

Geographic variation in and contextual factors related to biguanide adherence am...
source link: https://www.sciencedirect.com/science/article/pii/S2352827321002883
Go to the source link to view the article. You can view the picture content, updated content and better typesetting reading experience. If the link is broken, please click the button below to view the snapshot at that time.
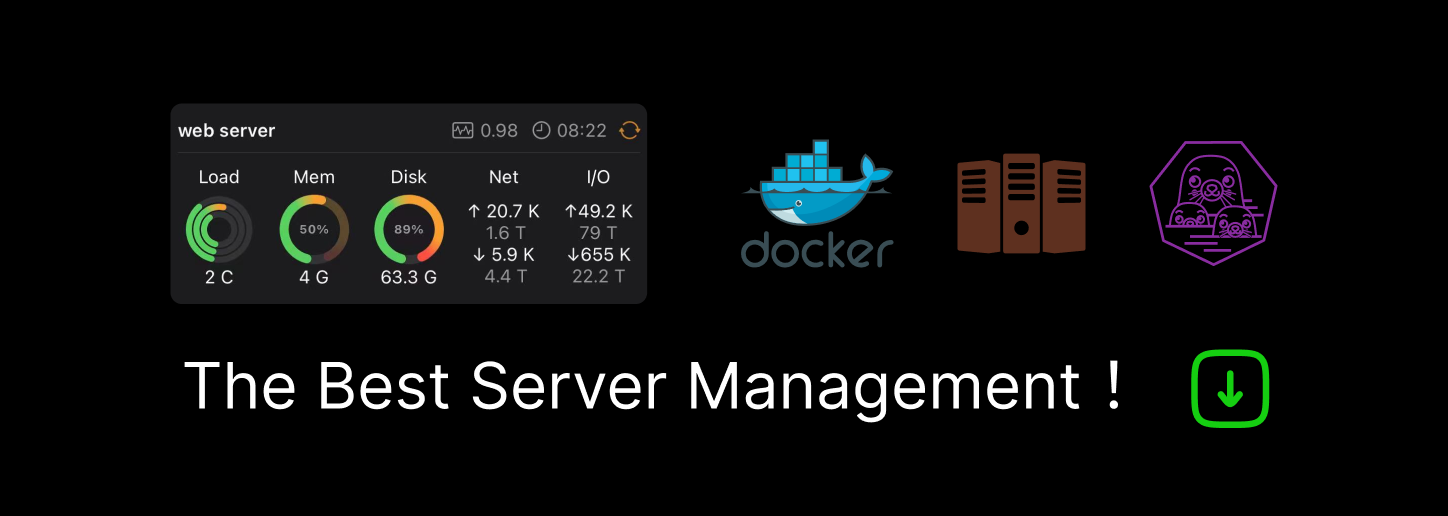
Geographic variation in and contextual factors related to biguanide adherence amongst medicaid enrolees with type 2 Diabetes Mellitus
Highlights
Medication adherence is a problem amongst Type 2 Diabetes patients on Medicaid.
•Social and contextual factors' influence on medication adherence is underexplored.
•Higher social vulnerability is associated with non-adherence to biguanides.
•Adopting spatial analysis techniques enables geographic targeting of health risk.
Abstract
Much is known about the adverse impacts on diabetes outcomes of non-adherence to diabetes medication. Less is known about how adherence to diabetes medication varies geographically, and the correspondence of this variation to social and contextual factors. Using pharmacy claims data over a two-year period, this study analysed non-adherence to biguanide medication for N=24,387 adult Medicaid enrolees diagnosed with Type 2 Diabetes Mellitus (T2DM) and residing in Ohio. Spatial analysis was used to detect clusters of census tract level rates of non-adherence, defined as the proportion of patients below the Proportion Days Covered (PDC) threshold of 80%, the level at which patients have a reasonable likelihood of achieving most clinical benefit from their medication. Multilevel models were used to understand associations between medication non-adherence and contextual factors including social vulnerability, urbanicity and distance to utilised pharmacy, with adjustment for individual-level covariates. These findings indicate that contextual factors are associated with medication non-adherence in Medicaid clients with T2DM. They suggest a need for spatially specific, multifaceted intervention programmes that target and/or account for the features of residential settings beyond individual and health system-level factors alone. While “environmental” considerations are often acknowledged, few intervention initiatives are predicated on explicit knowledge of spatially variable influences that can be targeted to enable and support medication adherence.
