

Sean的学习博客
source link: https://seanys.github.io/2020/12/10/%E6%8F%90%E9%AB%98%E8%AE%A1%E7%AE%97%E6%95%88%E7%8E%87%E7%AD%96%E7%95%A5/
Go to the source link to view the article. You can view the picture content, updated content and better typesetting reading experience. If the link is broken, please click the button below to view the snapshot at that time.
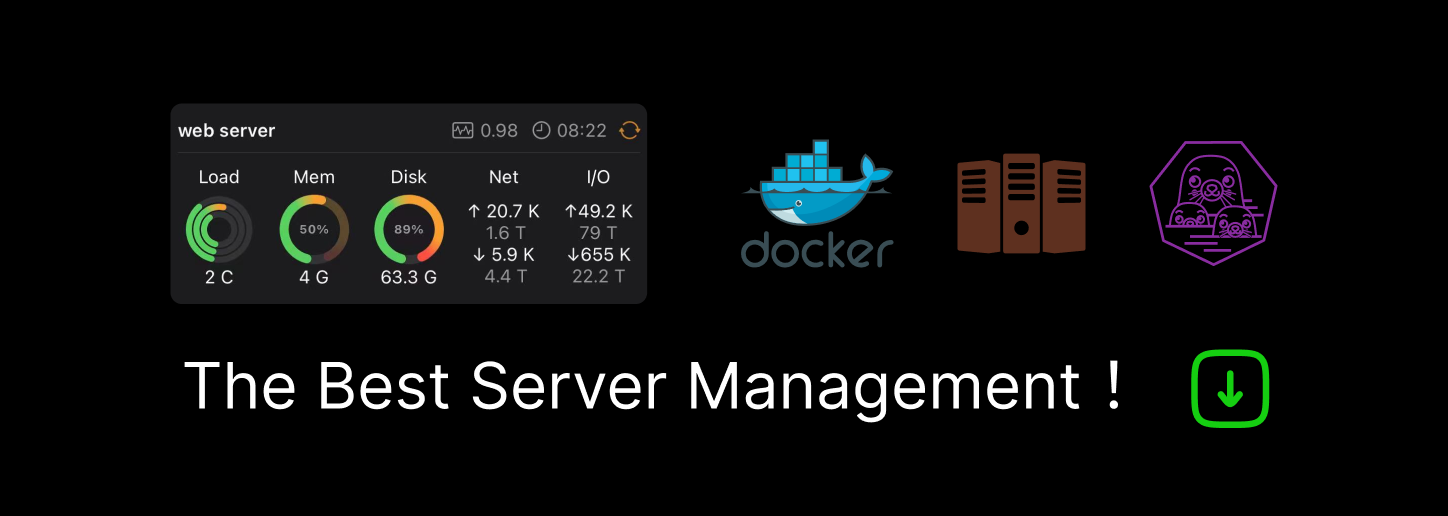
计算效率提高策略
今年做的二维排样和拼车都面临大规模数据的计算,其中二维排样NP-hard,求解时间非常长,在迭代求解均衡状态的程序中,由于Python的for循环计算很慢,同时数据量过大,会造成计算时间非常长,以我们当前的研究内容为例,解释如何进行速度优化。
策略一-矩阵计算
在部分计算中,存在非常多的循环计算,就会出现大量的for循环,这种情况下,需要想办法将部分运算改为numpy计算,由于底层采用了C++编写,其效率会更高。
import numpy as np
arr1 = np.array([1,2,3])
arr2 = np.array([2,3,4])
print(arr1*arr2.T)
策略二-字典检索
相对而言,dictionary的检索速度比list的速度会更快,我们的数据存储存在origin vertex和destination vertex,共同存储在OD中,这就需要存储为二维数组。由于:
- 并非全部的OD都存在合适的订单,如果存储二维数组会出现大量冗余数据
- List的读取速度和检索速度都不及Dictionary,后者的检索速度为O(1),在检索规模比较大的时候,list判断是否存在速度非常慢
所以,优先采用字典进行处理
# 设计唯一Key值
dic = {}
key = ""
dic[key] = "detail"
# 查询是否存在
if key in dic:
print("exist")
# 遍历全部Key
for key in dic.keys():
print(key)
策略三-分析计算内容
在大规模的数据计算中,会出现部分函数的执行时间过长,建议通过Cprofile进行时间分析,比如以下分析可以看到,obtainLamS所花费的时间是最长的,同时还有obtainP,然后我们就可以通过数据结构优化、算法优化等策略,优化该函数,降低执行次数。
34372393 function calls (34191034 primitive calls) in 123.443 seconds
Ordered by: standard name
ncalls tottime percall cumtime percall filename:lineno(function)
236 8.009 0.034 8.010 0.034 prediction.py:377(obtainLamSDNA)
236 74.598 0.316 74.629 0.316 prediction.py:390(obtainLamS)
236 20.741 0.088 21.518 0.091 prediction.py:408(obtainP)
1 0.005 0.005 0.006 0.006 prediction.py:426(getNumberLamSN)
1416 0.007 0.000 9.689 0.007 prediction.py:433(getBias)
1416 7.893 0.006 9.216 0.007 prediction.py:435(<listcomp>)
16865032 1.322 0.000 1.322 0.000 {built-in method builtins.abs}
策略四-多进程计算
