
4

TensorFlow官方word2vec_basic代码解析
source link: http://nathanlvzs.github.io/TensorFlow_word2vec_basic_Code_Explained.html
Go to the source link to view the article. You can view the picture content, updated content and better typesetting reading experience. If the link is broken, please click the button below to view the snapshot at that time.
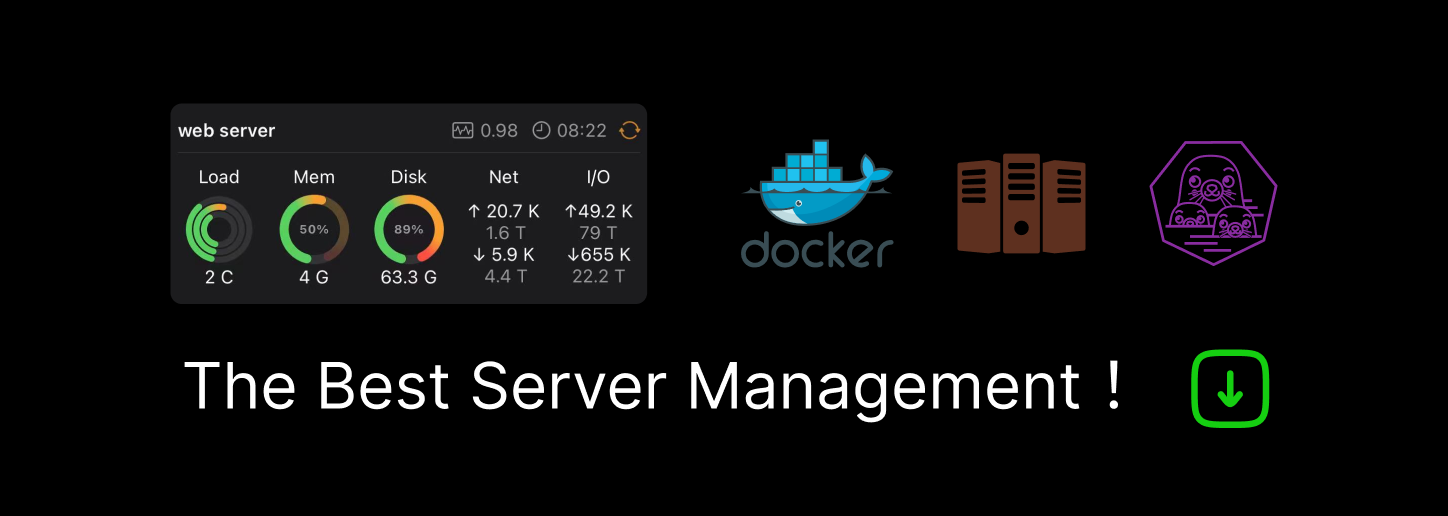
TensorFlow官方word2vec_basic代码解析
NathanLVZS | Wednesday 22 November 2017
Category: NLP
这份代码实现是基于skip-gram模型的。详细的tutorial参见链接。
# encoding=utf-8
"""Basic word2vec example."""
from __future__ import absolute_import
from __future__ import division
from __future__ import print_function
import collections
import math
import os
import random
from tempfile import gettempdir
import zipfile
import numpy as np
from six.moves import urllib
from six.moves import xrange # pylint: disable=redefined-builtin
import tensorflow as tf
# 步骤1:下载准备数据
url = 'http://mattmahoney.net/dc/'
# pylint: disable=redefined-outer-name
def maybe_download(filename, expected_bytes):
"""Download a file if not present, and make sure it's the right size."""
# 在临时目录下寻找数据集文件,如果没有则联网下载,并对文件做大小验证
local_filename = os.path.join(gettempdir(), filename)
# 改:换成当前目录
local_filename = filename
if not os.path.exists(local_filename):
local_filename, _ = urllib.request.urlretrieve(url + filename,
local_filename)
statinfo = os.stat(local_filename)
if statinfo.st_size == expected_bytes:
print('Found and verified', filename)
else:
print(statinfo.st_size)
raise Exception('Failed to verify ' + local_filename +
'. Can you get to it with a browser?')
return local_filename
# 从zip包中读取数据,返回字符串列表
def read_data(filename):
"""Extract the first file enclosed in a zip file as a list of words."""