Keywords
1. Introduction
Adherence to a prescribed medication regimen is critical for the maintenance of health and quality of life amongst patients with Type 2 Diabetes Mellitus (T2DM) (Krass, Schieback, & Dhippayom, 2015). Medication adherence is a key self-care behaviour that, along with physical activity, healthful diet, blood glucose monitoring, foot care and ophthalmological check-ups, is needed to effectively manage T2DM in the long-term (Sabate, 2003). Given the global burden of T2DM, and projected growth of this condition in the U.S. alone (J. Lin et al., 2018; X. Lin et al., 2020), medication non-adherence in persons with T2DM requires urgent attention.
A systematic review of adherence to oral hypoglycaemic agents found wide variation in rates ranging between 36% and 93% (Cramer, 2004). Not taking medication as prescribed can lead to a range of adverse health effects in those with T2DM including worsening of disease, increased hospitalisations, and greater risk of death (Ho et al., 2006). Improving medication adherence is necessary to ensure T2DM patients realise the full benefit of prescribed therapies including glycaemic control and significant reductions in risk of microvascular complications and myocardial infarction (Krass et al., 2015). Further benefits include improved health system efficiency related to hospital utilisation and economic cost (Pednekar, Heller, & Peterson, 2020), with one U.S. study estimating almost 25 billion dollars per year in avoidable healthcare costs due to diabetes (Morello & Hirsch, 2017).
Medication adherence is recognised as a complex health-related behaviour influenced by multiple factors. A patient's ability to manage his or her behaviour, achieve tight metabolic control and prevent the long-term complications of diabetes is determined by a host of intra-personal, inter-personal and environmental factors (Sabate, 2003). To act on modifiable factors that lead to medication non-adherence, one line of research enquiry has proposed a theory-driven framework that integrates evidence-based behavioural change techniques (Tesfaye et al., 2021). Social influences and environmental context and resources are key domains in this theoretical framework. In the medication adherence literature to date however, research into these domains remains largely focused on patients and health provider level factors in healthcare settings, with less attention being paid to broader social and contextual determinants of health (Wilder et al., 2021). This circumstance is perpetuated despite past clinical research acknowledging that a failure to consider factors relevant to patients, such as low health literacy, nutritional deprivation, and inability to afford medications, may constitute a ‘contextual error’ resulting in poorer health outcomes (Weiner et al., 2010).
Disadvantaging social-contextual factors have been linked with disease progression in persons with T2DM, including worsened glycaemic control, cholesterol, and blood pressure (Walker, Smalls, Campbell, Strom Williams, & Egede, 2014), and medication adherence is implicated as a primary mechanism by which social-contextual factors shape disease outcomes. One example of social-contextual factors related to health is a U.S. study on perceived neighbourhood safety and medication non-adherence amongst adults with T2DM. Relative to respondents residing in safe neighbourhoods, a higher proportion of respondents in unsafe neighbourhoods reported having had to delay filling a prescription for any reason, and due to cost (Billimek & Sorkin, 2012b). Another study of an urban adult Medicaid population with T2DM found, however, that distance to dispensing pharmacies and prescribers was not associated with adherence to angiotensin-converting enzyme inhibitors (ACEIs) or angiotensin II receptor blockers (ARBs) (Syed et al., 2016). A recent systematic review and metanalysis concluded that adverse social-contextual factors, including housing instability, food insecurity and social determinants overall, can adversely impact medication adherence, however, the review highlighted the limited number of research studies examining social determinants of medication adherence (Wilder et al., 2021).
Knowledge about how medication adherence varies across geographic regions is extremely limited (Han et al., 2020). Studies that have examined geographic variation in medication adherence have adopted a variety of scales (U.S. regions, counties, ZIP codes, census tracts) for a variety of chronic health conditions (Couto et al., 2014; Erickson & Yuan-Nung, 2014; Han et al., 2020; Hoang, Kolenic, Kline-Rogers, Eagle, & Erickson, 2011; Pizzonia et al., 2019; Tan et al., 2017) making valid comparisons of relationships difficult due to issues including the modifiable areal unit problem (Openshaw, 1984). An early study of geographic variation demonstrated the feasibility of hot spot analysis to identify census tract-level clusters of medication non-adherence among Acute Coronary Syndrome patients (Hoang et al., 2011). It has since been proposed that identifying areas of high and low medication adherence may lead to identifying shared facilitators or barriers to adherence that may be locally relevant and thus potential targets for intervention (Erickson & Yuan-Nung, 2014).