在部分情况下,可以采用多进程计算的方式,优化计算速度,主要采用multiprocess模块。但是多进程计算不是所有的都使用,比如在排样算法求解中,理论上是在分担任务的过程中,时间损耗,导致最后效果不是特别好;
# 多金衡案例
from multiprocessing import Pool
from time import sleep
from datetime import datetime
def test(i):
print(f'{i} is doing...')
sleep(3)
if __name__ == '__main__':
p = Pool(4)
print(datetime.now())
for i in range(4):
p.apply_async(test, (i,))
p.close()
p.join()
print(datetime.now())
# 求解过程中的多进程方案
...
def obtainLamSN(self,_cur,_last):
'''公式3-4计算'''
p = Pool()
p.apply_async(self.obtainLamSMulti, args=(_cur,_last,self.all_node_keys[:int(self.all_node_num/3)],))
p.apply_async(self.obtainLamSMulti, args=(_cur,_last,self.all_node_keys[int(self.all_node_num/3):int(self.all_node_num*2/3)],))
p.apply_async(self.obtainLamSMulti, args=(_cur,_last,self.all_node_keys[int(self.all_node_num*2/3):],))
p.close()
p.join()
def obtainLamSMulti(self,_cur,_last):
for i in keys:
if self.ALL_NODES[i]["matching_segments"] == []: continue
first_segment = self.ALL_NODES[i]["matching_segments"][0]
s_n = {first_segment : self.lam_n_a[i][_last]}
self.lam_s_n[first_segment][i][_cur] = self.lam_n_a[i][_last]
for j in range(len(self.ALL_NODES[i]["matching_segments"])-1):
last_segment_key = self.ALL_NODES[i]["matching_segments"][j]
current_segment_key = self.ALL_NODES[i]["matching_segments"][j+1]
s_n[current_segment_key] = s_n[last_segment_key] * (1 - self.P_s_e[last_segment_key][_last])
self.lam_s_n[current_segment_key][i][_cur] = s_n[current_segment_key]
策略五-Numba
在采用Numpy计算和非常多循环的情况下,可以采用Numba进行优化,但是暂时没有测试成功,主要
from numba import jit
import random
@jit(nopython=True)
def monte_carlo_pi(nsamples):
acc = 0
for i in range(nsamples):
x = random.random()
y = random.random()
if (x ** 2 + y ** 2) < 1.0:
acc += 1
return 4.0 * acc / nsamples
Recommend
About Joyk
Aggregate valuable and interesting links.
Joyk means Joy of geeK