

Create YOLOv3 using PyTorch from scratch (Part-3)
source link: https://numbersmithy.com/create-yolov3-using-pytorch-from-scratch-part-3/
Go to the source link to view the article. You can view the picture content, updated content and better typesetting reading experience. If the link is broken, please click the button below to view the snapshot at that time.
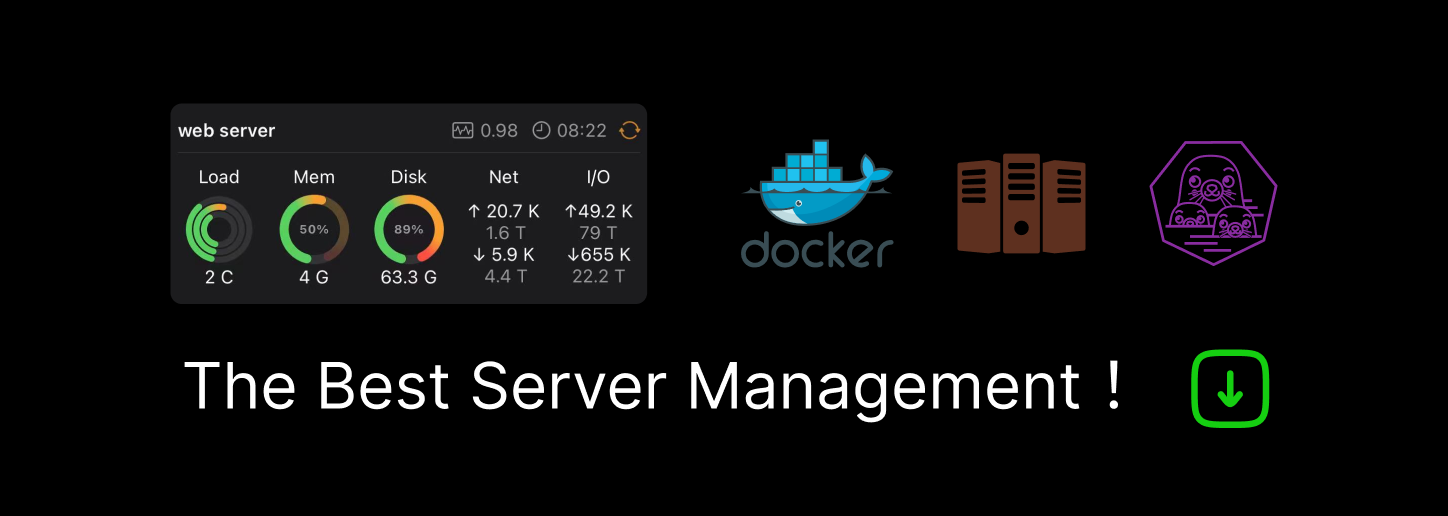
3 Write the weight-loading method
We are going to equip our Darknet53
model with a load_weights()
method that reads and loads the downloaded weights into the model
layers. So if you haven’t built the Darknet53
model, please go to
Part-2 of the series and get the model ready.
Below is the load_weights()
method. Put it inside our Darknet53
class:
def load_weights(self, weight_file): '''Load pretrained weights''' def getSlice(w, cur, length): return torch.from_numpy(w[cur:cur+length]), cur+length def loadW(data, target): data = data.view_as(target) with torch.no_grad(): target.copy_(data) return with open(weight_file, 'rb') as fin: # the 1st 5 values are header info # 1. major version number # 2. minor version number # 3. subversion number # 4,5. images seen by the network during training self.header_info = np.fromfile(fin, dtype=np.int32, count=5) self.seen = self.header_info[3] weights = np.fromfile(fin, dtype=np.float32) ptr = 0 for layer in self.layers.values(): if not isinstance(layer, ConvBNReLU): continue conv = layer.layers[0] if layer.bn: bn = layer.layers[1] # get the number of weights of bn layer num = bn.bias.numel() # load the weights bn_bias, ptr = getSlice(weights, ptr, num) bn_weight, ptr = getSlice(weights, ptr, num) bn_running_mean, ptr = getSlice(weights, ptr, num) bn_running_var, ptr = getSlice(weights, ptr, num) # cast the loaded weights into dims of module weights loadW(bn_bias, bn.bias) loadW(bn_weight, bn.weight) loadW(bn_running_mean, bn.running_mean) loadW(bn_running_var, bn.running_var) else: # number of conv biases num = conv.bias.numel() # load the weights conv_bias, ptr = getSlice(weights, ptr, num) loadW(conv_bias, conv.bias) # conv weights num = conv.weight.numel() conv_weight, ptr = getSlice(weights, ptr, num) loadW(conv_weight, conv.weight) assert len(weights) == ptr, 'Not all weight values loaded.' return
Some more explanations.
The pre-trained weights are saved in binary format, so we open it in
binary-reading (rb
) mode:
with open(weight_file, 'rb') as fin:
The numpy.fromfile()
function is used to read from the opened file
object.
The 1st 5 numbers are header information.
Starting from the 6th number are the model weights. We read them all
into a weights
array:
self.header_info = np.fromfile(fin, dtype=np.int32, count=5) weights = np.fromfile(fin, dtype=np.float32)
It is important to keep track of how many numbers we read from this big array. The exact number of weights needs to be read and fed into the correct places of the model layers, such that the trained weights can function as they were trained to do.
To help getting slices of numbers from the array, we create a
getSlice()
helper function that cuts a slice starting from a pointed
location cur
, with
length length
. The function then shifts the pointer cur
by length
so
that it points to the next number to be read:
def getSlice(w, cur, length): return torch.from_numpy(w[cur:cur+length]), cur+length
Then we initialize the pointer ptr
to point to the beginning of
the array weights
, and enter into an iteration through the model
layers:
ptr = 0 for layer in self.layers.values(): if not isinstance(layer, ConvBNReLU): continue conv = layer.layers[0]
Only convolutional layers have trainable weights, so we skip all other types of layers.
Recall that if the convolutional layer is followed by a batch
normalization, then the Conv2d
module has no bias terms.
So we query the layer’s .bn
attribute to see if it is case. If so,
we call bn.bias.numel()
to get the number weights in the
BatchNorm2d
module, slice out the weight numbers, and call a
loadW()
helper function to feed the weights into the module:
if layer.bn: bn = layer.layers[1] # get the number of weights of bn layer num = bn.bias.numel() # load the weights bn_bias, ptr = getSlice(weights, ptr, num) bn_weight, ptr = getSlice(weights, ptr, num) bn_running_mean, ptr = getSlice(weights, ptr, num) bn_running_var, ptr = getSlice(weights, ptr, num) # cast the loaded weights into dims of module weights loadW(bn_bias, bn.bias) loadW(bn_weight, bn.weight) loadW(bn_running_mean, bn.running_mean) loadW(bn_running_var, bn.running_var)
If the convolutional layer has no batch normalization, then load a bias term:
else: # number of conv biases num = conv.bias.numel() # load the weights conv_bias, ptr = getSlice(weights, ptr, num) loadW(conv_bias, conv.bias)
Lastly, we slice out the weights for the convolutional kernel and feed
that into the Conv2d
module:
# conv weights num = conv.weight.numel() conv_weight, ptr = getSlice(weights, ptr, num) loadW(conv_weight, conv.weight)
Once the iteration through the network layers is finished, we should have a properly functioning YOLOv3. Let’s test that out.
Recommend
About Joyk
Aggregate valuable and interesting links.
Joyk means Joy of geeK