Recent studies have not only described spatial variation in non-adherence but also evaluated associations with local barriers to medication adherence (Han et al., 2020; Hu, Warren, & Exeter, 2019; Tan et al., 2017). The study by Han and colleagues, identified geographic variation in adherence to antihypertensive drugs amongst elderly patients with chronic kidney disease across counties of the United States. Medically underserved areas, areas experiencing high levels of deprivation and those defined as “low income” but not receiving a low-income subsidy had higher levels of non-adherence to medication (Han et al., 2020). Additional research is needed to achieve an improved evidence base to support the delivery of population-level interventions tailored to the local features of residents and their contextual milieu.
This study aimed (1) to examine over a two-year period the associations between social-contextual factors including social vulnerability, urbanicity and distance to utilised pharmacy, and non-adherence to biguanide medication amongst adults with T2DM enrolled in Medicaid in Ohio, and (2) to examine the extent to which rates of non-adherence to biguanide medication were spatially clustered at the census tract level. Two hypotheses were tested; Firstly, that adverse residential-area social-contextual factors would be associated with non-adherence to medication used to treat T2DM. Secondly, that non-adherence to medication among patients would cluster geographically in the form of groupings of areas with high rates of non-adherence (hot spots) and/or low rates of non-adherence (cold spots).
2. Methodology
2.1. Population and setting
The setting for this study was the state of Ohio and the population of interest was all adult residents eligible for and enrolled in Medicaid with Ohio's largest health insurance provider and having a T2DM diagnosis in the past 5 years (n = 101,982, see Fig. 1).

Fig. 1. Derivation of analytic sample of Medicaid enrolees
1 Nelson, Zeng, Kilbourne, Powell, and Moore (2011) (Nelson et al., 2011).
2.2. Study design
The design was cross-sectional with the period of observation from January 1, 2017, to December 31, 2018. The observation period was selected based on recency and a review indicating consistent data quality. The pharmacy claims database was used to calculate medication adherence to the biguanide class of medications for Medicaid members with T2DM across the study period. Fig. 1 illustrates how the analytic sample was derived. De-identified Medicaid recipient claim data provided by the data custodian included member ZIP+4® location information. The ZIP+4® Code uses the basic five-digit zip code plus four additional digits to identify a geographic segment within the five-digit delivery area (e.g., city block, a group of apartments, an individual high-volume receiver of mail, a post office box, or a specific delivery route). For spatial analysis, members were linked to census tract geometry based on whether their ZIP+4® was located within the boundaries of an Ohio census tract. Census tracts with a sample of less than 10 Medicaid recipients were suppressed to support the confidentiality of Medicaid members with T2DM.
2.3. Outcome measure
2.3.1. Medication adherence
In most international guidelines on the management of hyperglycaemia, the biguanide drug class (which includes metformin) is the recommended first-line glucose-lowering agent for T2DM (Zaccardi, Khunti, Marx, & Davies, 2020). Given this precedent, along with rising patient preference and demand for oral medication as opposed to injections in T2DM therapy (Brietzke, 2015), an annual measure of medication adherence to biguanides was developed. Medication adherence was calculated for Medicaid recipients using Proportion Days Covered (PDC) based on prescription refill data available in the pharmacy claims database. This measure provides an indication of adherence to a prescribed medication therapy regimen over a set period and for this study an annual measure (calendar year) was used. Member inclusion criteria for the development of the PDC measure are detailed in Fig. 1. PDC was defined as being equal to the total days that each medication prescription was intended to cover (days’ supply), based on the date that the first prescription of the calendar year was filled (fill date) as a proportion of the sum of days between the first prescription fill date and the end of the calendar year.
For patients with a PDC calculated for both years of the evaluation period, a mean value was calculated. Potential overlap of prescriptions over time (start and end dates) were accounted for in this calculation. For example, if a Medicaid recipient filled their prescription three days before the expiry of their existing prescription, three days were added to ensure the number of days covered was correctly attributed across the calendar year. The Python and Structured Query Language code used for the development of PDC is available upon request from the authors. Per standard practice, a PDC threshold ≥0.8 indicating a minimum of 80% of days covered was used to define “good” medication adherence and thus a reasonable likelihood of achieving the greatest clinical benefit from medication (Pharmacy Quality Alliance, 2021). For this study, a patient was considered non-adherent when their PDC was below the 80% threshold.
