

Basic Python Profiling
source link: https://bbengfort.github.io/2020/07/basic-python-profiling/
Go to the source link to view the article. You can view the picture content, updated content and better typesetting reading experience. If the link is broken, please click the button below to view the snapshot at that time.
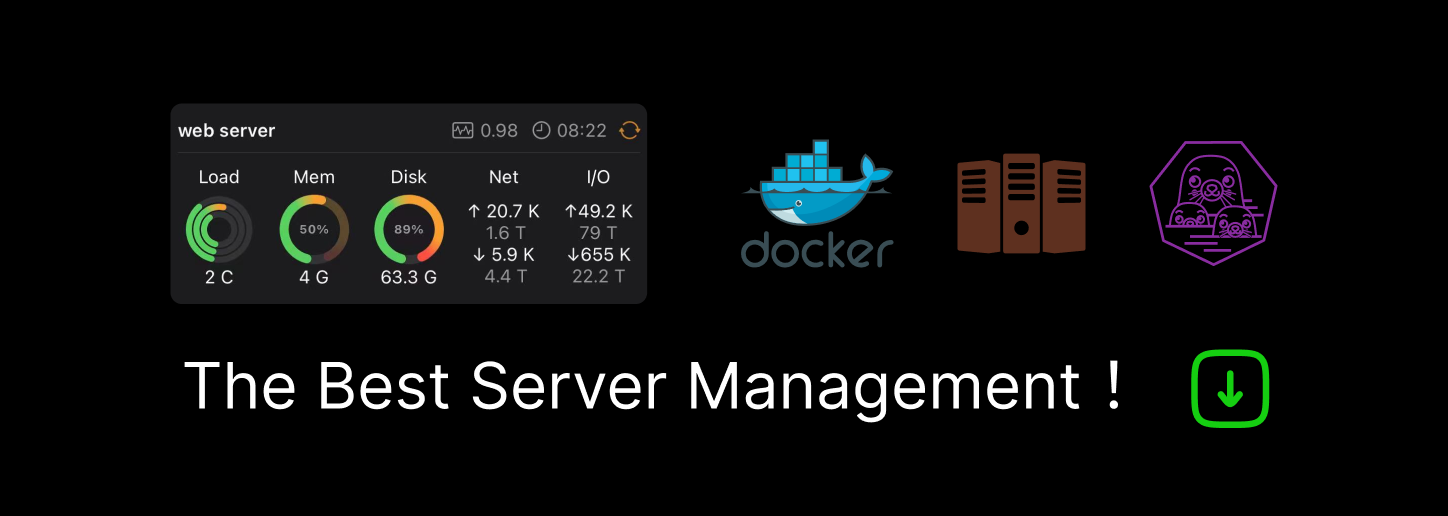
Basic Python Profiling
I’m getting started on some projects that will make use of extensive Python performance profiling, unfortunately Python doesn’t focus on performance and so doesn’t have benchmark tools like I might find in Go. I’ve noticed that the two most important usages I’m looking at when profiling are speed and memory usage. For the latter, I simply use memory_profiler
from the command line - which is pretty straight forward. However for speed usage, I did find a snippet that I thought would be useful to include and update depending on how my usage changes.
import cProfile
from pstats import Stats
from functools import wraps
def sprofile(func):
@wraps(func)
def wrapper(*args, **kwargs):
pr = cProfile.Profile()
pr.enable()
result = func(*args, **kwargs)
pr.disable()
Stats(pr).strip_dirs().sort_stats('cumulative').print_stats(20)
return result
return wrapper
This decorator allows you to profile the speed performance of functions on the stack below the function being decorated. It uses standard library dependencies, which is great, and you can change the way the stats are printed out to suit your needs (e.g. this is formatted well for my analysis style).
The report it prints out is as follows:
7636523 function calls (7636479 primitive calls) in 14.669 seconds
Ordered by: cumulative time
List reduced from 306 to 20 due to restriction <20>
ncalls tottime percall cumtime percall filename:lineno(function)
1 0.000 0.000 14.669 14.669 sequential.py:107(run)
150 2.584 0.017 14.633 0.098 sequential.py:75(step)
843750 10.228 0.000 10.335 0.000 grid.py:72(neighborhood_sum)
843750 0.765 0.000 0.988 0.000 grid.py:129(__setitem__)
843750 0.529 0.000 0.726 0.000 grid.py:124(__getitem__)
2531454 0.275 0.000 0.275 0.000 {built-in method builtins.isinstance}
1687783 0.145 0.000 0.145 0.000 {built-in method builtins.len}
843750 0.107 0.000 0.107 0.000 grid.py:57(adjacency)
151 0.001 0.000 0.020 0.000 std.py:1099(__iter__)
82 0.000 0.000 0.019 0.000 std.py:1317(refresh)
83 0.000 0.000 0.017 0.000 std.py:1447(display)
83 0.000 0.000 0.015 0.000 std.py:1089(__repr__)
9/4 0.000 0.000 0.014 0.004 <frozen importlib._bootstrap>:978(_find_and_load)
9/4 0.000 0.000 0.014 0.004 <frozen importlib._bootstrap>:948(_find_and_load_unlocked)
83 0.002 0.000 0.014 0.000 std.py:310(format_meter)
9/4 0.000 0.000 0.013 0.003 <frozen importlib._bootstrap>:663(_load_unlocked)
17/6 0.000 0.000 0.011 0.002 <frozen importlib._bootstrap>:211(_call_with_frames_removed)
1 0.000 0.000 0.010 0.010 std.py:511(__new__)
1 0.000 0.000 0.009 0.009 std.py:623(get_lock)
1 0.000 0.000 0.009 0.009 std.py:79(__init__)
You can see in this report that the majority of time is being spent in the neighborhood_sum
function from line 3 and that the step
function calls it nearly 5,625 times!
Recommend
About Joyk
Aggregate valuable and interesting links.
Joyk means Joy of geeK