
3

LSI算法实现篇
source link: https://ylhao.github.io/2018/05/17/3/
Go to the source link to view the article. You can view the picture content, updated content and better typesetting reading experience. If the link is broken, please click the button below to view the snapshot at that time.
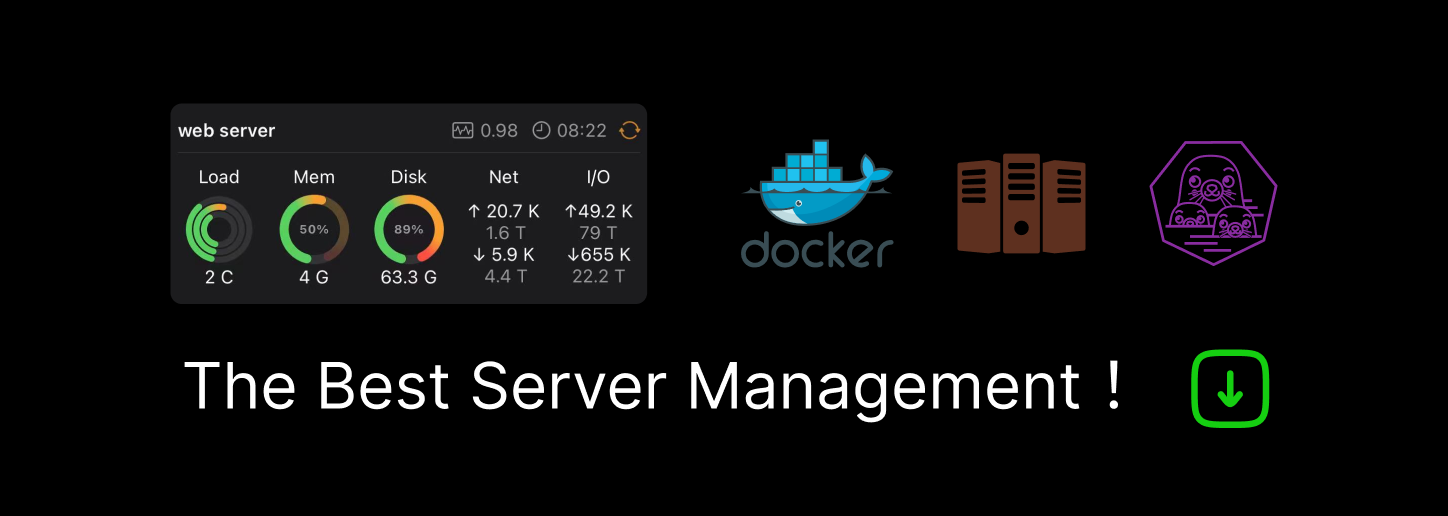
LSI算法实现篇
创建时间:2018-05-17 23:34
字数:1,073
阅读:35
这里主要是用 gensim 这个工具包实现了 LSI 算法,用 LSI 算法做文本相似度分析。程序的执行流程大致如下:
- 过滤(只保留了汉字和英文,去掉了所有的空白字符和标点,英文大写转小写,注意这里没有去停用词)
- 得到词袋模型
- 计算每个文本的 tf-idf 向量
- 基于所有文本的 tf-idf 向量执行 LSI 算法得到所有文本的 LSI 向量(假设主题数为 200)
- 基于 LSI 向量进行文本相似度的计算
# encoding: utf-8
from gensim import corpora, models, similarities, logging
import jieba
import re
import pandas as pd
# 分词
words = []
with open('./train_data.csv', 'r', encoding='utf-8') as f:
next(f)
for line in f:
label, content = line.split(',', 1)
content = ' '.join(list(jieba.cut(content)))
words.append((label, content))
# 过滤
patterns = [re.compile(u'[^\u4E00-\u9FA5A-Za-z]'), # 匹配汉字和英文
re.compile('\s'), # 匹配所有的空格字符
re.compile(' {2,}')] # 匹配 2 个以上空格(包含 2 个)
labels = []
contents = []
for label, content in words:
for pattern in patterns: # 依据正则表达式过滤
content = re.sub(pattern, ' ', content)
content = content.lower()
content = content.strip()
labels.append(label)
contents.append(content)
# 转为 DataFrame
train_data = {
'label': labels,
'content': contents
}
train_df = pd.DataFrame(train_data)
train_df['content'] = train_df['content'].apply(lambda x: x.split())
# check
# print(train_df.shape)
# print(train_df.head(5))
# 得到词典,词袋模型,tfidf 模型,每个文本的 tfidf 向量,lsi 模型,每个文本对应的 lsi 向量,索引
# logging.basicConfig(format='%(asctime)s : %(levelname)s : %(message)s', level=logging.INFO) # gensim 日志
dct = corpora.Dictionary(train_df['content'])
bow = [dct.doc2bow(content) for content in train_df['content']]
tfidf_model = models.TfidfModel(bow)
tfidf_vecs = tfidf_model[bow]
lsi_model = models.LsiModel(tfidf_vecs, id2word=dct, num_topics=200)
lsi_vecs = lsi_model[tfidf_vecs]
indexes = similarities.SparseMatrixSimilarity(lsi_vecs, num_features=200)
# check
querys = [
'日本 制造业 pmi 终值 初值',
'外媒 新加坡 金管局 局长 警告 称 不要 低估 全球 经济 风险',
'连续 跌停 次新股 能科 股份 资金 翘开 跌停板',
'德拉 基 充足 货币政策 宽松',
'英镑 美元 刷新 低 支撑位'
]
for query in querys:
sims = indexes[lsi_model[tfidf_model[dct.doc2bow(query.split(' '))]]]
sims = sorted(enumerate(sims), key=lambda item: -item[1])
print(query)
for index, sim in sims[:3]:
print(' '.join(train_df['content'][index]), ', 相似度为: ', sim)
print()
程序执行结果
日本 制造业 pmi 终值 初值
日本 月 制造业 pmi 终值 初值 , 相似度为: 0.99582595
markit 经济学家 rob dobson 英国 制造业 pmi 对 制造业 状况 的 反映 好坏 参半 , 相似度为: 0.84928674
法国 月 制造业 pmi 初值 预期 前值 , 相似度为: 0.7943848
外媒 新加坡 金管局 局长 警告 称 不要 低估 全球 经济 风险
据 外媒 新加坡 金管局 局长 警告 称 不要 低估 全球 经济 风险 , 相似度为: 0.98838115
世界 银行行长 金墉 全球 经济 处于 前所未见 的 领域 政府 在 财政政策 的 杠杆 已经 被 推到 极致 , 相似度为: 0.38092345
oecd 秘书长 全球 经济 正 非常 缓慢 地 改善 , 相似度为: 0.373093
连续 跌停 次新股 能科 股份 资金 翘开 跌停板
连续 六个 跌停 的 次新股 能科 股份 被 资金 翘开 跌停板 , 相似度为: 0.97551125
顺威 股份 闪崩 跌停 , 相似度为: 0.7192299
次新股 逆势 拉升 邦 宝 益智 读者 传媒 博敏 电子 等 开板 次新股 均 从 大跌 到 强势 涨停 道森 股份 凯龙 股份 等 连续 暴跌 后 拉升 , 相似度为: 0.50892377
德拉 基 充足 货币政策 宽松
德拉 基 充足 的 货币政策 宽松 仍 是 必要 的 , 相似度为: 0.9683843
德拉 基 记者会 欧洲央行 行长 德拉 基 没有 讨论 要 在 指引 中 删掉 qe 通胀 的 关联 , 相似度为: 0.87764835
德拉 基 记者会 欧洲央行 行长 德拉 基 欧洲央行 行长 德拉 基 中期 核心 通胀 将 逐步 上扬 , 相似度为: 0.8646995
英镑 美元 刷新 低 支撑位
英镑 美元 刷新 日低 至 支撑位 在 , 相似度为: 0.89580685
英镑 兑 美元 持续 走高 刷新 逾 一周 高点 , 相似度为: 0.66900176
英镑 美元 涨幅 扩大 至 逾 触及 刷新 日高 , 相似度为: 0.6406657
数据集下载
注意事项:数据仅供个人学习使用,请不要用于商业用途。
[百度网盘,密码:bd8k](https://pan.baidu.com/s/17U8Y8pl9Qwce6LyAt3yktg),
转载请注明来源,欢迎对文章中的引用来源进行考证,欢迎指出任何有错误或不够清晰的表达,可以在文章下方的评论区进行评论,也可以邮件至 [email protected]
Recommend
About Joyk
Aggregate valuable and interesting links.
Joyk means Joy of geeK