# 读取数据集压缩包文件中第一个文件的内容,返回单词列表
# https://www.tensorflow.org/api_docs/python/tf/compat/as_str_any
with zipfile.ZipFile(filename) as f:
data = tf.compat.as_str(f.read(f.namelist()[0])).split()
return data
filename = maybe_download('text8.zip', 31344016)
vocabulary = read_data(filename)
# Data size 17005207
print('Data size', len(vocabulary))
# Step 2: 构建词典,将罕见字替换成UNK
# 限制词典大小为50000
vocabulary_size = 50000
def build_dataset(words, n_words):
"""Process raw inputs into a dataset."""
# 构建数据集
# 返回四个元素:编号表示的文本,词典中词及其频次结果列表,词到编号的映射字典,编号到词的映射字典
count = [['UNK', -1]] # UNK这里的内容之所以是列表,是因为后面要更改它的频次
# 统计文本数据中的词频,将出现频次在前n_words-1的字和其字频添加到count列表中
count.extend(collections.Counter(words).most_common(n_words - 1))
# 按照count列表中的顺序,给每个字编号
dictionary = dict()
for word, _ in count:
dictionary[word] = len(dictionary)
# 将文本转换成编号表示
data = list()
unk_count = 0 # 统计文本数据中出现了多少次那些要被替换成UNK的词
for word in words:
index = dictionary.get(word, 0)
if index == 0: # dictionary['UNK']
unk_count += 1
data.append(index)
count[0][1] = unk_count # 更新UNK的频次
reversed_dictionary = dict(zip(dictionary.values(), dictionary.keys()))
return data, count, dictionary, reversed_dictionary
# Filling 4 global variables:
# data - list of codes (integers from 0 to vocabulary_size-1).
# This is the original text but words are replaced by their codes
# count - map of words(strings) to count of occurrences
# dictionary - map of words(strings) to their codes(integers)
# reverse_dictionary - maps codes(integers) to words(strings)
data, count, dictionary, reverse_dictionary = build_dataset(vocabulary,
vocabulary_size)
del vocabulary # Hint to reduce memory.
print('Most common words (+UNK)', count[:5])
print('Sample data', data[:10], [reverse_dictionary[i] for i in data[:10]])
data_index = 0
# Step 3: Function to generate a training batch for the skip-gram model.
def generate_batch(batch_size, num_skips, skip_window):
# batch_size 一个batch包含多少个样本,须是num_skips的整数倍
# num_skips 一个目标词要预测多少次上下文词语,用于采样
# skip_window 目标词一边窗口的大小,比如为2的话,表示要根据目标词预测上文2个词、下文2个词
# 生成batch,
global data_index
assert batch_size % num_skips == 0
assert num_skips <= 2 * skip_window
batch = np.ndarray(shape=(batch_size), dtype=np.int32) # 每个样本的x为目标词本身
labels = np.ndarray(shape=(batch_size, 1), dtype=np.int32) # 每个样本的y为目标词窗口中的一个词
span = 2 * skip_window + 1 # [ skip_window target skip_window ]
# 定长的buffer
buffer = collections.deque(maxlen=span)
if data_index + span > len(data): # 不足一个span,将data_index复位
data_index = 0
# 将一个span的数据放入buffer中
buffer.extend(data[data_index:data_index + span])
data_index += span
# batch//num_skips 为一个batch中要包含目标词的数量
for i in range(batch_size // num_skips):
# 上下文词语的位置索引
context_words = [w for w in range(span) if w != skip_window]
# 从context_words中采样num_skips次
words_to_use = random.sample(context_words, num_skips)
for j, context_word in enumerate(words_to_use):
batch[i * num_skips + j] = buffer[skip_window]
labels[i * num_skips + j, 0] = buffer[context_word]
# 更新data_index和buffer
if data_index == len(data):
buffer[:] = data[:span]
data_index = span
else:
# 加入一项,相当于buffer窗口往右滑一下
buffer.append(data[data_index])
data_index += 1
# Backtrack a little bit to avoid skipping words in the end of a batch
data_index = (data_index + len(data) - span) % len(data)
return batch, labels
batch, labels = generate_batch(batch_size=8, num_skips=2, skip_window=1)
# 打印batch和labels中的部分内容
for i in range(8):
print(batch[i], reverse_dictionary[batch[i]],
'->', labels[i, 0], reverse_dictionary[labels[i, 0]])
# Step 4: Build and train a skip-gram model.
# 构建训练skip-gram模型
batch_size = 128
embedding_size = 128 # Dimension of the embedding vector.
skip_window = 1 # How many words to consider left and right.
num_skips = 2 # How many times to reuse an input to generate a label.
num_sampled = 64 # Number of negative examples to sample.
