

scikit-learn中的GBDT实现
source link: https://liangyaorong.github.io/blog/2017/scikit-learn%E4%B8%AD%E7%9A%84GBDT%E5%AE%9E%E7%8E%B0/
Go to the source link to view the article. You can view the picture content, updated content and better typesetting reading experience. If the link is broken, please click the button below to view the snapshot at that time.
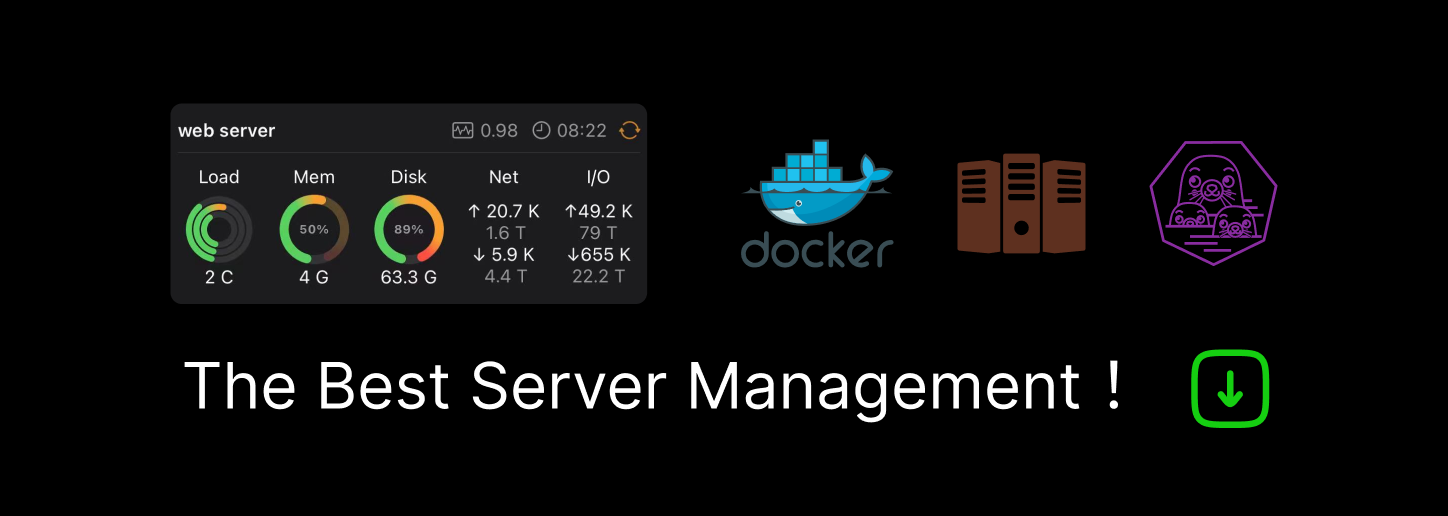
scikit-learn中的GBDT实现
上一篇文章中我们已经大概了解了Gradient Boosting的来源和主要数学思想。在这篇文章里,我们将以sklearn中的Gradient Boosting为基础 源码在这,了解GBDT的实现过程.希望大家能在看这篇文章的过程中有所收获.
这里面会有大量的代码,请耐住性子,我们一起把它啃下来.
什么是GBDT
GBDT全称是Gradient Boosting Decision Trees,顾名思义,就是在梯度提升框架下(上一篇文章有详细解说传送门),用回归树作为基本分类器的算法(分类树相加会有问题,如男+女=?).因此可以想到,参数调优时有两方面:
1.梯度提升框架方面:
- “损失函数 loss”
- “步长 learning_rate”
- “迭代次数 n_estimators”
- “样本权重”
2.决策树方面:
- “最大深度max_depth”
- “特征分割标准criterion(默认为friedman_mse)”
- “最小样本分割次数min_samples_split ”
- “叶子中最小样本数min_samples_leaf ”
- “特征选取数max_features”
- “最大叶子数max_leaf_nodes ”
- “重采样比例subsample”…
大家可以参考sklearn的用户手册Guide
为什么要用决策树作为基本分类器?
决策树优点在于可理解性强,可以快速生成我们能理解的规则,而且计算量相对而言不大(每棵树的复杂度为O(mnlog(Depth))).
但决策树有一个很大的缺点:高方差和不稳定.由于决策数是运用启发式生成的,初始分割点的一点改变就会导致最后结果完全不同.这使得决策树对特征的要求非常高.但实际中特征噪声是很多的.
因此它是典型的弱分类器.
