

图论(graph)相关算法总结
source link: https://blog.51cto.com/zc123/5333290
Go to the source link to view the article. You can view the picture content, updated content and better typesetting reading experience. If the link is broken, please click the button below to view the snapshot at that time.
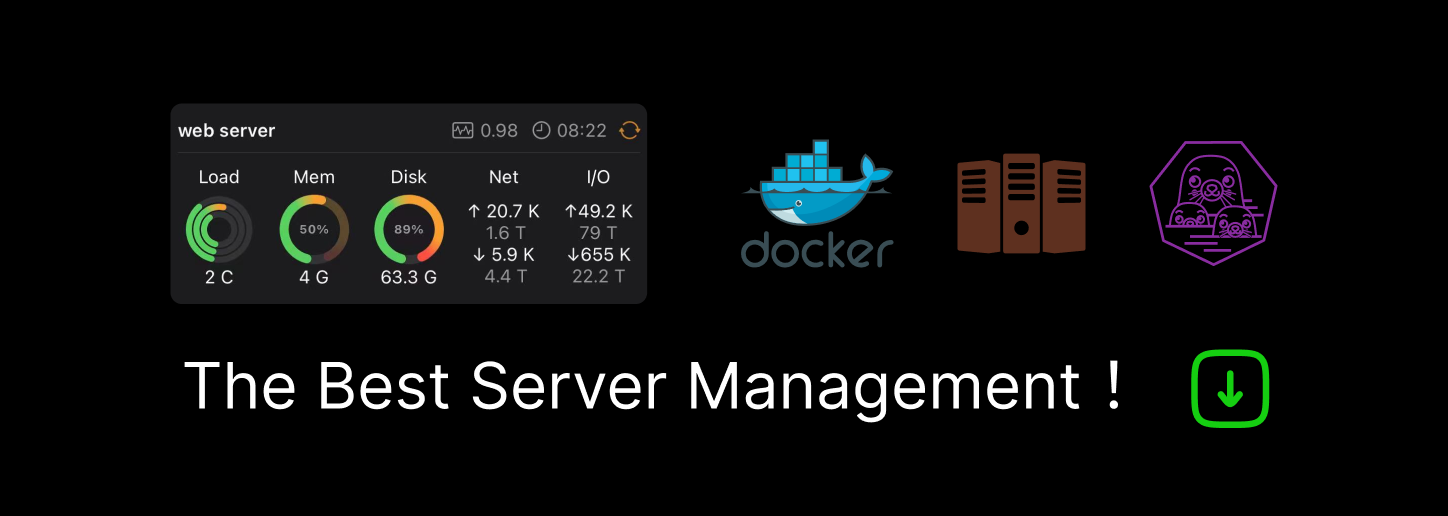
2 无向图
2.1 术语表
2.2 表示无向图的数据类型
2.3 图的几种表示方法
2.4 邻接表的数据结构
2.5 深度优先搜索(DFS)
2.6 广度优先搜索(BFS)
2.7 连通分量
2.8 无环图的判断
2.9 二分图的判断
3 有向图
3.1 有向图术语
3.2 有向图的数据类型
3.3 标记-清除的垃圾收集
3.4 寻找有向环
3.5 有向图基于DFS搜索的顶点排序
3.6 拓扑排序
3.7 有向图的强连通性
3.8 Kosaraju算法求强连通分量
开始本次的内容:
1 图的典型应用

特殊的图:我们的定义允许出现两种简单而特殊的情况
- 自环 即一条连接一个顶点和其自身的边
- 连接同一对顶点的两条边称为平行边
数学家常常将含有平行边的图称为多重图,而将没有平行边或自环的图称简单图。一般来说,允许出现自环和平行边。
2.1 术语表
当两个顶点通过一条边相连时,我们称这两个顶点是相邻的,并称这条边依附于这两个顶点。某个顶点的度数即为依附于它的边的总数。子图
是由一幅图的所有边的一个子集(以及它们所依附的所有顶点)组成的图。
环是一条至少含有一条边且起点和终点相同的路径。简单环是一条
(除了起点和终点必须相同之外)不含有重复顶点和边的环。路径或者边的长度为其中所包含的边数。
定义:如果从任意一个顶点都存在一条路径到达另一个任意顶点,我们称这幅图是连通图。
一幅非连通的图由若干连通的部分组成,它们都是其极大连通子图
树是一幅无环连通图。互不相连的树组成的集合称为森林。
连通图的生成树是它的一幅子图,它含有图中的所有顶点且是一颗树。
图的生成树森林是它的所有连通子图的生成树的集合。
2.2 表示无向图的数据类型
无向图的API
---------------------------------------------------------------------------------
Graph(int V) 创建一个含有V个顶点但不含有边的图
Graph(In in) 从标准输入流in中读入一幅图
int V() 顶点数
int E() 边数
void addEdge(int v, int w) 向图中添加一条边
Iterable adj(int v) 和v相邻的所有顶点
String toString() 对象的字符串表示
public static int degree(Graph G, int v) {
int degree = 0;
for (int w : G.adj(v)) degree++;
return degree;
}
//计算所有顶点的最大度数
public static int maxDegree(Graph G) {
int max = 0;
for (int v = 0; v < G.V(); v++) {
if (degree(G, v) > max)
max = degree(G, v);
}
return max;
}
//计算所有定点的平均度数
public static double avgDegree(Graph G) {
return 2.0 * G.E() / G.V();
}
//计算自环的个数
public static int numberOfSelfLoops(Graph G) {
int count = 0;
for (int v = 0; v < G.V(); v++) {
for (int w : G.adj(v)) {
if (w == v) count++;
}
}
//每一条边都被标记过两次
return count / 2;
}
2.3 图的几种表示方法
图处理实现API必须满足以下两个要求:
- 它必须为可能在应用中碰到的各种类型的图预留出足够的空间
- Graph的实例方法的实现一定要快——它们是开发处理图的各种用例的基础
- 邻接矩阵:我们可以使用一个V乘V的布尔矩阵来表示,但对于大图(上百万顶点)来说,VxV个布尔值所需的空间是不能满足的。
- 边的数组:我们可以使用一个Edge类,它含有两个int实例变量。这种方法简单却不满足第二个条件——要实现adj()需要检查图中的所有边
- 邻接表数组:以顶点为索引的列表数组,其中的每个元素都是和该顶点相邻的顶点列表。
2.4 邻接表的数据结构
我们使用这个数组就是为了快速访问给定顶点的邻接顶点列表。

2.5 深度优先搜索(DFS)
深度优先搜索适合解决单点路径问题
private boolean[] marked;
private int count;
public DepthFirstSearch(Graph G, int s) {
marked = new boolean[G.V()];
dfs(G, s);
}
private void dfs(Graph G, int v) {
//System.out.println("结" + v + "已被标记");
marked[v] = true;