For statistical models, a binary outcome variable indicating non-adherence to oral biguanide medication or adherent to oral biguanide (non-adherent=1, adherent=0) was assigned to each member. For spatial analysis, the mean proportion adherent was expressed for each census tract. The crude rate was indirectly age standardised prior to mapping and undertaking hot spot analysis.
Two publicly available census tract level variables were linked to Medicaid recipient data, based on spatial location.
2.4. Area level exposures
Social Vulnerability The U.S. Centers for Disease Control and Prevention (CDC)/Agency for Toxic Substances and Disease Registry (ATSDR) Social Vulnerability Index (SVI), calculated from U.S. Census data, a measure of the social vulnerability of census tracts, was used here (Flanagan, Hallisey, Adams, & Lavery, 2018). The SVI ranks each tract on 15 social factors into four related themes: socioeconomic status, household composition and disability, minority status and language, and housing and transportation. Examples of variables included are poverty, single parent households, English language proficiency and vehicle access. This index has been primarily used for planning for and responding to natural disasters (Flanagan, Gregory, Hallisey, Heitgerd, & Lewis, 2011); however, it also has been recognised as a way to understand social conditions that can impact health behaviours including physical activity (Gay, Robb, Benson, & White, 2016). The 2018 version of the SVI was used for this study, with the specific variable representing the overall percentile ranking of vulnerability across all four themes for each census tract in Ohio.
UrbanicityRural-urban commuting area (RUCA) codes classify U.S. census tracts using measures of population density, urbanisation, and daily commuting (USDA Economic Research Service, 2021). These codes use data from the 2010 decennial census and the 2006-10 American Community Survey. Based on guidelines, a consolidation scheme was adopted (Scheme 1) that used both primary and secondary commuting patterns to incorporate the concept of potential access to resources and services (Washington State Department of Health, 2016). The categories are urban core, suburban, large rural and small town/rural. A conversion table whereby RUCA codes are assigned to these categories and their definitions is provided in the Supplement (Table A).
2.4.1. Distance to utilised pharmacy
Prescription claim data were linked via their National Provider Identifier (NPI) to a purchased database with attributes including the health provider taxonomy classification and geocoded street address (CarePrecise LLC, 2020). This enabled a file to be developed based on pharmacy type (primarily retail and community pharmacy) and frequency of claims per member (see Supplement: Table B for frequency of members by pharmacy type). The distance to utilised pharmacy was calculated as the nearest point-to-point road network distance measured in kilometres from each Medicaid recipient's ZIP+4® Code location to the pharmacy for which the member had obtained the most frequent number of prescriptions and refills.
2.5. Individual-level covariates
Individual-level measures available in the claims data included member year of birth which was converted to age in years for the beginning of the evaluation period. Gender was coded; however, ethnicity was unavailable. A measure of comorbidity was used to indicate whether a member, in addition to T2DM, had a diagnostic claim for hypertension, coronary heart disease or congestive heart failure based on ICD10 codes or the equivalent ICD9. The final variable was converted to a categorical variable indicating 0, 1, 2 or 3 comorbidities.
2.6. Statistical analysis
Given the individual and contextual information available, multilevel logistic regression models were used to estimate the likelihood of an individual being non-adherent to their biguanide medication (1 = non-adherent, 0 = adherent). The Intraclass Correlation Coefficient (ICC) was used to estimate the homogeneity of non-adherence within census tracts. The ICC represents the between census tract variation in non-adherence as a proportion of total variation consisting of between plus within census tract variation in non-adherence). The Akaike Information Criterion (AIC) and Bayesian Information Criterion (BIC) were used to compare model fit. Statistical analysis was performed using Stata version 16 (StataCorp., 2019).
2.7. Spatial analysis
To investigate spatial clustering in age-adjusted rates of non-adherence across the Ohio study region, a global test of spatial autocorrelation, Global Moran's I, was calculated (Cliff & Ord, 1981; Moran, 1950). The inverse distance weighting method using Euclidean distance was applied ensuring each census tract centroid had at least one neighbour. Row standardisation was applied to the spatial weights' matrix. The Global Moran's I value can range from −1 indicating dissimilar values clustering together, to +1 indicating similar values clustering together, with a value of 0 indicating complete spatial randomness.