# 挑一些数据出来用于绘图展示
# We pick a random validation set to sample nearest neighbors. Here we limit the
# validation samples to the words that have a low numeric ID, which by
# construction are also the most frequent. These 3 variables are used only for
# displaying model accuracy, they don't affect calculation.
valid_size = 16 # Random set of words to evaluate similarity on.
valid_window = 100 # Only pick dev samples in the head of the distribution.
valid_examples = np.random.choice(valid_window, valid_size, replace=False)
graph = tf.Graph()
with graph.as_default():
# Input data.
train_inputs = tf.placeholder(tf.int32, shape=[batch_size])
train_labels = tf.placeholder(tf.int32, shape=[batch_size, 1])
valid_dataset = tf.constant(valid_examples, dtype=tf.int32)
# Ops and variables pinned to the CPU because of missing GPU implementation
with tf.device('/cpu:0'):
# embedding层
# Look up embeddings for inputs.
embeddings = tf.Variable(
tf.random_uniform([vocabulary_size, embedding_size], -1.0, 1.0))
embed = tf.nn.embedding_lookup(embeddings, train_inputs)
# Construct the variables for the NCE loss
# 为noise contrastive estimation loss构造相关参数容器
# 权重矩阵,维度 词典大小×词向量大小,正态分布初始化
nce_weights = tf.Variable(
tf.truncated_normal([vocabulary_size, embedding_size],
stddev=1.0 / math.sqrt(embedding_size)))
# 偏置向量,维度:词典大小
nce_biases = tf.Variable(tf.zeros([vocabulary_size]))
# 计算一个batch的平均NCE损失
# Compute the average NCE loss for the batch.
# tf.nce_loss automatically draws a new sample of the negative labels each
# time we evaluate the loss.
# Explanation of the meaning of NCE loss:
# http://mccormickml.com/2016/04/19/word2vec-tutorial-the-skip-gram-model/
loss = tf.reduce_mean(
tf.nn.nce_loss(weights=nce_weights,
biases=nce_biases,
labels=train_labels,
inputs=embed,
num_sampled=num_sampled, # 负采样的次数
num_classes=vocabulary_size))
# 随机梯度下降优化器,学习率1.0
# Construct the SGD optimizer using a learning rate of 1.0.
optimizer = tf.train.GradientDescentOptimizer(1.0).minimize(loss)
# Compute the cosine similarity between minibatch examples and all embeddings.
# 使embedding中每个词向量的norm都为1
norm = tf.sqrt(tf.reduce_sum(tf.square(embeddings), 1, keep_dims=True))
normalized_embeddings = embeddings / norm
valid_embeddings = tf.nn.embedding_lookup(
normalized_embeddings, valid_dataset)
# 求挑选出的词向量跟全部词向量的cosine距离
similarity = tf.matmul(
valid_embeddings, normalized_embeddings, transpose_b=True)
# Add variable initializer.
init = tf.global_variables_initializer()
# Step 5: Begin training.
# 迭代训练
num_steps = 100001
with tf.Session(graph=graph) as session:
# We must initialize all variables before we use them.
# 初始化计算图中的所有变量
init.run()
print('Initialized')
average_loss = 0
for step in xrange(num_steps):
batch_inputs, batch_labels = generate_batch(
batch_size, num_skips, skip_window)
feed_dict = {train_inputs: batch_inputs, train_labels: batch_labels}
# We perform one update step by evaluating the optimizer op (including it
# in the list of returned values for session.run()
_, loss_val = session.run([optimizer, loss], feed_dict=feed_dict)
average_loss += loss_val
# 每2000个batch处理后打印平均loss
if step % 2000 == 0:
if step > 0:
average_loss /= 2000
# The average loss is an estimate of the loss over the last 2000 batches.
print('Average loss at step ', step, ': ', average_loss)
average_loss = 0
# Note that this is expensive (~20% slowdown if computed every 500 steps)
# 每10000个batch处理后,打印看看一些词的k近邻
if step % 10000 == 0:
sim = similarity.eval()
for i in xrange(valid_size):
valid_word = reverse_dictionary[valid_examples[i]]
top_k = 8 # number of nearest neighbors
nearest = (-sim[i, :]).argsort()[1:top_k + 1]
log_str = 'Nearest to %s:' % valid_word
for k in xrange(top_k):
close_word = reverse_dictionary[nearest[k]]
log_str = '%s %s,' % (log_str, close_word)
print(log_str)
# 得到最终的词向量结果
final_embeddings = normalized_embeddings.eval()
# Step 6: Visualize the embeddings.
# 可视化
# pylint: disable=missing-docstring
# Function to draw visualization of distance between embeddings.
def plot_with_labels(low_dim_embs, labels, filename):
assert low_dim_embs.shape[0] >= len(labels), 'More labels than embeddings'
plt.figure(figsize=(18, 18)) # in inches
for i, label in enumerate(labels):
x, y = low_dim_embs[i, :]
plt.scatter(x, y)
plt.annotate(label,
xy=(x, y),
xytext=(5, 2),
textcoords='offset points',
ha='right',
va='bottom')
plt.savefig(filename)
try:
# pylint: disable=g-import-not-at-top
from sklearn.manifold import TSNE
import matplotlib.pyplot as plt
tsne = TSNE(perplexity=30, n_components=2, init='pca', n_iter=5000, method='exact')
plot_only = 500
low_dim_embs = tsne.fit_transform(final_embeddings[:plot_only, :])
labels = [reverse_dictionary[i] for i in xrange(plot_only)]
# plot_with_labels(low_dim_embs, labels, os.path.join(gettempdir(), 'tsne.png'))
# 改到当前目录
plot_with_labels(low_dim_embs, labels, 'tsne.png')
except ImportError as ex:
print('Please install sklearn, matplotlib, and scipy to show embeddings.')
print(ex)
Found and verified text8.zip
Data size 17005207
Most common words (+UNK) [['UNK', 418391], ('the', 1061396), ('of', 593677), ('and', 416629), ('one', 411764)]
Sample data [5239, 3084, 12, 6, 195, 2, 3137, 46, 59, 156] ['anarchism', 'originated', 'as', 'a', 'term', 'of', 'abuse', 'first', 'used', 'against']
3084 originated -> 5239 anarchism
3084 originated -> 12 as
12 as -> 6 a
12 as -> 3084 originated
6 a -> 12 as
6 a -> 195 term
195 term -> 6 a
195 term -> 2 of
Initialized
Average loss at step 0 : 268.651245117
Nearest to or: amide, digraphs, indication, thence, principles, nickel, infancy, grams,
Nearest to over: vern, slopes, booty, chronicled, opinions, kazantzakis, freak, donates,
Nearest to state: derivation, cryptologia, dde, acutely, cryonics, mycelium, cj, choose,
Nearest to so: spinner, renunciation, prevailing, npd, epstein, djing, generational, open,
Nearest to united: beelzebub, camacho, backslash, overthrown, announce, forbidding, cics, slater,
Nearest to they: irssi, acre, newborn, nipples, ibo, laf, dhea, warship,
Nearest to zero: romanian, quotation, popularly, tucked, alight, benedictine, encephalitis, enumerates,
Nearest to by: gogh, vicki, rsha, fitting, novas, colossians, relating, earths,
Nearest to called: alba, ryde, pregnancy, remedies, absorbing, toys, challenger, abrogated,
Nearest to his: presley, assaulting, lamellar, started, website, farsi, sombre, english,
Nearest to most: cypriot, stu, ridge, indicate, typhoon, pommel, dal, rower,
Nearest to was: influence, ihr, overdose, bit, jetliner, armchair, derleth, botanicals,
Nearest to all: beacons, pq, absence, constantin, uplifting, vla, exegetical, uncontrolled,
Nearest to use: haynes, carrot, mauro, rocket, malice, substandard, peterson, cessna,
Nearest to while: devoting, cartographic, optative, leaves, langmuir, juris, emirates, dyer,
Nearest to is: serbs, shunned, ergodic, retake, hitchcock, alters, ilo, remainders,