count++;
for (int w : G.adj(v)) {
if (!marked[w]) {
dfs(G, w);
}
}
}
public int getCount() {
return count;
}
}
从结点0开始遍历上图,遍历顺序为[0,1,2,3,4,5]
private boolean[] marked;
private int[] edgeTo; //从起点到一个顶点的已知路径上的最后一个顶点(父链接数组)
private final int s; //起点
public DepthFirstPaths(Graph G, int s) {
marked = new boolean[G.V()];
edgeTo = new int[G.V()];
this.s = s;
dfs(G, s);
}
private void dfs(Graph G, int v) {
marked[v] = true;
for (int w : G.adj(v)) {
if (!marked[w]) {
edgeTo[w] = v;
dfs(G, w);
}
}
}
public boolean hasPathTo(int v) {
return marked[v];
}
public Stack pathTo(int v) {
if (!hasPathTo(v)) return null;
Stack path = new Stack();
for (int x = v; x != s; x = edgeTo[x]) {
path.push(x);
}
path.push(s);
return path;
}
}
2.6 广度优先搜索(BFS)
广度优先搜索适合解决单点最短路径问题
private boolean[] marked;
private int[] edgeTo; //父链接数组
private final int s; //起点
public BreadthFirstPaths(Graph G, int s) {
marked = new boolean[G.V()];
edgeTo = new int[G.V()];
this.s = s;
bfs(G, s);
}
private void bfs(Graph G, int s) {
Queue queue = new ArrayDeque<>();
marked[s] = true; //标记起点
queue.offer(s);
while (!queue.isEmpty()) {
int v = queue.poll();
for (int w : G.adj(v)) {
if (!marked[w]) {
edgeTo[w] = v;
marked[w] = true;
queue.offer(w);
}
}
}
}
public boolean hasPathTo(int v) {
return marked[v];
}
public Stack pathTo(int v) {
if (!hasPathTo(v)) return null;
Stack path = new Stack();
for (int x = v; x != s; x = edgeTo[x]) {
path.push(x);
}
path.push(s);
return path;
}
}
命题B(续):广度优先搜索所需的时间在最坏情况下和V+E成正比
public class GraphTest {
public static void main(String[] args) {
int V = 6;
int[][] edges = {{0, 1}, {0, 2}, {0, 5}, {1, 2}, {2, 3}, {2, 4}, {3, 4}, {3, 5}};
Graph g = new Graph(V, edges);
System.out.println("顶点数为:" + g.V());
System.out.println("边数为:" + g.E());
HashSet set = (HashSet) g.adj(2);
System.out.println("顶点2包含的边有:");
for (Integer v : set) {
System.out.println(v);
}
DepthFirstSearch df = new DepthFirstSearch(g, 0);
System.out.println("结点数为:" + df.getCount());
System.out.println("\n深度优先遍历:");
DepthFirstPaths dps = new DepthFirstPaths(g, 3);
Stack stackd = dps.pathTo(1);
while (!stackd.isEmpty()) {
System.out.println("-> " + stackd.pop());
}
System.out.println("\n广度优先遍历:");
BreadthFirstPaths bps = new BreadthFirstPaths(g, 0);
Stack stackb = bps.pathTo(5);
while (!stackb.isEmpty()) {
System.out.println("-> " + stackb.pop());
}
}
}
2.7 连通分量
连通是一种等价关系,它能够将所有顶点切分为等价类(连通分量);
连通分量的API
------------------------------------------------------------------------------------
