
7

Learning garment manipulation policies toward robot-assisted dressing
source link: https://www.science.org/doi/10.1126/scirobotics.abm6010
Go to the source link to view the article. You can view the picture content, updated content and better typesetting reading experience. If the link is broken, please click the button below to view the snapshot at that time.
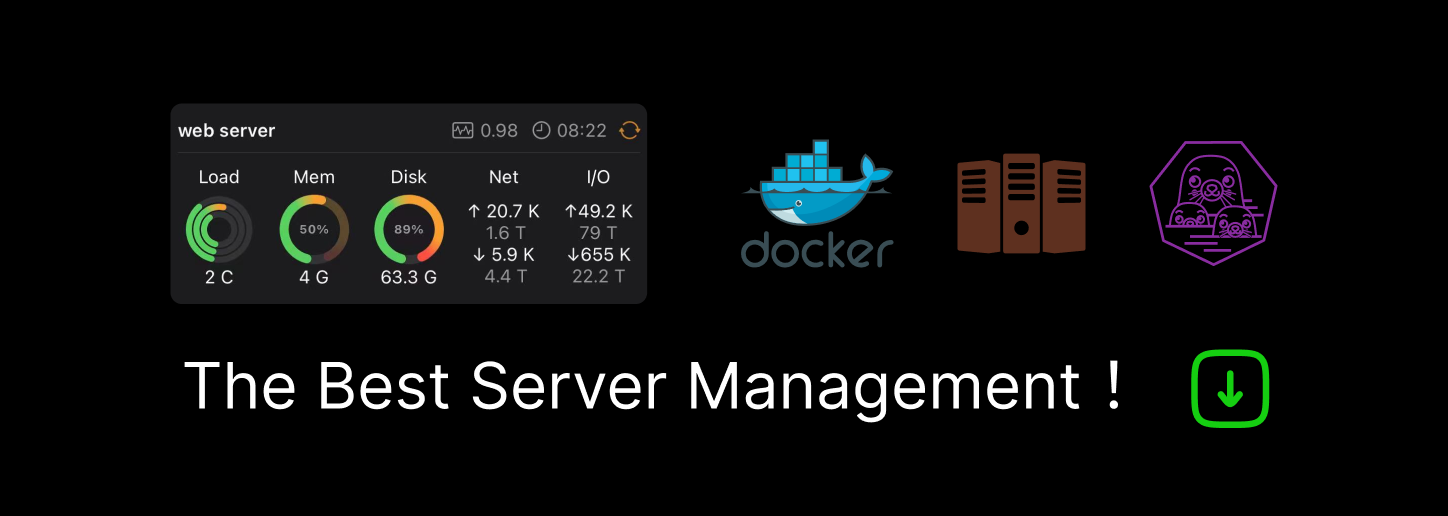
HomeScience RoboticsVol. 7, No. 65Learning garment manipulation policies toward robot-assisted dressing
Research Article
HUMAN-ROBOT INTERACTION
Share on
Learning garment manipulation policies toward robot-assisted dressing
Science Robotics • 6 Apr 2022 • Vol 7, Issue 65 • DOI: 10.1126/scirobotics.abm6010
4871
Metrics
Total downloads487
- Last 6 Months487
- Last 12 Months487
Total citations1
- Last 6 Months1
- Last 12 Months1
Abstract
Assistive robots have the potential to support people with disabilities in a variety of activities of daily living, such as dressing. People who have completely lost their upper limb movement functionality may benefit from robot-assisted dressing, which involves complex deformable garment manipulation. Here, we report a dressing pipeline intended for these people and experimentally validate it on a medical training manikin. The pipeline is composed of the robot grasping a hospital gown hung on a rail, fully unfolding the gown, navigating around a bed, and lifting up the user’s arms in sequence to finally dress the user. To automate this pipeline, we address two fundamental challenges: first, learning manipulation policies to bring the garment from an uncertain state into a configuration that facilitates robust dressing; second, transferring the deformable object manipulation policies learned in simulation to real world to leverage cost-effective data generation. We tackle the first challenge by proposing an active pre-grasp manipulation approach that learns to isolate the garment grasping area before grasping. The approach combines prehensile and nonprehensile actions and thus alleviates grasping-only behavioral uncertainties. For the second challenge, we bridge the sim-to-real gap of deformable object policy transfer by approximating the simulator to real-world garment physics. A contrastive neural network is introduced to compare pairs of real and simulated garment observations, measure their physical similarity, and account for simulator parameters inaccuracies. The proposed method enables a dual-arm robot to put back-opening hospital gowns onto a medical manikin with a success rate of more than 90%.
Get full access to this article
View all available purchase options and get full access to this article.
Already a subscriber or AAAS Member?Log In
Supplementary Materials
This PDF file includes:
Sections S1 to S5
Figs. S1 to S4
Table S1
- Download
- 4.39 MB
REFERENCES AND NOTES
T. L. Mitzner, T. L. Chen, C. C. Kemp, W. A. Rogers, Identifying the potential for robotics to assist older adults in different living environments. Int. J. Soc. Robot. 6, 213–227 (2014).
H. Robinson, B. MacDonald, E. Broadbent, The role of healthcare robots for older people at home: A review. Int. J. Soc. Robot. 6, 575–591 (2014).
B. J. Dudgeon, J. M. Hoffman, M. A. Ciol, A. Shumway-Cook, K. M. Yorkston, Managing activity difficulties at home: A survey of Medicare beneficiaries. Arch. Phys. Med. Rehabil. 89, 1256–1261 (2008).
A. Colomé, A. Planells, C. Torras, A friction-model-based framework for reinforcement learning of robotic tasks in non-rigid environments, in Proceedings of the 2015 IEEE International Conference on Robotics and Automation (ICRA) (IEEE, 2015), pp. 5649–5654.
T. Tamei, T. Matsubara, A. Rai, T. Shibata, Reinforcement learning of clothing assistance with a dual-arm robot, in Proceedings of the 2011 11th IEEE-RAS International Conference on Humanoid Robots (IEEE, 2011), pp. 733–738.
T. Matsubara, D. Shinohara, M. Kidode, Reinforcement learning of a motor skill for wearing a t-shirt using topology coordinates. Adv. Robot. 27, 513–524 (2013).
N. Koganti, T. Tamei, T. Matsubara, T. Shibata, Estimation of human cloth topological relationship using depth sensor for robotic clothing assistance, in Proceedings of Conference on Advances In Robotics (ACM, 2013), pp. 1–6.
N. Koganti, T. Tamei, T. Matsubara, T. Shibata, Real-time estimation of human-cloth topological relationship using depth sensor for robotic clothing assistance, The 23rd IEEE International Symposium on Robot and Human Interactive Communication (IEEE, 2014), pp. 124–129.
N. Koganti, J. G. Ngeo, T. Tomoya, K. Ikeda, T. Shibata, Cloth dynamics modeling in latent spaces and its application to robotic clothing assistance, in Proceedings of the 2015 IEEE/RSJ International Conference on Intelligent Robots and Systems (IROS) (IEEE, 2015), pp. 3464–3469.
Z. Erickson, A. Clegg, W. Yu, G. Turk, C. K. Liu, C. C. Kemp, What does the person feel? learning to infer applied forces during robot-assisted dressing, in Proceedings of the 2017 IEEE International Conference on Robotics and Automation (ICRA) (IEEE, 2017), pp. 6058–6065.
F. Zhang, A. Cully, Y. Demiris, Personalized robot-assisted dressing using user modeling in latent spaces, in Proceedings of the 2017 IEEE/RSJ International Conference on Intelligent Robots and Systems (IROS) (IEEE, 2017), pp. 3603–3610.
Z. Erickson, M. Collier, A. Kapusta, C. C. Kemp, Tracking human pose during robot-assisted dressing using single-axis capacitive proximity sensing. IEEE Robot. Autom. Lett. 3, 2245–2252 (2018).
E. Pignat, S. Calinon, Learning adaptive dressing assistance from human demonstration. Robot. Auton. Syst. 93, 61–75 (2017).
N. Koganti, T. Tamei, K. Ikeda, T. Shibata, Bayesian nonparametric learning of cloth models for real-time state estimation. IEEE Trans. Robot. 33, 916–931 (2017).
Z. Erickson, H. M. Clever, V. Gangaram, G. Turk, C. K. Liu, C. C. Kemp, Multidimensional capacitive sensing for robot-assisted dressing and bathing, in Proceedings os the 2019 IEEE 16th International Conference on Rehabilitation Robotics (ICORR) (IEEE, 2019), pp. 224–231.
A. Kapusta, Z. Erickson, H. M. Clever, W. Yu, C. K. Liu, G. Turk, C. C. Kemp, Personalized collaborative plans for robot-assisted dressing via optimization and simulation. Auton. Robot. 43, 2183–2207 (2019).
Y. Gao, H. J. Chang, Y. Demiris, Iterative path optimisation for personalised dressing assistance using vision and force information, in Proceedings of the 2016 IEEE/RSJ International Conference on Intelligent Robots and Systems (IROS) (IEEE, 2016), pp. 4398–4403.
Y. Gao, H. J. Chang, Y. Demiris, User modelling using multimodal information for personalised dressing assistance. IEEE Access 8, 45700–45714 (2020).
F. Zhang, A. Cully, Y. Demiris, Probabilistic real-time user posture tracking for personalized robot-assisted dressing. IEEE Trans. Robot. 35, 873–888 (2019).
E. Hernandez-Medina, S. Eaton, D. Hurd, A. White, Training Programs for Certified Nursing Assistants (AARP Public Policy Instutute, 2006).
CNA Skill 15 Dress the resident with a paralyzed / contracted arm (2021); www.youtube.com/watch?v=-IkJ5ev3edM&t=263s.
H. Yin, A. Varava, D. Kragic, Modeling, learning, perception, and control methods for deformable object manipulation. Sci. Robot. 6, eabd8803 (2021).
J. Sanchez, J.-A. Corrales, B.-C. Bouzgarrou, Y. Mezouar, Robotic manipulation and sensing of deformable objects in domestic and industrial applications: A survey. Int. J. Robot. Res. 37, 688–716 (2018).
V. E. Arriola-Rios, P. Guler, F. Ficuciello, D. Kragic, B. Siciliano, J. L. Wyatt, Modeling of deformable objects for robotic manipulation: A tutorial and review. Front. Robot. AI 7, 82 (2020).
A. Ganapathi, P. Sundaresan, B. Thananjeyan, A. Balakrishna, D. Seita, J. Grannen, M. Hwang, R. Hoque, J. E. Gonzalez, N. Jamali, K. Yamane, S. Iba, K. Goldberg, Learning to smooth and fold real fabric using dense object descriptors trained on synthetic color images. arXiv:2003.12698 (2020).
E. Corona, G. Alenya, A. Gabas, C. Torras, Active garment recognition and target grasping point detection using deep learning. Pattern Recogn. 74, 629–641 (2018).
Z. Erickson, V. Gangaram, A. Kapusta, C. K. Liu, C. C. Kemp, Assistive gym: A physics simulation framework for assistive robotics, in Proceedings of the 2020 IEEE International Conference on Robotics and Automation (ICRA) (IEEE, 2020), pp. 10169–10176.
F. Zhang, Y. Demiris, Learning grasping points for garment manipulation in robot-assisted dressing, in Proceedings of the 2020 IEEE International Conference on Robotics and Automation (ICRA) (IEEE, 2020), pp. 9114–9120.
A. Doumanoglou, J. Stria, G. Peleka, I. Mariolis, V. Petrik, A. Kargakos, L. Wagner, V. Hlaváč, T.-K. Kim, S. Malassiotis, Folding clothes autonomously: A complete pipeline. IEEE Trans. Robot. 32, 1461–1478 (2016).
D. Seita, N. Jamali, M. Laskey, A. K. Tanwani, R. Berenstein, P. Baskaran, S. Iba, J. Canny, K. Goldberg, Deep transfer learning of pick points on fabric for robot bed-making, International Symposium on Robotics Research (ISRR) (Springer, 2019).
K. Saxena, T. Shibata, Garment recognition and grasping point detection for clothing assistance task using deep learning, in Proceedings of the 2019 IEEE/SICE International Symposium on System Integration (SII) (IEEE, 2019), pp. 632–637.
M. Cusumano-Towner, A. Singh, S. Miller, J. F. O’Brien, P. Abbeel, Bringing clothing into desired configurations with limited perception, in Proceedings of the 2011 IEEE international Conference on Robotics and Automation (IEEE, 2011), pp. 3893–3900.
R. Jangir, G. Alenyà, C. Torras, Dynamic cloth manipulation with deep reinforcement learning, in Proceedings of the 2020 IEEE International Conference on Robotics and Automation (ICRA) (IEEE, 2020), pp. 4630–4636.
Y. Tsurumine, Y. Cui, E. Uchibe, T. Matsubara, Deep reinforcement learning with smooth policy update: Application to robotic cloth manipulation. Robot. Auton. Syst. 112, 72–83 (2019).
A. Zeng, S. Song, S. Welker, J. Lee, A. Rodriguez, T. Funkhouser, Learning synergies between pushing and grasping with self-supervised deep reinforcement learning, in Proceedings of the 2018 IEEE/RSJ International Conference on Intelligent Robots and Systems (IROS) (IEEE, 2018), pp. 4238–4245.
H. Liang, X. Lou, Y. Yang, C. Choi, Learning visual affordances with target-orientated deep q-network to grasp objects by harnessing environmental fixtures, 2021 IEEE International Conference on Robotics and Automation (ICRA) (IEEE, 2021), pp. 2562–2568.
H. Ha, S. Song, Flingbot: The unreasonable effectiveness of dynamic manipulation for cloth unfolding, Conference on Robotic Learning (CoRL) (PMLR, 2021), pp. 24–33.
K. S. Sahari, H. Seki, Y. Kamiya, M. Hikizu, Edge tracing manipulation of clothes based on different gripper types. J. Comput. Sci. 6, 872–879 (2010).
I. Garcia-Camacho, M. Lippi, M. C. Welle, H. Yin, R. Antonova, A. Varava, J. Borras, C. Torras, A. Marino, G. Alenya, D. Kragic, Benchmarking bimanual cloth manipulation. IEEE Robot. Autom. Lett. 5, 1111–1118 (2020).
J. Matas, S. James, A. J. Davison, Sim-to-real reinforcement learning for deformable object manipulation, in Conference on Robot Learning (CoRL) (PMLR, 2018), pp. 734–743.
K. Bousmalis, A. Irpan, P. Wohlhart, Y. Bai, M. Kelcey, M. Kalakrishnan, L. Downs, J. Ibarz, P. Pastor, K. Konolige, S. Levine, V. Vanhoucke, Using simulation and domain adaptation to improve efficiency of deep robotic grasping, in Proceedings of the 2018 IEEE international conference on robotics and automation (ICRA) (IEEE, 2018), pp. 4243–4250.
P. D. Nguyen, T. Fischer, H. J. Chang, U. Pattacini, G. Metta, Y. Demiris, Transferring visuomotor learning from simulation to the real world for robotics manipulation tasks, in Proceedings of the 2018 IEEE/RSJ International Conference on Intelligent Robots and Systems (IROS) (IEEE, 2018), pp. 6667–6674.
F. Sadeghi, S. Levine, Cad2rl: Real single-image flight without a single real image, Proceedings of Robotics: Science and Systems (2017).
K. Rao, C. Harris, A. Irpan, S. Levine, J. Ibarz, M. Khansari, Rl-cyclegan: Reinforcement learning aware simulation-to-real, Proceedings of the IEEE/CVF Conference on Computer Vision and Pattern Recognition (IEEE, 2020), pp. 11157–11166.
J. Hwangbo, J. Lee, A. Dosovitskiy, D. Bellicoso, V. Tsounis, V. Koltun, M. Hutter, Learning agile and dynamic motor skills for legged robots. Sci. Robot. 4, eaau5872 (2019).
F. Golemo, A. A. Taiga, A. Courville, P.-Y. Oudeyer, Sim-to-real transfer with neural-augmented robot simulation, Conference on Robot Learning (CoRL) (PMLR, 2018), pp. 817–828.
P. Christiano, Z. Shah, I. Mordatch, J. Schneider, T. Blackwell, J. Tobin, P. Abbeel, W. Zaremba, Transfer from simulation to real world through learning deep inverse dynamics model. arXiv:1610.03518 [cs.RO] (11 October 2016).
S. Kolev, E. Todorov, Physically consistent state estimation and system identification for contacts, in Proceedings of the 2015 IEEE-RAS 15th International Conference on Humanoid Robots (Humanoids) (IEEE, 2015), pp. 1036–1043.
Z. Xu, J. Wu, A. Zeng, J. B. Tenenbaum, S. Song, Densephysnet: Learning dense physical object representations via multi-step dynamic interactions, Proceedings of Robotics: Science and Systems (2019).
J. K. Li, W. S. Lee, D. Hsu, Push-net: Deep planar pushing for objects with unknown physical properties. Proc. Robot. 14, 1–9 (2018).
A. Zeng, S. Song, J. Lee, A. Rodriguez, T. Funkhouser, Tossingbot: Learning to throw arbitrary objects with residual physics, in Proceedings of the IEEE Transactions on Robotics (IEEE, 2020).
A. Ajay, J. Wu, N. Fazeli, M. Bauza, L. P. Kaelbling, J. B. Tenenbaum, A. Rodriguez, Augmenting physical simulators with stochastic neural networks: Case study of planar pushing and bouncing, in Proceedings of the 2018 IEEE/RSJ International Conference on Intelligent Robots and Systems (IROS) (IEEE, 2018), pp. 3066–3073.
A. Kloss, S. Schaal, J. Bohg, Combining learned and analytical models for predicting action effects from sensory data. Int. J. Robot. Res. , 0278364920954896 (2020).
P. Chang, T. Padif, Sim2real2sim: Bridging the gap between simulation and real-world in flexible object manipulation, in Proceedings of the 2020 Fourth IEEE International Conference on Robotic Computing (IRC) (IEEE, 2020), pp. 56–62.
E. Miguel, D. Bradley, B. Thomaszewski, B. Bickel, W. Matusik, M. A. Otaduy, S. Marschner, Data-driven estimation of cloth simulation models, in Computer Graphics Forum (Wiley Online Library, 2012), vol. 31, pp. 519–528.
H. Wang, J. F. O’Brien, R. Ramamoorthi, Data-driven elastic models for cloth: Modeling and measurement. ACM Trans. Graphics 30, 1–12 (2011).
K. L. Bouman, B. Xiao, P. Battaglia, W. T. Freeman, Estimating the material properties of fabric from video, Proceedings of the IEEE International Conference on Computer Vision (IEEE, 2013), pp. 1984–1991.
Y. Li, Y. Wang, M. Case, S.-F. Chang, P. K. Allen, Real-time pose estimation of deformable objects using a volumetric approach, in Proceedings of the 2014 IEEE/RSJ International Conference on Intelligent Robots and Systems (IEEE, 2014), pp. 1046–1052.
S. Yang, J. Liang, M. C. Lin, Learning-based cloth material recovery from video, in Proceedings of the IEEE International Conference on Computer Vision (IEEE, 2017), pp. 4383–4393.
Y.-L. Qiao, J. Liang, V. Koltun, M. C. Lin, Scalable differentiable physics for learning and control, International Conference on Machine Learning (ICML) (PMLR, 2020).
J. K. Murthy, M. Macklin, F. Golemo, V. Voleti, L. Petrini, M. Weiss, B. Considine, J. Parent-Lévesque, K. Xie, K. Erleben, L. Paull, F. Shkurti, D. Nowrouzezahrai, S. Fidler, gradsim: Differentiable simulation for system identification and visuomotor control, International Conference on Learning Representations (2020).
X. Lin, Y. Wang, J. Olkin, D. Held, Softgym: Benchmarking deep reinforcement learning for deformable object manipulation, Conference on Robot Learning (CoRL) (PMLR, 2020).
Y. Wu, W. Yan, T. Kurutach, L. Pinto, P. Abbeel, Learning to manipulate deformable objects without demonstrations, Proceedings of Robotics: Science and Systems (2020).
R. Hadsell, S. Chopra, Y. LeCun, Dimensionality reduction by learning an invariant mapping, in Proceedings of the 2006 IEEE Computer Society Conference on Computer Vision and Pattern Recognition (CVPR’06) (IEEE, 2006), vol. 2, pp. 1735–1742.
K. He, G. Gkioxari, P. Dollár, R. Girshick, Mask r-cnn, in Proceedings of the IEEE International Conference on Computer Vision (IEEE, 2017), pp. 2961–2969.
P. Khosla, P. Teterwak, C. Wang, A. Sarna, Y. Tian, P. Isola, A. Maschinot, C. Liu, D. Krishnan, Supervised contrastive learning. Adv. Neural Inf. Proces. Syst. 33, 18661–18673 (2020).
L. van der Maaten, G. Hinton, Visualizing data using t-SNE. J. Mach. Learn. Res. 9, 2579–2605 (2008).
J. Cardona, M. Howland, J. Dabiri, Seeing the wind: Visual wind speed prediction with a coupled convolutional and recurrent neural network, in Advances in Neural Information Processing Systems (PMLR, 2019), pp. 8735–8745.
T. F. Runia, K. Gavrilyuk, C. G. Snoek, A. W. Smeulders, Cloth in the wind: A case study of physical measurement through simulation, in Proceedings of the IEEE/CVF Conference on Computer Vision and Pattern Recognition (IEEE, 2020), pp. 10498–10507.
A. Allevato, E. S. Short, M. Pryor, A. Thomaz, Tunenet: One-shot residual tuning for system identification and sim-to-real robot task transfer, in Conference on Robot Learning (CoRL) (PMLR, 2020), pp. 445–455.
A. Medela, A. Picon, Constellation loss: Improving the efficiency of deep metric learning loss functions for the optimal embedding of histopathological images. J. Pathol. Informatics 11, 38 (2020).
M. Lippi, P. Poklukar, M. C. Welle, A. Varava, H. Yin, A. Marino, D. Kragic, Latent space roadmap for visual action planning of deformable and rigid object manipulation, 2020 IEEE/RSJ International Conference on Intelligent Robots and Systems (IROS) (IEEE, 2020), pp. 5619–5626.
W. Yan, A. Vangipuram, P. Abbeel, L. Pinto, Learning predictive representations for deformable objects using contrastive estimation, Conference on Robot Learning (CoRL) (PMLR, 2020).
K. Sun, B. Xiao, D. Liu, J. Wang, Deep high-resolution representation learning for human pose estimation, in Proceedings of the IEEE Conference on Computer Vision and Pattern Recognition (IEEE, 2019), pp. 5693–5703.
A. Veit, S. Belongie, T. Karaletsos, Conditional similarity networks, in Proceedings of the IEEE Conference on Computer Vision and Pattern Recognition (2017), pp. 830–838.
J. Donahue, L. Anne Hendricks, S. Guadarrama, M. Rohrbach, S. Venugopalan, K. Saenko, T. Darrell, Long-term recurrent convolutional networks for visual recognition and description, in Proceedings of the IEEE Conference on Computer Vision and Pattern Recognition (IEEE, 2015), pp. 2625–2634.
S. Hochreiter, J. Schmidhuber, Long short-term memory. Neural Comput. 9, 1735–1780 (1997).
G. Huang, Z. Liu, L. Van Der Maaten, K. Q. Weinberger, Densely connected convolutional networks, in Proceedings of the IEEE Conference on Computer Vision and Pattern Recognition (IEEE, 2017), pp. 4700–4708.
T. Hester, M. Vecerik, O. Pietquin, M. Lanctot, T. Schaul, B. Piot, D. Horgan, J. Quan, A. Sendonaris, I. Osband, J. Agapiou, J. Z. Leibo, A. Gruslys, Deep q-learning from demonstrations, in Proceedings of the AAAI Conference on Artificial Intelligence (PMLR, 2018).
A. Singh, L. Yang, K. Hartikainen, C. Finn, S. Levine, End-to-end robotic reinforcement learning without reward engineering, in Proceedings of Robotics: Science and Systems (2019).
A. Xie, A. Singh, S. Levine, C. Finn, Few-shot goal inference for visuomotor learning and planning, Conference on Robot Learning (CoRL) (PMLR, 2018), pp. 40–52.
C. Heindl, S. Zambal, J. Scharinger, Learning to predict robot keypoints using artificially generated images, in Proceedings of the 2019 24th IEEE International Conference on Emerging Technologies and Factory Automation (ETFA) (IEEE, 2019), pp. 1536–1539.
G. Brockman, V. Cheung, L. Pettersson, J. Schneider, J. Schulman, J. Tang, W. Zaremba, Openai gym. arXiv:1606.01540 [cs.LG] (5 June 2016).
Recommend
About Joyk
Aggregate valuable and interesting links.
Joyk means Joy of geeK