Average loss at step 2000 : 113.84280809
Average loss at step 4000 : 52.5495715497
Average loss at step 6000 : 33.0720247313
Average loss at step 8000 : 23.7988801641
Average loss at step 10000 : 17.8842850461
Nearest to or: and, palestine, lyon, qutb, should, in, zero, motile,
Nearest to over: profound, gulfs, aberdeen, mathilde, detect, opinions, mathbf, slopes,
Nearest to state: derivation, mctaggart, defensive, ultimately, chadic, york, diamond, destiny,
Nearest to so: mosque, archie, vs, prevailing, spinner, karl, simultaneously, marker,
Nearest to united: altenberg, zoroastrianism, vs, announce, unable, aardwolf, allies, official,
Nearest to they: passing, acre, referred, branched, ideas, but, folding, prussian,
Nearest to zero: nine, var, mctaggart, six, bckgr, omnibus, ep, one,
Nearest to by: in, and, gogh, succession, is, relating, rsha, of,
Nearest to called: pregnancy, marple, toys, remedies, sleep, challenger, during, altenberg,
Nearest to his: the, agave, aberdeen, started, glass, english, its, a,
Nearest to most: indicate, ridge, miss, hat, in, buddhists, atheist, characteristic,
Nearest to was: is, in, sigma, bckgr, march, had, rifle, and,
Nearest to all: vs, absence, benguela, cardinality, alien, agave, archie, the,
Nearest to use: carrot, agave, rocket, premature, hotly, turn, taken, phi,
Nearest to while: leaves, and, the, vs, afghanistan, khoisan, immigrants, grounds,
Nearest to is: was, and, bckgr, are, aberdeen, altenberg, cartoons, in,
Average loss at step 12000 : 13.9714334568
Average loss at step 14000 : 11.8597703229
Average loss at step 16000 : 9.92282870471
Average loss at step 18000 : 8.34154448366
Average loss at step 20000 : 8.01315830123
Nearest to or: and, zero, the, nine, agouti, six, eight, bckgr,
Nearest to over: four, gulfs, eight, profound, mathilde, slopes, detect, obtained,
Nearest to state: iota, derivation, mctaggart, regeneration, defensive, diamond, agamemnon, amazonas,
Nearest to so: mosque, archie, prevailing, vs, spinner, karl, prized, simultaneously,
Nearest to united: cranmer, zoroastrianism, altenberg, factor, overthrown, allies, forbidding, backslash,
Nearest to they: he, acre, it, passing, but, referred, ideas, sterilized,
Nearest to zero: nine, seven, eight, five, six, four, dasyprocta, two,
Nearest to by: was, in, is, gogh, and, were, agouti, as,
Nearest to called: pregnancy, toys, sleep, remedies, marple, apatosaurus, apologia, autosomal,
Nearest to his: the, its, their, agave, agouti, a, aberdeen, s,
Nearest to most: indicate, ridge, hbox, hat, miss, agouti, credit, bicycles,
Nearest to was: is, were, by, had, and, are, in, agouti,
Nearest to all: absence, vs, benguela, the, pond, cardinality, describing, madness,
Nearest to use: carrot, agave, rocket, hotly, premature, haynes, turn, taken,
Nearest to while: agouti, leaves, glycerol, catalog, button, devoting, immigrants, eternal,
Nearest to is: was, are, were, by, agouti, nine, in, but,
Average loss at step 22000 : 6.91144368327
Average loss at step 24000 : 6.86921860325
Average loss at step 26000 : 6.71094323111
Average loss at step 28000 : 6.3006513741
Average loss at step 30000 : 5.91089563227
Nearest to or: and, agouti, birkenau, six, circ, bckgr, nine, qutb,
Nearest to over: seven, profound, gulfs, four, detect, mathilde, dialect, biscuit,
Nearest to state: iota, derivation, regeneration, mctaggart, defensive, agamemnon, during, diamond,
Nearest to so: mosque, prevailing, archie, writes, vs, spinner, tuition, marker,
Nearest to united: beelzebub, cranmer, zoroastrianism, forbidding, camacho, altenberg, backslash, temperatures,
Nearest to they: he, it, aorta, acre, passing, not, thrace, prussian,
Nearest to zero: eight, seven, six, five, nine, four, three, dasyprocta,
Nearest to by: in, was, is, with, were, gogh, be, as,
Nearest to called: pregnancy, toys, sleep, at, apologia, circ, marple, during,
Nearest to his: the, their, its, agouti, agave, s, baumgarten, aberdeen,
Nearest to most: indicate, ridge, hbox, cypriot, credit, miss, ige, hottest,
Nearest to was: is, were, by, had, birkenau, sensitive, are, has,
Nearest to all: absence, vs, some, cardinality, describing, agave, mishnayot, madness,
Nearest to use: carrot, agave, haynes, cessna, hotly, turn, compute, premature,
Nearest to while: agouti, and, glycerol, leaves, catalog, medulla, button, devoting,
Nearest to is: was, are, by, were, agouti, has, operatorname, priestly,
Average loss at step 32000 : 5.98407553422
Average loss at step 34000 : 5.68312362564
Average loss at step 36000 : 5.80198390901
Average loss at step 38000 : 5.55209315801
Average loss at step 40000 : 5.26424281788
Nearest to or: and, albury, agouti, birkenau, circ, dasyprocta, bckgr, apatosaurus,
Nearest to over: seven, four, profound, gulfs, detect, georges, eight, two,
Nearest to state: iota, regeneration, derivation, clocking, mctaggart, kattegat, defensive, dasyprocta,
Nearest to so: mosque, prevailing, spinner, bryozoans, archie, marker, heaters, vs,
Nearest to united: beelzebub, cranmer, abkhazian, zoroastrianism, camacho, forbidding, altenberg, ethnicity,
Nearest to they: he, it, there, aorta, who, we, not, scripting,
Nearest to zero: eight, seven, nine, six, four, five, dasyprocta, three,
Nearest to by: was, in, gogh, were, be, is, with, birkenau,
Nearest to called: pregnancy, at, toys, sleep, circ, apologia, volvo, and,
Nearest to his: their, the, its, agouti, her, agave, s, baumgarten,
Nearest to most: indicate, albury, ridge, recitative, more, agouti, hbox, life,
Nearest to was: is, were, by, had, has, sensitive, been, be,
Nearest to all: some, absence, vs, beacons, cardinality, mishnayot, describing, vla,
Nearest to use: draught, agave, haynes, carrot, malice, cessna, hotly, turn,
Nearest to while: agouti, and, catalog, where, glycerol, leaves, or, button,
Nearest to is: was, are, has, were, agouti, by, priestly, birkenau,
Average loss at step 42000 : 5.33473952091
Average loss at step 44000 : 5.23443731594
Average loss at step 46000 : 5.21015189886
Average loss at step 48000 : 5.21985202241
Average loss at step 50000 : 4.97897449636
Nearest to or: and, albury, agouti, birkenau, three, dasyprocta, apatosaurus, seven,
Nearest to over: four, seven, profound, three, gulfs, two, dasyprocta, obtained,
Nearest to state: iota, regeneration, clocking, derivation, kattegat, defensive, mctaggart, during,
Nearest to so: mosque, prevailing, kapoor, spinner, marker, archie, tuition, heaters,
Nearest to united: beelzebub, cranmer, abkhazian, camacho, zoroastrianism, forbidding, kapoor, ethnicity,
Nearest to they: he, there, we, it, not, who, aorta, scripting,
Nearest to zero: eight, seven, five, four, six, nine, three, dasyprocta,
Nearest to by: was, be, with, gogh, were, in, as, birkenau,
Nearest to called: pregnancy, at, circ, sleep, volvo, toys, recitative, apologia,
Nearest to his: their, its, the, her, s, agouti, agave, baumgarten,
Nearest to most: indicate, more, ridge, albury, kapoor, bicycles, recitative, life,
Nearest to was: is, were, had, by, has, sensitive, became, be,
Nearest to all: some, two, three, vs, four, absence, mishnayot, altenberg,
Nearest to use: draught, agave, turn, haynes, carrot, compute, malice, cessna,
Nearest to while: and, agouti, where, leaves, for, catalog, button, glycerol,
Nearest to is: was, are, has, agouti, eight, were, be, operatorname,
Average loss at step 52000 : 5.02508091223
Average loss at step 54000 : 5.18100037599
Average loss at step 56000 : 5.03854976296
Average loss at step 58000 : 5.04216036534
Average loss at step 60000 : 4.94132102251
Nearest to or: and, albury, bckgr, agouti, circ, dasyprocta, three, wct,
Nearest to over: profound, five, seven, four, three, ursus, dasyprocta, after,
Nearest to state: iota, regeneration, clocking, derivation, during, kattegat, ursus, defensive,
Nearest to so: mosque, ursus, kapoor, prevailing, archie, marker, spinner, bryozoans,
Nearest to united: beelzebub, abkhazian, cranmer, camacho, zoroastrianism, kapoor, forbidding, ethnicity,
Nearest to they: he, there, we, it, who, not, but, you,
Nearest to zero: seven, eight, six, nine, four, five, three, dasyprocta,
Nearest to by: was, be, with, gogh, were, eight, agouti, birkenau,
Nearest to called: at, pregnancy, volvo, beacon, toys, circ, electrolyte, recitative,
Nearest to his: their, its, her, the, agouti, s, baumgarten, agave,
Nearest to most: more, indicate, albury, bicycles, kapoor, jong, some, ridge,
Nearest to was: is, were, had, by, has, sensitive, been, became,
Nearest to all: some, three, two, ursus, many, four, beacons, vs,
Nearest to use: draught, agave, compute, turn, agouti, malice, haynes, carrot,
Nearest to while: agouti, and, where, is, wct, leaves, button, for,
Nearest to is: was, are, has, agouti, operatorname, but, became, circ,
Average loss at step 62000 : 5.0153428297
Average loss at step 64000 : 4.8223820622
Average loss at step 66000 : 4.60808043647
Average loss at step 68000 : 4.96915031564
Average loss at step 70000 : 4.88760380042
Nearest to or: and, thaler, albury, bckgr, circ, apatosaurus, dasyprocta, ursus,
Nearest to over: four, profound, five, ursus, three, six, dasyprocta, coachella,
Nearest to state: iota, clocking, regeneration, derivation, during, ursus, defensive, kattegat,
Nearest to so: mosque, ursus, prevailing, marmoset, marker, kapoor, archie, simultaneously,
Nearest to united: beelzebub, abkhazian, cranmer, thaler, camacho, zoroastrianism, ethnicity, kapoor,
Nearest to they: he, there, we, it, who, you, not, unassigned,
Nearest to zero: eight, seven, six, five, four, nine, three, dasyprocta,
Nearest to by: was, be, gogh, mitral, mishnayot, were, rsha, been,
Nearest to called: at, pregnancy, volvo, circ, electrolyte, toys, recitative, and,
Nearest to his: their, its, the, her, agouti, s, baumgarten, agave,
Nearest to most: more, albury, indicate, some, jong, kapoor, meteorological, bicycles,
Nearest to was: is, were, had, has, by, sensitive, became, been,
Nearest to all: some, many, ursus, three, altenberg, agave, recessions, beacons,
Nearest to use: leontopithecus, draught, compute, agave, turn, malice, haynes, agouti,
Nearest to while: and, agouti, where, but, wct, upanija, when, operatorname,
Nearest to is: was, are, has, priestly, mico, agouti, operatorname, cebus,
Average loss at step 72000 : 4.73238538039
Average loss at step 74000 : 4.79503891718
Average loss at step 76000 : 4.7304057287
Average loss at step 78000 : 4.80684278604
Average loss at step 80000 : 4.80336784804
Nearest to or: and, thaler, albury, agouti, bckgr, mico, apatosaurus, circ,
Nearest to over: four, five, ursus, profound, trilled, dasyprocta, six, biscuit,
Nearest to state: clocking, regeneration, iota, during, derivation, city, kattegat, dasyprocta,
Nearest to so: mosque, marker, ursus, marmoset, kapoor, prevailing, limehouse, moor,
Nearest to united: beelzebub, abkhazian, cranmer, camacho, ethnicity, zoroastrianism, thaler, forbidding,
Nearest to they: he, we, there, it, you, who, not, unassigned,
Nearest to zero: five, six, four, eight, nine, seven, dasyprocta, mico,
Nearest to by: gogh, was, be, were, mitral, with, through, been,