CC(Graph G) 预处理构造函数
boolean connected(int v, int w) v和w连通吗
int count() 连通分量数
int id(int v) v所在的连通分量标识符(0~count-1)
使用深度优先搜索找出图中的所有连通分量
private boolean[] marked;
private int[] id;
private int count;
public CC(Graph G) {
marked = new boolean[G.V()];
id = new int[G.V()];
for (int s = 0; s < G.V(); s++) {
if (!marked[s]) {
dfs(G, s);
count++;
}
}
}
private void dfs(Graph G, int v) {
marked[v] = true;
id[v] = count;
for (int w : G.adj(v)) {
if (!marked[w]) {
dfs(G, w);
}
}
}
public boolean connected(int v, int w) {
return id[v] == id[w];
}
public int id(int v) {
return id[v];
}
public int count() {
return count;
}
}
2.8 无环图的判断
private boolean[] marked;
private boolean hasCycle;
public Cycle(Graph G) {
marked = new boolean[G.V()];
for (int s = 0; s < G.V(); s++) {
if (!marked[s]) {
dfs(G, s, s);
}
}
}
private void dfs(Graph G, int v, int u) {
marked[v] = true;
for (int w : G.adj(v)) {
if (!marked[w]) {
dfs(G, w, v);
} else if (w != u) {
//若当前结点w已被标记且不等于其最初遍历父链接顶点u,表示有环
hasCycle = true;
}
}
}
public boolean hasCycle() {
return hasCycle;
}
}
2.9 二分图的判断
二分图也称为二部图。
双色问题:能够用两种颜色将图的所有顶点着色,使得任意一条边的两个端点的颜色都不相同。
无向图G为二分图的充要条件是:
- G中至少包含两个顶点
- G中所有的回路长度都必须是偶数
private boolean[] marked;
private boolean[] color;
private boolean isTwoColorable = true;
public TwoColor(Graph G) {
marked = new boolean[G.V()];
color = new boolean[G.V()];
for (int s = 0; s if (!marked[s]) {
dfs(G, s);
}
}
}
private void dfs(Graph G, int v) {
marked[v] = true;
for (int w : G.adj(v)) {
if (!marked[w]) {
color[w] = !color[v];
dfs(G, w);
} else if (color[w] == color[v]) {
isTwoColorable = false;
return;
}
}
}
public boolean isBipartite() {
return isTwoColorable;
}
}
();>

public class GraphTest {
public static void main(String[] args)
int V = 7;
int[][] edges = {{0, 1}, {0, 2}, {1, 3}, {2, 6}, {3, 5}, {3, 5}, {4, 6}, {5, 6}};
Graph g = new Graph(V, edges);
System.out.println("\n判断G中是否含有环:");
Cycle cy = new Cycle(g);
System.out.println(cy.hasCycle());
System.out.println("\n判断G是否是二部图:");
TwoColor tc = new TwoColor(g);
System.out.println(tc.isBipartite());
}
}

3.1 有向图术语
每条有方向的边都连接着有序的一对顶点。
定义:在一幅有向图中,有向路径由一系列顶点组成,对于其中的每个顶点都存在一条有向边从它指向序列中的下一个顶点。
有向环为一条至少含有一条边且起点和终点相同的有向路径。简单有向环是一条(除了起点和终点必须相同之外)
不含有重复的顶点和边的环。路径或者环的长度即为其中所包含的边数。
3.2 有向图的数据类型
有向图的API
-----------------------------------------------------------------------------------
Digraph(int V) 创建一幅含有V个顶点但没有边的有向图
Digraph(In in) 从输入流in中读入一幅有向图
int V() 顶点总数
int E() 边的总数
void addEdge(int v, int w) 添加一条边v->w
Iterable adj 由v指出的边所连接的所有顶点
Digraph reverse() 该图的反向图
String toString() 对象的字符串表示
Digraph数据类型
private final int V;
private int E;
private List[] adj;
public Digraph(int V) {
this.V = V;
this.E = 0;
adj = new ArrayList[V];
for (int v = 0; v < V; v++) {
adj[v] = new ArrayList();
}
}
public Digraph(int V, int[][] edges) {
this.V = V;
this.E = 0;
adj = new ArrayList[V];
for (int v = 0; v < V; v++) {
adj[v] = new ArrayList();
}
for (int[] edge : edges) {
addEdge(edge[0], edge[1]);
}
}
public void addEdge(int w, int v) {
adj[w].add(v);
E++;
}
public int V() {
return V;
}
public int E() {
return E;
}
public List adj(int v) {
return adj[v];
}
public Digraph reverse() {
Digraph R = new Digraph(V);
for (int v = 0; v < V; v++) {
for (int w : adj(v)) {
R.addEdge(w, v);
}
}
return R;
}
public String toString() {
String str = "";
for (int v = 0; v < V; v++) {
str += v + " : ";
for (int w : adj(v)) {
str += " -> " + w;
}
str += "\n";
}
return str;
}
}
3.3 标记-清除的垃圾收集
一个顶点表示一个对象,一条边则表示一个对象对另一个对象的引用。
这个模型很好地表现了运行中的Java程序的内存使用状况。在程序执行的任何时候都有某些对象是可以被直接访问的,
而不能通过这些对象访问到的所有对象都应该被回收以便释放内存。
标记-清除的垃圾回收策略会为每个对象保留一个位做垃圾收集之用。
它会周期性地运行一个类似于DirectedDFS的有向图可达性算法来标记所有可以被访问到的对象,然后清理所有对象,
回收没有被标记的对象,以腾出内存供新的对象使用。
3.4 寻找有向环
private boolean[] marked;
private int[] edgeTo;
private Stack cycle; //有向环中的所有顶点
private boolean[] onStack; //递归调用的栈上所有顶点
public DirectedCycle (Digraph G) {
onStack = new boolean[G.V()];
edgeTo = new int[G.V()];
marked = new boolean[G.V()];
for (int v = 0; v < G.V(); v++) {
if (!marked[v]) {
dfs(G, v);
}
}
}
private void dfs(Digraph G, int v) {
onStack[v] = true;
marked[v] = true;
for (int w : G.adj(v)) {
if (this.hasCycle()) return;
else if (!marked[w]) {
edgeTo[v] = w;
dfs(G, w);
} else if (onStack[w]){
cycle = new Stack();
for (int x = v; x != w; x = edgeTo[x]) {
cycle.push(x);
}
cycle.push(w);
cycle.push(v);
}
}
onStack[v] = false;
}
public boolean hasCycle() {
return cycle != null;
}
public Stack cycle() {
return cycle;
}
}

public static void main(String[] args) {
int V = 4;
int[][] edges = {{0, 1}, {1, 3}, {3, 2}, {2, 1}};
Digraph g = new Digraph(V, edges);
DirectedCycle dc = new DirectedCycle(g);
if (dc.hasCycle()) {
Stack cycle = dc.cycle();
String cycleV = "";
while (!cycle.isEmpty()) {
cycleV += "->" + String.valueOf(cycle.pop());
}
System.out.println("环为 : " + cycleV);
}
}
}
3.5 有向图基于DFS搜索的顶点排序
- 前序:在递归调用之前将顶点加入队列
- 后序:在递归调用之后将顶点加入队列
- 逆后序:在递归调用之后将顶点压入栈