To assess the occurrence of local clusters of medication non-adherence among neighbouring census tracts, the local Getis-Ord Gi* statistic was calculated (Getis & Ord, 1992). This approach identifies statistically significant spatial clusters of high non-adherence (hot spots) and low non-adherence (cold spots). The Optimised Hot Spot Analysis tool adopted corrects for multiple testing and spatial dependence using the False Discovery Rate correction (Chen, Feng, & Yi, 2017; ESRI, 2021b). The hot spot analysis was also undertaken for the SVI measure whereby clusters of high and low social vulnerability were identified. Hot spots (high non-adherence/high vulnerability) and cold spots (low non-adherence/low vulnerability) were mapped to assess spatial convergence. Statistical significance was indicated by a p value ≤ 0.05. All spatial analysis and map development were undertaken in ArcGIS Pro software version 2.6.0. (ESRI, 2021a).
3. Results
A statistical description of the adult sample is provided in Table 1. Greater proportions of adults with T2DM enrolled in Medicaid during the two-year evaluation period were female, aged between 50 and 64 years and having at least one comorbid condition of which hypertension was the most common (comorbid conditions not shown). The sample was concentrated in urban core areas of the state, defined as built up areas of 50,000 people or more although the sample included individuals located in all four of the RUCA classifications. The highest proportion of the sample was in areas having the greatest social vulnerability. Distance to the most frequently utilised pharmacy was highly skewed, with a median of 3.69 km (0–435).
Table 1. Type 2 Diabetes-diagnosed Medicaid sample by percentage of non-adherence to biguanide medication.
% non-adherentp valueGenderMale9235 (37.9)50.80.036Female15,152 (62.1)52.2Age category (years)18–342269 (9.3)71.7<0.00135–498766 (36.0)58.350–6412,589 (51.6)44.2≥65763 (3.1)40.5Comorbidities (count)03419 (14.0)64.2<0.001115,352 (63.0)50.624141 (17.0)46.831475 (6.1)48.5RUCA classificationUrban core17,550 (72.0)53.5<0.001Suburban1507 (6.2)48.5Large rural4084 (16.8)46.7Small town/rural1246 (5.1)46.2Social vulnerability (quintile)Q1 (Least)700 (2.9)42.9<0.001Q22119 (8.7)48.8Q33908 (16.0)48.5Q47022 (28.8)50.8Q5 (Greatest)10,638 (43.6)54.6<12743 (11.3)50.6Distance to utilised1–2.97617 (31.2)52.2pharmacy (km)3–4.94462 (17.9)52.5<0.2565–9.94312 (17.7)51.9>105353 (22.0)50.7Total24,38751.7
The mean PDC value in the sample was 0.721 (SD = 0.246). Individuals with a PDC less than 0.8 classified as non-adherent to biguanide medication accounted for 51.7% (12,608/24,387) of the sample. Table 1 indicates a higher percentage of non-adherence to biguanides among women, those aged 18–34 years, and those without any of the specified comorbid conditions. A higher percentage of those living in the urban core and in areas of greatest social vulnerability were non-adherent to biguanide medication.
Table 2 presents four models used for estimating the likelihood of non-adherence to biguanide medication for two-level random intercept multilevel logistic models developed for N=24,387 individual Medicaid members diagnosed with T2DM and residing in 1276 census tracts in Ohio. The number of individuals per tract ranged from 10 to 71 (x‾ = 23.4, sd = 11.2). For the null model, the correlation of non-adherence amongst individuals within the same census tract was shown not to be negligible (ICC=0.0224). The ICC increased when individual-level covariates were added to the model (Model 1) but reduced when contextual level variables were added. Model selection statistics (AIC, BIC) indicated Model 2 (individual covariates & contextual variables) was the best fit to the data compared to Model 3 (individual covariates, contextual variables & distance to utilised pharmacy) and Model 1 (individual covariates only)), Accounting for individual-level variables, the contextual factors of rural-urban classification and social vulnerability were significantly associated with non-adherence (p ≤ 0.05). For Model 2, compared to those living in the urban core, those living in small town/rural tracts were 25% less likely to be non-adherent to their biguanide medication. Conversely, those living in census tracts classified as having the greatest level of social vulnerability were 66% more likely to be non-adherent. A test for linear trend following Model 2 indicated a statistically significant positive trend between social vulnerability and non-adherence to biguanide medication (p ≤ 0.05). The distance to the most frequently utilised pharmacy was not found to be associated with non-adherence (Model 3). Transforming or categorising this variable had no effect on its association with non-adherence.