Nearest to called: at, and, pregnancy, circ, volvo, electrolyte, iit, recitative,
Nearest to his: their, its, her, the, s, baumgarten, agouti, him,
Nearest to most: more, some, albury, many, indicate, kapoor, agouti, iit,
Nearest to was: is, were, had, has, became, sensitive, been, by,
Nearest to all: some, many, ursus, three, two, both, these, mishnayot,
Nearest to use: draught, leontopithecus, compute, turn, malice, agave, hotly, haynes,
Nearest to while: where, agouti, but, wct, however, and, when, upanija,
Nearest to is: was, are, has, agouti, mico, iit, operatorname, invertible,
Average loss at step 82000 : 4.74254066306
Average loss at step 84000 : 4.74353921854
Average loss at step 86000 : 4.76269113183
Average loss at step 88000 : 4.74936543357
Average loss at step 90000 : 4.73277113521
Nearest to or: and, thaler, bckgr, circ, agouti, albury, apatosaurus, but,
Nearest to over: four, however, ursus, profound, five, trilled, candide, about,
Nearest to state: regeneration, clocking, iota, during, city, dasyprocta, derivation, ursus,
Nearest to so: mosque, ursus, marmoset, marker, kapoor, escuela, limehouse, moor,
Nearest to united: beelzebub, abkhazian, camacho, ethnicity, cranmer, thaler, forbidding, zoroastrianism,
Nearest to they: he, we, there, you, who, it, not, unassigned,
Nearest to zero: eight, five, seven, six, four, nine, dasyprocta, mico,
Nearest to by: was, gogh, mitral, through, be, with, mishnayot, were,
Nearest to called: calypso, at, pregnancy, electrolyte, volvo, circ, iit, UNK,
Nearest to his: their, its, her, the, s, baumgarten, agouti, him,
Nearest to most: more, some, many, albury, indicate, jong, iit, kapoor,
Nearest to was: is, had, has, were, became, been, by, sensitive,
Nearest to all: some, many, both, ursus, these, altenberg, three, various,
Nearest to use: leontopithecus, draught, agave, turn, compute, agouti, futurist, malice,
Nearest to while: where, however, and, agouti, when, but, wct, upanija,
Nearest to is: was, has, are, be, became, mico, agouti, operatorname,
Average loss at step 92000 : 4.66651302779
Average loss at step 94000 : 4.72329252827
Average loss at step 96000 : 4.70242218912
Average loss at step 98000 : 4.57907619059
Average loss at step 100000 : 4.6917175796
Nearest to or: and, thaler, but, albury, agouti, dasyprocta, ursus, bckgr,
Nearest to over: four, five, ursus, about, profound, dasyprocta, three, candide,
Nearest to state: regeneration, clocking, iota, city, dasyprocta, boutros, during, derivation,
Nearest to so: mosque, marmoset, ursus, kapoor, dmd, escuela, limehouse, moor,
Nearest to united: beelzebub, abkhazian, camacho, ethnicity, cranmer, forbidding, thaler, zoroastrianism,
Nearest to they: he, we, there, it, you, not, who, unassigned,
Nearest to zero: eight, seven, five, four, nine, six, dasyprocta, mico,
Nearest to by: gogh, was, be, with, through, mishnayot, mitral, as,
Nearest to called: calypso, electrolyte, volvo, pregnancy, at, circ, used, iit,
Nearest to his: their, her, its, the, s, agouti, columbus, baumgarten,
Nearest to most: more, many, some, albury, jong, less, indicate, iit,
Nearest to was: is, had, has, were, became, been, being, by,
Nearest to all: some, many, both, these, various, ursus, altenberg, two,
Nearest to use: leontopithecus, draught, agave, agouti, turn, compute, ursus, dmd,
Nearest to while: and, when, where, stenella, although, however, but, agouti,
Nearest to is: was, has, are, stenella, became, agouti, mico, be,
Process finished with exit code 0
可以看到,挑选出来的几个词的最近邻结果,was
、zero
的结果应该是里面最好的,其他的感觉都比较差,有5个词最近邻结果集中都出现了agouti
。
总体很一般吧。
可视化结果如下图。
Recommend
About Joyk
Aggregate valuable and interesting links.
Joyk means Joy of geeK