private boolean[] marked;
private Queue pre; //所有顶点的前序排列
private Queue post; //所有顶点的后序排列
private Stack reversepost; //所有顶点的逆后续排列
public DepthFirstOrder(Digraph G) {
pre = new ArrayDeque<>();
post = new ArrayDeque<>();
reversepost = new Stack<>();
marked = new boolean[G.V()];
for (int v = 0; v < G.V(); v++) {
if (!marked[v]) {
dfs(G, v);
}
}
}
private void dfs(Digraph G, int v) {
pre.offer(v);
marked[v] = true;
for (int w : G.adj(v)) {
if (!marked[w]) {
dfs(G, w);
}
}
post.offer(v);
reversepost.push(v);
}
public Iterable pre() {
return pre;
}
public Iterable post() {
return post;
}
//注意:此处不用迭代器的原因是迭代器对栈的遍历是从栈底开始的
public Stack reversePost() {
return reversepost;
}
}
后序排列为:1, 4, 5, 10, 12, 11, 9, 6, 0, 3, 2, 7, 8
逆后序排列为:8, 7, 2, 3, 0, 6, 9, 11, 12, 10, 5, 4, 1
public static void main(String[] args) {
int V = 13;
int[][] edges = {{0, 1}, {0, 5}, {0, 6}, {2, 0}, {2, 3}, {3, 5}, {5, 4},
{6, 4}, {6, 9}, {7, 6}, {8, 7}, {9, 10}, {9, 11}, {9, 12}, {11, 12}};
Digraph g = new Digraph(V, edges);
DepthFirstOrder df = new DepthFirstOrder(g);
Iterable pre = df.pre();
String spre = "";
Iterator ipre = pre.iterator();
while (ipre.hasNext()) {
spre += ipre.next() + ", ";
}
System.out.println("前序排列为:" + spre);
//同理可得后序排列,逆后续排列
Iterable post = df.post();
String spost = "";
Iterator ipost = post.iterator();
while (ipost.hasNext()) {
spost += ipost.next() + ", ";
}
System.out.println("后序排列为:" + spost);
Stack reversePost = df.reversePost();
String sreversePost = "";
while (!reversePost.isEmpty()) {
sreversePost += reversePost.pop() + ", ";
}
System.out.println("逆后序排列为:" + sreversePost);
}
}
3.6 拓扑排序
命题: 一幅有向图的拓扑排序顺序即为所有顶点的逆后续排列
private Stack order; //顶点的拓扑排序
public Topological(Digraph G) {
DirectedCycle cycleFinder = new DirectedCycle(G);
if (!cycleFinder.hasCycle()) {
DepthFirstOrder dfs = new DepthFirstOrder(G);
order = dfs.reversePost();
}
}
public Stack order() {
return order;
}
//是有向无环图吗
public boolean isDAG() {
return order != null;
}
}
private Queue order;
private int[] degrees;
BaseQueueTopological(Digraph G) {
order = new ArrayDeque<>();
DirectedCycle cycleFinder = new DirectedCycle(G);
if (!cycleFinder.hasCycle()) {
degrees = new int[G.V()];
for (int v = 0; v < G.V(); v++) {
for (int w : G.adj(v)) {
degrees[w]++;
}
}
ordered(G);
}
}
private void ordered(Digraph G) {
Queue queue = new ArrayDeque<>();
for (int v = 0; v < G.V(); v++) {
if (degrees[v] == 0) {
queue.offer(v);
}
}
while (!queue.isEmpty()) {
int v = queue.poll();
order.offer(v);
for (int w : G.adj(v)) {
degrees[w]--;
if (degrees[w] == 0) {
queue.offer(w);
}
}
}
}
public Iterable order() {
return order;
}
//是有向无环图吗
public boolean isDAG() {
return !order.isEmpty();
}
}
public static void main(String[] args) {
int V = 13;
int[][] edges = {{0, 1}, {0, 5}, {0, 6}, {2, 0}, {2, 3}, {3, 5}, {5, 4},
{6, 4}, {6, 9}, {7, 6}, {8, 7}, {9, 10}, {9, 11}, {9, 12}, {11, 12}};
Digraph g = new Digraph(V, edges);
BaseQueueTopological bt = new BaseQueueTopological(g);
if (!bt.isDAG()) {
System.out.println("有环");
} else {
Iterator order = bt.order().iterator();
String so = "";
while (order.hasNext()) {
so += order.next() + ", ";
}
System.out.println("拓扑排序为: " + so);
}
}
}
3.7 有向图的强连通性
也就是说,也就是说,既存在一条从v到w的有向路径,也存在一条从w到v的有向路径。
如果一幅有向图中的任意两个顶点都是强连通的,则称这幅有向图也是强连通的。
和无向图中的连通性一样,有向图中的强连通性也是一种顶点之间的等价关系,因为它有着以下性质。
3.8 Kosaraju算法求强连通分量
kosaraju(科萨拉朱)算法的步骤:
- 在给定的一幅有向图G中,使用DepthFirstOrder来计算它的反图G的逆后续排列。
- 在G中按照得到的逆后续排列进行标准的深度优先搜索来访问未标记结点,其每一次调用递归所标记的顶点都在同一个强连通分量中
算法原理:
- 反图与原图的强连通分量相同
- 若原图能从分量1走到分量2,则反图不能从分量1走到分量2
private boolean[] marked;
private int[] id; //强连通分量的标识符
private int count; //强连通分量的数量
public KosarajuSCC(Digraph G) {
marked = new boolean[G.V()];
id = new int[G.V()];
DepthFirstOrder order = new DepthFirstOrder(G.reverse());
Stack reversePost = order.reversePost();
while (!reversePost.isEmpty()) {
int w = reversePost.pop();
if (!marked[w]) {
dfs(G, w);
count++;
}
}
}
private void dfs(Digraph G, int v) {
marked[v] = true;
id[v] = count;
for (int w : G.adj(v))
if (!marked[w])
dfs(G, w);
}
public boolean stronglyConnected(int v, int w) {
return id[v] == id[w];
}
public int id(int v) {
return id[v];
}
public int count() {
return count;
}
}

public static void main(String[] args) {
int V = 13;
int[][] edges = {{0, 1}, {0, 5}, {2, 0}, {2, 3}, {3, 2}, {3, 5}, {4, 2},
{4, 3}, {5, 4}, {6, 0}, {6, 4}, {6, 9}, {7, 6}, {7, 8}, {8, 7},
{8, 9}, {9, 10}, {9, 11}, {10, 12}, {11, 12}, {12, 9}};
Digraph g = new Digraph(V, edges);
KosarajuSCC ko = new KosarajuSCC(g);
System.out.println("共有几个连通分量:" + ko.count());
for (int i = 0; i < ko.count(); i++) {
System.out.print("第" + (i + 1) + "个连通分量:");
for (int v = 0; v < g.V(); v++) {
if (ko.id(v) == i) {
System.out.print(v + ", ");
}
}
System.out.println("");
}
}
}
第1个连通分量:9, 10, 11, 12,
第2个连通分量:1,
第3个连通分量:0, 2, 3, 4, 5,
第4个连通分量:6,
第5个连通分量:7, 8,
Recommend
About Joyk
Aggregate valuable and interesting links.
Joyk means Joy of geeK