

AI Regulations are Coming. Are you Prepared?
source link: https://jaxenter.com/ai-regulations-177423.html
Go to the source link to view the article. You can view the picture content, updated content and better typesetting reading experience. If the link is broken, please click the button below to view the snapshot at that time.
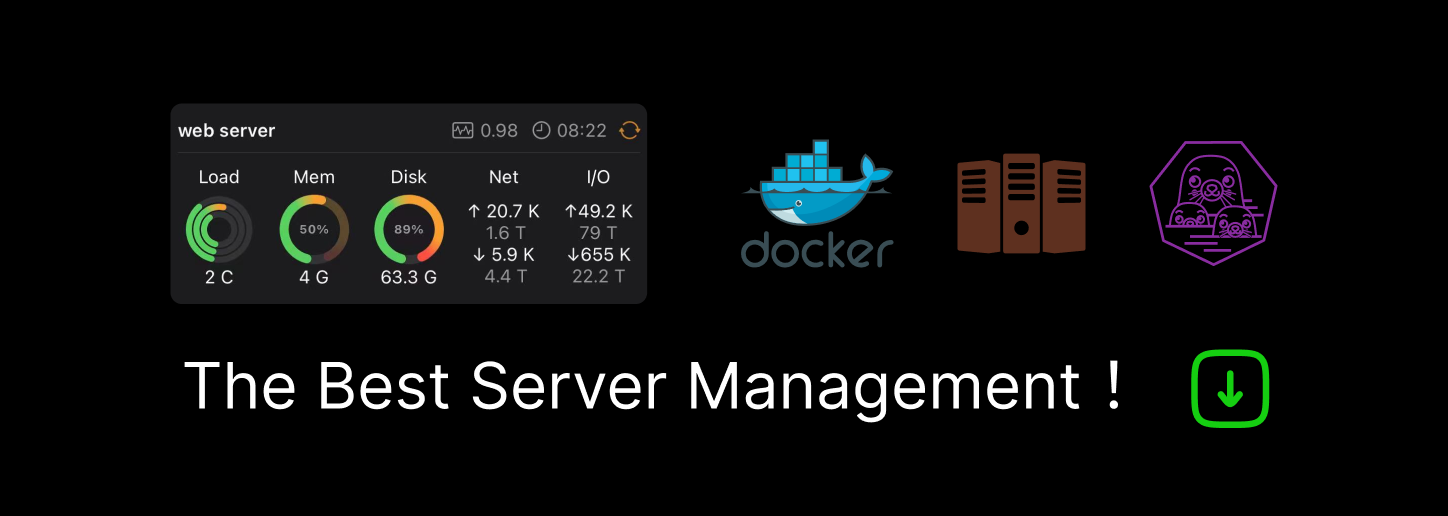
AI Regulations are Coming. Are you Prepared?

Focusing on bias detection and Artificial Intelligence (AI) explainability can help organizations be prepared with the ability to prove the fairness and accuracy of their algorithms. This article discusses three best practices for bias detection and AI explainability for your organizations.
Artificial Intelligence (AI) has the potential to do great good in the world. Some extraordinary AI use cases include reducing overfishing, detecting cancer and identifying an outbreak of COVID-19 while it was still an unknown illness.
Despite AI’s profound capabilities, it has the potential to run awry when not properly monitored and regulated. Amazon famously ditched its AI recruiting tool when it realized it was riddled with bias that overlooked female job applicants. Racial discrimination through AI-run facial recognition software has also been widely reported.
Federal and state governments are making strides to ensure Americans aren’t negatively impacted by AI algorithms that contain harmful bias. Recently, Oregon Democratic Sen. Ron Wyden introduced the Algorithmic Accountability Act of 2022. The proposed legislation would mandate AI accountability and transparency in decision-making surrounding key areas like loans, education, and healthcare.
Legislation like this is crucial for guiding future innovation both in enterprise and consumer-facing technologies. However, many obstacles stand in the way towards regulation. Some individuals see regulations as needless red tape that would hamper innovation and stifle growth. In reality, regulations should be seen as a set of rules in which AI can flourish and enhance the work of researchers and organizations while protecting citizens.
Regardless of the current obstacles, regulations are likely inevitable at some point and businesses must prepare now to ensure their algorithms are free from harmful bias. To achieve this, the automated decisions and predictions made by these algorithms must be transparent and explainable. Companies will need new processes and tools to comply with the AI regulations that are on the horizon, including system audits, documentation, enhanced data management, and AI monitoring. Focusing on bias detection and AI explainability can help organizations be prepared with the ability to prove the fairness and accuracy of their algorithms.
Below are three best practices for bias detection and AI explainability:
1. Measure population shifts over time
Measuring population shifts enables data science teams to measure the potential for sample selection bias. To detect bias, data scientists examine the outputs from an algorithm to look for anomalous results. Comparing outcomes across groups can be an excellent first step. This practice can even be done through simulations.
Measuring population shifts can show unequal outcomes; however, unequal does not necessarily mean unfair. To account for unequal outcomes, data scientists should examine the equality of error rates and whether one subset of people has more errors than another.
2. Don’t remove protected characteristics from training data before training
Modern automated decisions and machine learning models contain increasingly correlated data elements. Removing certain subsets of data increases risk by reducing transparency and visibility.
In fact, simply removing a protected characteristic that is highly correlated to other data points severely hampers your ability to see just how harmfully biased your model may unintentionally be. This is called fairness through unawareness (or fairness through blindness) and it has been proven to be ineffective because it does not account for the potential correlation of remaining data to the variable excluded. Additionally, it gives no transparent comparison to determine if the remaining proxy characteristics led to harmful bias even with the obvious element removed.
For example, let’s say we want to remove gender as a characteristic from our data. However, we leave the data for marital status and dependents in the data. According to the Pew Research Center, 81% of single parents in the US are female, so the two columns we’ve left in are highly correlated with gender. Further, we’ve removed the one that could help us evaluate whether our model is biased, whether directly or indirectly.
By training models with data that includes protected characteristics, organizations can better evaluate the fairness of a model. This means your enterprise can measure and mitigate potential risks with true visibility and transparency.
3. Regularly evaluate processes and key stakeholders
Organizations use multiple algorithms running across different regions, markets, or contexts, responding to local data and environments. To ensure that all the algorithms operate appropriately and within authorized risk ranges, organizations may need to create new processes and involve new stakeholders and subject matter experts. The Chief Risk Officer may also have to broaden his or her mandate to monitor and assess the risk of autonomous AI.
Artificial intelligence is transforming every industry. From financial services to manufacturing to media and entertainment, the opportunities for machine learning and other AI innovations to drive business growth are tremendous.
However, those potential rewards bring with them potential risk. While it is impossible to completely remove all bias from algorithms, harmful bias can be mitigated when organizations deploy AI innovations that prioritize transparency and explainability. By having the ability to look deep into algorithms, organizations can capitalize on the power of AI while they protect the business, their reputations and most importantly, their customers.
Recommend
About Joyk
Aggregate valuable and interesting links.
Joyk means Joy of geeK