Table 2. Multilevel logistic regression models estimating odds of non-adherence to biguanide medication amongst Type 2 Diabetes patients enrolled in Medicaid over two years (2017–2018).
*p value < 0.05, **p value < 0.01 OR Odds Ratio, CI Confidence Interval.
aCount of comorbid conditions including hypertension, coronary heart disease and coronary heart failure.
bRUCA Rural-Urban Commuting Area codes, ICC intra-level correlation coefficient, AIC Akaike Information Criterion, BIC Bayesian Information Criteria.
4. Results from spatial analysis
For the evaluation period, the total number of census tracts analysed was 1276 (each tract contained a minimum of 10 individuals enrolled in Medicaid and diagnosed with (T2DM). Indirect age-standardised rates (ASR) of non-adherence across the 1276 census tracts ranged from 0 to 0.978 with a mean ASR of 51.3 (sd 0.14). Fig. 2 shows a weak positive relationship between census tract level non-adherence and social vulnerability (Pearson's r = 0.21). These rates were mapped for the entire sample across the State of Ohio. Fig. 3 shows the rates for two major cities and their surrounding regions; Cleveland and Cincinnati, with boundaries indicating census tracts included in the sample. For comparison the percentile ranks were converted to quintiles of social vunerability and are shown in Fig. 2.

Fig. 2. Relationship between biguanide non-adherence and social vulnerability (Pearson Correlation Coefficient = 0.21). For legend see Fig. 3 Non-Adherence.

Fig. 3. Census tract level non-adherence and social vulnerability:
Top Left: Age Standardised Rate (ASR) of Non-adherence (Cleveland Region),
Top Right: Social Vulnerability Index ranking (Cleveland Region),
Bottom Left: Age Standardised Rate of Non-adherence (Cincinnati Region), Bottom Right: Social Vulnerability percentile ranking (Cincinnati Region).
The Global Moran's I test of spatial autocorrelation was positive and statistically signifcant indicating the clustering of census tracts with high rates of non-adherence (Morans I = 11.89, Z=13.02, p < 0.000). The local test of spatial autocorrelation using Getis-Ord Gi* statistic revealed hot and cold spots of non-adherence. A map showing hot and cold spots of non-adherence across the State of Ohio is shown in Fig. 3, with more detailed hot spot maps of Cincinnati and Cleveland provided in the Supplement (Figure A). A total of 469 statistically significant hot spots and 73 cold spots (95% Confidence) were identified based on a false discovery correction for multiple testing and spatial dependence. Many of the census tracts in the sample located in the cities of Columbus, Cleveland and Cincinnati were considered hot spots of non-adherence to biguanide medication (see Fig. 4).
A hot spot analysis of the social vunerability index for all 1276 census tracts in the sample revealed 43 statistically significant hot spots and 100 cold spots (95% Confidence) in analyses including a false discovery correction for multiple testing and accounting for spatial dependence. A map showing hot and cold spots of social vulnerability across Ohio is given in Fig. 5. Hot spots of social vulnerability were prominent in the urban centers of Cleveland, Toledo and Cincinnati with concentrations of cold spots in the north east around Youngstown and in the south-west of the State around Clermont County. A more detailed hot spot map of Cincinnati and Cleveland is provided in the Supplement (Figure A). Fig. 6 indicates spatial convergence of hot and cold spots of non-adherence and social vulnerability. In total, 241 census tracts were classifed as hot spots and 6 as cold spots (95% confidence). Two contiguous areas of spatial convergence, one in greater Cleveland and one in greater Cincinnati made up 97% of all census tract hot spots identified. Four of the six cold spots were identified within Miami County, Ohio.

Fig. 4. Hot and Cold Spot Analysis: Age Standardised Rate (ASR) of non-adherence to biguanides. See detailed hot/cold spot maps of Cleveland and Cincinnati regions in Supplement Figure A.