GBDT的中的数学
当我们的基本分类器是一个包含J个节点的回归树时,回归树模型可以表示为
其中Rj为不相交的区域,它们的集合覆盖了预测值空间,bj是叶子节点的值.
因此,在回归树为基模型下,算法最终的结果为:
通俗的理解就是这样:
说白了就是每次的预测相加.不难理解吧
了解了最基本的构造,我们接着看一下GBDT的代码实现
片段一(回归树叶子节点估计,以取平均为例)
class MeanEstimator(BaseEstimator):
"""An estimator predicting the mean of the training targets."""
def fit(self, X, y, sample_weight=None):
if sample_weight is None:
self.mean = np.mean(y)
else:
self.mean = np.average(y, weights=sample_weight)
def predict(self, X):
check_is_fitted(self, 'mean')
y = np.empty((X.shape[0], 1), dtype=np.float64)
y.fill(self.mean)
return y
若无样本权重,则简单求和;若有样本权重,则加权求和
除平均之外,叶子节点取值方式还有:
- 对数优势比(log Odd Ratio)
片段二(回归器损失函数,以平方损失为例)
class LeastSquaresError(RegressionLossFunction):
def init_estimator(self):
return MeanEstimator()
def __call__(self, y, pred, sample_weight=None):
if sample_weight is None:
return np.mean((y - pred.ravel()) ** 2.0)
else:
return (1.0 / sample_weight.sum() *
np.sum(sample_weight * ((y - pred.ravel()) ** 2.0)))
def negative_gradient(self, y, pred, **kargs):
return y - pred.ravel()
def update_terminal_regions(self, tree, X, y, residual, y_pred,
sample_weight, sample_mask,
learning_rate=1.0, k=0):
"""Least squares does not need to update terminal regions.
But it has to update the predictions.
"""
# update predictions
y_pred[:, k] += learning_rate * tree.predict(X).ravel()
def _update_terminal_region(self, tree, terminal_regions, leaf, X, y, residual, pred, sample_weight):
pass
我们可以看到损失函数类有三个方法:
- 将预测集在该轮训练得到的结果添加到最终预测中
除平方损失之外,
对于Regressor还有
- Huber损失
- 分位数损失
片段三(分类器损失函数,以指数损失为例)
class ExponentialLoss(ClassificationLossFunction):
"""Exponential loss function for binary classification.
Same loss as AdaBoost.
References
----------
Greg Ridgeway, Generalized Boosted Models: A guide to the gbm package, 2007
"""
def __init__(self, n_classes):
if n_classes != 2:
raise ValueError("{0:s} requires 2 classes.".format(
self.__class__.__name__))
# we only need to fit one tree for binary clf.
super(ExponentialLoss, self).__init__(1)
def init_estimator(self):
return ScaledLogOddsEstimator()
def __call__(self, y, pred, sample_weight=None):
pred = pred.ravel()
if sample_weight is None:
return np.mean(np.exp(-(2. * y - 1.) * pred))
else:
return (1.0 / sample_weight.sum() *
np.sum(sample_weight * np.exp(-(2 * y - 1) * pred)))
def negative_gradient(self, y, pred, **kargs):
y_ = -(2. * y - 1.)
return y_ * np.exp(y_ * pred.ravel())
def _update_terminal_region(self, tree, terminal_regions, leaf, X, y,
residual, pred, sample_weight):
terminal_region = np.where(terminal_regions == leaf)[0]
pred = pred.take(terminal_region, axis=0)
y = y.take(terminal_region, axis=0)
sample_weight = sample_weight.take(terminal_region, axis=0)
y_ = 2. * y - 1.
numerator = np.sum(y_ * sample_weight * np.exp(-y_ * pred))
denominator = np.sum(sample_weight * np.exp(-y_ * pred))
# prevents overflow and division by zero
if abs(denominator) < 1e-150:
tree.value[leaf, 0, 0] = 0.0
else:
tree.value[leaf, 0, 0] = numerator / denominator
def _score_to_proba(self, score):
proba = np.ones((score.shape[0], 2), dtype=np.float64)
proba[:, 1] = expit(2.0 * score.ravel())
proba[:, 0] -= proba[:, 1]
return proba
def _score_to_decision(self, score):
return (score.ravel() >= 0.0).astype(np.int)
和回归器的损失函数不同,分类器的损失函数类多了两个方法:
- 1._score_to_proba():将得分转化为概率
- 2._score_to_decision():将得分转化为最后的分类决策
ps:这两个函数的出现是因为GBDT中,所有基模型都是回归树,这在上面已经讲过了.所以需要将连续的结果离散化
除指数损失外,
对于classifier还有
- 二项偏差(Binomial Deviance)
- 多项偏差(Multinomial Deviance)
片段四(GBDT的训练 _fit_stages)
class BaseGradientBoosting(six.with_metaclass(ABCMeta, BaseEnsemble)):
"""Abstract base class for Gradient Boosting. """
@abstractmethod
def __init__(...):
...
...
def _fit_stages(self, X, y, y_pred, sample_weight, random_state,
begin_at_stage=0, monitor=None, X_idx_sorted=None):
"""Iteratively fits the stages.
For each stage it computes the progress (OOB, train score)
and delegates to ``_fit_stage``.
Returns the number of stages fit; might differ from ``n_estimators``
due to early stopping.
"""
1-> n_samples = X.shape[0]
do_oob = self.subsample < 1.0
sample_mask = np.ones((n_samples,), dtype=np.bool)
n_inbag = max(1, int(self.subsample * n_samples))
loss_ = self.loss_
# Set min_weight_leaf from min_weight_fraction_leaf
if self.min_weight_fraction_leaf != 0. and sample_weight is not None:
min_weight_leaf = (self.min_weight_fraction_leaf *
np.sum(sample_weight))
else:
min_weight_leaf = 0.
if self.verbose:
verbose_reporter = VerboseReporter(self.verbose)
verbose_reporter.init(self, begin_at_stage)
X_csc = csc_matrix(X) if issparse(X) else None
X_csr = csr_matrix(X) if issparse(X) else None
# perform boosting iterations
i = begin_at_stage
2-> for i in range(begin_at_stage, self.n_estimators):
# subsampling
if do_oob:
3-> sample_mask = _random_sample_mask(n_samples, n_inbag,random_state)
# OOB score before adding this stage
4-> old_oob_score = loss_(y[~sample_mask],
y_pred[~sample_mask],
sample_weight[~sample_mask])
# fit next stage of trees
5-> y_pred = self._fit_stage(i, X, y, y_pred, sample_weight,
sample_mask, random_state, X_idx_sorted,
X_csc, X_csr)
# track deviance (= loss)
6-> if do_oob:
self.train_score_[i] = loss_(y[sample_mask], #更新train_score_
y_pred[sample_mask],
sample_weight[sample_mask])
self.oob_improvement_[i] = (
old_oob_score - loss_(y[~sample_mask],
y_pred[~sample_mask],
sample_weight[~sample_mask]))
7-> else:
# no need to fancy index w/ no subsampling
self.train_score_[i] = loss_(y, y_pred, sample_weight)
#update verbose_reporter
8-> if self.verbose > 0:
verbose_reporter.update(i, self)
#update monitor
9-> if monitor is not None:
early_stopping = monitor(i, self, locals())
if early_stopping:
break
return i + 1
根据代码中的标号,我们一步一步来看
- 1.训练前的初始化,以及做好输入检测
- 2.进入T轮迭代,T=n_estimators. 注意,若采用重采样进行训练,若OOB中出现过拟合,会提前停止迭代(由monitor决定)
- 确定训练样本
- 计算原始oob error
- 调用_fit_stage进行当前轮训练并返回最新y_pred(!重点!)
- 计算oob_improvement_
- 更新train_score_
- 更新verbose_reporter
- 更新monitor,判断是否要提前停止迭代
是否很好奇,流程5是怎么实现的?我们接着看
片段五(迭代中每一轮的训练 _fit_stage)
def _fit_stage(self, i, X, y, y_pred, sample_weight, sample_mask,
random_state, X_idx_sorted, X_csc=None, X_csr=None):
"""Fit another stage of ``n_classes_`` trees to the boosting model. """
assert sample_mask.dtype == np.bool # if flase, raise error
loss = self.loss_
original_y = y
for k in range(loss.K):
if loss.is_multi_class:
y = np.array(original_y == k, dtype=np.float64)
1-> residual = loss.negative_gradient(y, y_pred, k=k,
sample_weight=sample_weight)
# induce regression tree on residuals
2-> tree = DecisionTreeRegressor(
criterion=self.criterion,
splitter='best',
max_depth=self.max_depth,
min_samples_split=self.min_samples_split,
min_samples_leaf=self.min_samples_leaf,
min_weight_fraction_leaf=self.min_weight_fraction_leaf,
min_impurity_split=self.min_impurity_split,
max_features=self.max_features,
max_leaf_nodes=self.max_leaf_nodes,
random_state=random_state,
presort=self.presort)
3-> if self.subsample < 1.0:
# no inplace multiplication!
sample_weight = sample_weight * sample_mask.astype(np.float64)
4-> if X_csc is not None:
tree.fit(X_csc, residual, sample_weight=sample_weight,
check_input=False, X_idx_sorted=X_idx_sorted)
else:
tree.fit(X, residual, sample_weight=sample_weight,
check_input=False, X_idx_sorted=X_idx_sorted)
# update tree leaves
5-> if X_csr is not None:
loss.update_terminal_regions(tree.tree_, X_csr, y, residual, y_pred,
sample_weight, sample_mask,
self.learning_rate, k=k)
else:
loss.update_terminal_regions(tree.tree_, X, y, residual, y_pred,
sample_weight, sample_mask,
self.learning_rate, k=k)
6-> # add tree to ensemble
self.estimators_[i, k] = tree
7-> return y_pred
假设大家已经对决策树的生成与预测有所了解,这篇文章里就不展开讲了.想看决策树基础代码的同学可以看一下这个传送门(代码来自《机器学习实战》,我加了一些注释)
函数_fit_stage展示的是GBDT每一轮里的训练过程.主要分为7部分(标号).
- 1.计算负梯度
- 2.初始化基模型(GBDT的基模型就是决策树)
- 3.若用子样本训练,则获取样本权重对应子集
- 4.用基模型进行该轮训练
- 5.更新预测空间的划分及其取值
- 6.将该轮模型储藏起来,用于以后的泛化
- 7.返回最新的预测值
注意,这里面有一个for循环,其实是针对分类的(二项分类与多项分类).
若是分类,模型会根据标签(label)类别对y进行重构,相同的一label放在一列.在训练中对每一列分别进行训练.
若是连续型的预测,很显然n_classes_==1,即进入一次循环就好了
其实也不是很复杂对不对.整个过程要注意的细节就是子样本的采样和分类器的训练
当然还有一些细节如:
- verbose_reporter的实现
- monitor的实现
这些就留给你们自己去研究了.看懂了收益也会很大吧
片段六(GBDT的预测)
预测对于分类器和回归器来说是不同的,但是在Base Gradient Boosting类中有他们共同要用到的函数
1-> def _init_decision_function(self, X):
self._check_initialized()
X = self.estimators_[0, 0]._validate_X_predict(X, check_input=True)
if X.shape[1] != self.n_features_:
raise ValueError("X.shape[1] should be {0:d}, not {1:d}.".format(
self.n_features_, X.shape[1]))
score = self.init_.predict(X).astype(np.float64)
return score
2-> def _decision_function(self, X):
score = self._init_decision_function(X)
predict_stages(self.estimators_, X, self.learning_rate, score)
return score
注:predict_stages()函数在_gradient_boosting.pyx中,用的cython写的,其实就是循环每一轮的基模型来预测.前面讲过所有训练好的基模型都储存在estimators_中. 这里就不深入了
我们看回来,对于上面的两个函数
- 1.初始化一个预测(循环之前总得先有个初始残差嘛)
- 2.对所有储存好的基模型进行迭代预测
接着我们分别看一下分类器和回归器的预测
分类器
class GradientBoostingClassifier(BaseGradientBoosting, ClassifierMixin):
...
...
def decision_function(self, X):
"""Compute the decision function of ``X``.
X = check_array(X, dtype=DTYPE, order="C", accept_sparse='csr')
score = self._decision_function(X)
if score.shape[1] == 1:
return score.ravel()
return score
def predict(self, X):
"""Predict class for X."""
score = self.decision_function(X)
decisions = self.loss_._score_to_decision(score)
return self.classes_.take(decisions, axis=0)
回归器
class GradientBoostingRegressor(BaseGradientBoosting, RegressorMixin):
...
...
def predict(self, X):
"""Predict regression target for X."""
X = check_array(X, dtype=DTYPE, order="C", accept_sparse='csr')
return self._decision_function(X).ravel()
分类器比回归归器的预测步骤多了一个decision_function函数,就是因为上面说过对于分类器,训练时会将不同label的样本进行重排.这里只是对预测出来的就过做个分类规范化处理而已.
当然,分类器中最后得到的答案还要进行离散化,调用的函数就是之前损失函数中的方法_score_to_decision().
好啦,到这里,我们对GBDT的源码解读就差不多啦,更多的细节大家可以深入阅读源码.
如果文章有不当的地方欢迎指出.页面下方有我的电子邮箱,谢谢大家的指点!
下一篇文章将会讲一下集成学习中另一个分支:bagging.下篇文章见吧.
Recommend
About Joyk
Aggregate valuable and interesting links.
Joyk means Joy of geeK