

t-SNE(t-distributed Stochastic Neighbor Embedding)简介与示例
source link: https://ylhao.github.io/2019/05/13/268/
Go to the source link to view the article. You can view the picture content, updated content and better typesetting reading experience. If the link is broken, please click the button below to view the snapshot at that time.
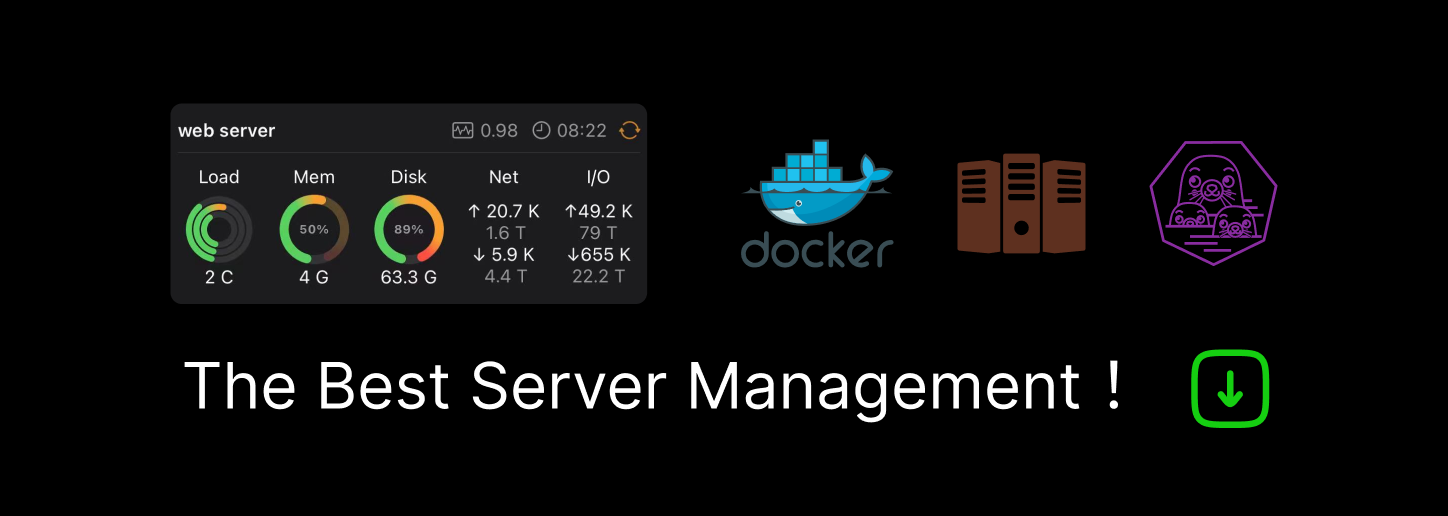
manifold
可以称之为流形数据。像绳结一样的数据,虽然在高维空间中可分,但是在人眼所看到的低维空间中,绳结中的绳子是互相重叠的不可分的。
t-SNE
t-SNE是目前来说效果最好的数据降维与可视化方法,但是它的缺点也很明显,比如:占内存大,运行时间长。但是,当我们想要对高维数据进行分类,又不清楚这个数据集有没有很好的可分性(即同类之间间隔小,异类之间间隔大),可以通过t-SNE投影到2维或者3维的空间中观察一下。如果在低维空间中具有可分性,则数据是可分的。如果在低维空间中不具有可分性,可能是数据不可分,也可能仅仅是因为不能投影到低维空间。
t-SNE将数据点之间的相似度转换为概率。原始空间中的相似度由高斯联合概率表示,嵌入空间的相似度由“学生t分布”表示。
通过原始空间和嵌入空间的联合概率的Kullback-Leibler(KL)散度来评估可视化效果的好坏,也就是说用有关KL散度的函数作为loss函数,然后通过梯度下降最小化loss函数,最终获得收敛结果。需要注意的是该loss函数不是凸函数,即具有不同初始值的多次运行可能收敛到KL散度函数的不同局部最小值,获得不同的结果。因此可以尝试不同的随机数种子(Python中可以通过设置seed来获得不同的随机分布),最后选择具有最低KL散度值的结果。
t-SNE的缺点是:
- t-SNE的计算复杂度很高,在数百万个样本数据集中可能需要几个小时,而PCA可以在几秒钟或几分钟内完成。
- Barnes-Hut t-SNE方法(下面讲)限于二维或三维嵌入。
- 算法是随机的,具有不同种子的多次实验可以产生不同的结果。虽然选择loss最小的结果就行,但可能需要多次实验以选择超参数。
- 全局结构未明确保留。这个问题可以通过PCA初始化点(使用init =’pca’)来缓解。
t-SNE的主要目的是高维数据的可视化。因此,当数据嵌入二维或三维时,效果最好。有五个参数可以控制t-SNE的优化,即会影响最后的可视化质量:
- perplexity:困惑度
- early exaggeration factor:前期放大系数
- learning rate:学习率
- maximum number of iterations:最大迭代次数
- angle: 角度
Barnes-Hut t-SNE
Barnes-Hut t-SNE主要是对传统t-SNE在速度上做了优化,是现在最流行的t-SNE方法,同时它与传统t-SNE还有一些不同:
- Barnes-Hut仅在目标维度为3或更小时才起作用。以2D可视化为主。
- Barnes-Hut仅适用于密集的输入数据。稀疏数据矩阵只能用特定的方法嵌入,或者可以通过投影近似,例如使用sklearn.decomposition.TruncatedSVD
- Barnes-Hut是一个近似值。使用angle参数对近似进行控制,当参数
method="exact"
时,TSNE()
使用传统方法,此时angle参数不能使用。 - Barnes-Hut可以处理更多的数据。 Barnes-Hut可用于嵌入数十万个数据点。
为了可视化的目的(这是t-SNE的主要用处),强烈建议使用Barnes-Hut方法。method="exact"
时,传统的t-SNE方法尽管可以达到该算法的理论极限,效果更好,但受制于计算约束,只能对小数据集的可视化。
数据集在所有特征维度上的尺度应该相同。
import numpy as np
from time import time
import matplotlib.pyplot as plt
from mpl_toolkits.mplot3d import Axes3D
from matplotlib.ticker import NullFormatter
from sklearn import manifold, datasets
%matplotlib inline
X = np.array([[0, 0, 0], [0, 1, 1], [1, 0, 1], [1, 1, 1]])
tsne = manifold.TSNE(n_components=2)
tsne.fit_transform(X)
print(tsne.embedding_)
// 输出
[[-520.725 302.84378 ]
[-116.2318 369.94275 ]
[-453.6018 -101.645386]
[ -49.10863 -34.546448]]
n_points = 1000
X, color = datasets.samples_generator.make_s_curve(n_points, random_state=0)
n_neighbors = 10
n_components = 2
print(X.shape)
print(X[:10])
print(color.shape)
print(color[:10])
// 输出
(1000, 3)
[[ 0.44399868 1.18576054 -0.10397256]
[ 0.89724097 0.02012739 -1.44154121]
[ 0.8240493 0.95165239 -0.43348191]
[ 0.41051068 1.41754078 -0.08814421]
[-0.65903619 0.08795086 0.24788877]
[ 0.98089687 1.75904297 -0.80547154]
[-0.55488662 1.04016283 0.16807402]
[-0.5233528 0.0613221 -1.8521161 ]
[-0.94192794 0.44882722 -1.33581507]
[-0.89054316 1.90735139 0.54510124]]
(1000,)
[ 0.46005644 2.028112 0.968522 0.42301403 -0.71953656 1.37501962
-0.58822668 3.69237354 4.36991857 -1.0985378 ]
fig = plt.figure(figsize=(8, 8))
# 创建了一个figure,标题为"Manifold Learning with 1000 points, 10 neighbors"
plt.suptitle("Manifold Learning with %i points, %i neighbors"
% (1000, n_neighbors), fontsize=14)
'''绘制S曲线的3D图像'''
ax = fig.add_subplot(211, projection='3d')
ax.scatter(X[:, 0], X[:, 1], X[:, 2], c=color, cmap=plt.cm.Spectral)
ax.view_init(4, -72) # 初始化视角
# t=SME
t0 = time()
tsne = manifold.TSNE(n_components=n_components, init='pca', random_state=0)
Y = tsne.fit_transform(X) # 转换后的输出
t1 = time()
print("t-SNE: %.2g sec" % (t1 - t0)) # 打印算法用时
ax = fig.add_subplot(2, 1, 2)
plt.scatter(Y[:, 0], Y[:, 1], c=color, cmap=plt.cm.Spectral)
plt.title("t-SNE (%.2g sec)" % (t1 - t0))
ax.xaxis.set_major_formatter(NullFormatter()) # 设置标签显示格式为空
ax.yaxis.set_major_formatter(NullFormatter())
plt.show()
// 输出
t-SNE: 21 sec
转载请注明来源,欢迎对文章中的引用来源进行考证,欢迎指出任何有错误或不够清晰的表达,可以在文章下方的评论区进行评论,也可以邮件至 [email protected]
Recommend
About Joyk
Aggregate valuable and interesting links.
Joyk means Joy of geeK