Fig. 5. Hot and Cold Spot Analysis: Social Vulnerability. See detailed hot/cold spot maps of Cleveland and Cincinnati regions in Appendix map A.

Fig. 6. Spatial convergence of social vulnerability and non-adherence to biguanides. See detailed spatial convergence maps of Cleveland and Cincinnati regions in Supplement Figure B.
5. Discussion
Despite its significant implications for health and wellbeing, medication adherence is an often-overlooked part of quality improvement in the clinical setting (Franklin, Abel, & Shojania, 2020). One reason for limited research into the contextual influences of medication adherence to date is the challenge of accessing and linking detailed clinical information including medical history of patients with broader social and environmental data not usually collected within the confines of a health clinic. More recently however, the increasing feasibility of linking large amounts of detailed health information with publicly available information on the characteristics of the local conditions in which people conduct their daily lives has allowed for a greater insight into potential social-contextual factors related to medication adherence.
This study confirmed that social-contextual factors including social vulnerability and urbanicity were associated with a greater likelihood of non-adherence (PDC ≤0.8) to biguanide medication amongst a sample of adult T2DM patients enrolled in Medicaid. The second hypothesis of geographic clustering of high rates of non-adherence to biguanide medication was also confirmed. A recent systematic review and meta-analysis of 29 studies reported that social determinants of health were associated with medication adherence, but only six of these specifically involved samples of T2DM patients (Wilder et al., 2021). Comparisons of these studies can be difficult due to the different measurement of medication adherence (self-report measures vs measures based on pharmacy dispensing data) and the different social-contextual factors examined. Nevertheless, studies of T2DM patients do suggest that health literacy, food insecurity and housing insecurity influence medication adherence (Billimek & Sorkin, 2012a; Fan, Lyons, Goodman, Blanchard, & Kaphingst, 2016; Sattler, Lee, & Bhargava, 2014; Silverman et al., 2015).
The finding that T2DM patients living in areas with greater social vulnerability were more likely to be non-adherent after adjustment for individual factors is supported by a recent study of patients undergoing statin therapy, where associations were observed between lower levels of adherence and lower neighbourhood socioeconomic status and urban-versus rural-dwelling residents (Erickson, Bravo, & Tootoo, 2020). This is the first time to our knowledge that the CDC/ATSDR Social Vulnerability Index has been used to study potential social determinants of medication adherence. This index has several advantages. Firstly, it covers the entire United States at a reasonably fine geographic scale (census tract) enabling sub-state and sub-county level comparisons. Secondly, it is publicly available for researchers and practitioners to apply. Thirdly, while the CDC/ATSDR SVI was developed to help public health officials and local planners to better prepare for and respond to emergency events including disease outbreaks and natural disasters (Flanagan et al., 2011, 2018), our study supports the use of this index to identify locations that may be at higher risk of non-adherence to medication, a key T2DM self-care behaviour. Other studies have also reported that it may be useful for understanding other diabetes self-care behaviours including physical activity and nutrition (Gay et al., 2016; Yu, Woo, Emrich, & Wang, 2020). Our research contribution validates a valuable public resource in the context of a significant health issue that has rarely been considered from a social and contextual viewpoint, as opposed to a medical, individual level perspective. Furthermore, it demonstrates that spatial convergence can be used as a tool to highlight where non-adherence and social vulnerability overlap in space, advancing on previous spatial targeting efforts to tailor interventions (Pizzonia et al., 2019). The CDC/ATSDR SVI is comprised of variables covering themes of socioeconomic, household and disability, minority status and language, and housing and transportation, hence the expression of high social vulnerability represents a wide scope of the community who are at risk of non-adherence that may not be considered otherwise. This study did not seek to decompose the index further to determine with specificity which social-contextual factors may be particularly relevant to medication adherence. An opportunity for important research exists in this emerging area.
The importance of our finding that rural areas beyond the urban core had lower odds of non-adherence are twofold. Firstly, this recognition supports the allocation of resources toward other important issues in rural areas, such as improving access to specialist care and community support for those with T2DM (Egede et al., 2011). Secondly, it can support targeting of disease within Cleveland and Cincinnati with medication therapy management services shown to be effective in improving medication adherence and intermediary chronic disease outcomes (Rodis et al., 2017; