否则会逐层将分片文件覆盖,同时也兼具了断点续写的功能。有些逻辑会将分片个数传入后端,让后端判断分片合并个数,其实并不需要,因为如果寻址失败,会自动抛出异常并且跳出循环,从而节约了一个参数的带宽占用。
在真实的超大文件传输场景中,由于网络或者其他因素,很可能导致分片任务中断,此时就需要通过降级快速响应,返回托底数据,避免用户的长时间等待,这里我们使用基于Tornado的Apscheduler库来调度分片任务:
pip install apscheduler
随后编写job.py轮询服务文件:
from datetime import datetime
from tornado.ioloop import IOLoop, PeriodicCallback
from tornado.web import RequestHandler, Application
from apscheduler.schedulers.tornado import TornadoScheduler
scheduler = None
job_ids = []
# 初始化
def init_scheduler():
global scheduler
scheduler = TornadoScheduler()
scheduler.start()
print('[Scheduler Init]APScheduler has been started')
# 要执行的定时任务在这里
def task1(options):
print('{} [APScheduler][Task]-{}'.format(datetime.now().strftime('%Y-%m-%d %H:%M:%S.%f'), options))
class MainHandler(RequestHandler):
def get(self):
self.write('<a href="/scheduler?job_id=1&action=add">add job</a><br><a href="/scheduler?job_id=1&action=remove">remove job</a>')
class SchedulerHandler(RequestHandler):
def get(self):
global job_ids
job_id = self.get_query_argument('job_id', None)
action = self.get_query_argument('action', None)
if job_id:
# add
if 'add' == action:
if job_id not in job_ids:
job_ids.append(job_id)
scheduler.add_job(task1, 'interval', seconds=3, id=job_id, args=(job_id,))
self.write('[TASK ADDED] - {}'.format(job_id))
else:
self.write('[TASK EXISTS] - {}'.format(job_id))
# remove
elif 'remove' == action:
if job_id in job_ids:
scheduler.remove_job(job_id)
job_ids.remove(job_id)
self.write('[TASK REMOVED] - {}'.format(job_id))
else:
self.write('[TASK NOT FOUND] - {}'.format(job_id))
else:
self.write('[INVALID PARAMS] INVALID job_id or action')
if __name__ == "__main__":
routes = [
(r"/", MainHandler),
(r"/scheduler/?", SchedulerHandler),
]
init_scheduler()
app = Application(routes, debug=True)
app.listen(8888)
IOLoop.current().start()
每一次分片接口被调用后,就建立定时任务对分片文件进行监测,如果分片成功就删除分片文件,同时删除任务,否则就启用降级预案。
分治法对超大文件进行分片切割,同时并发异步发送,可以提高传输效率,降低传输时间,和之前的一篇:聚是一团火散作满天星,前端Vue.js+elementUI结合后端FastAPI实现大文件分片上传,逻辑上有异曲同工之妙,但手法上却略有不同,确是颇有相互借镜之处,最后代码开源于Github:https://github.com/zcxey2911/Tornado6_Vuejs3_Edu,与众亲同飨。
Recommend
About Joyk
Aggregate valuable and interesting links.
Joyk means Joy of geeK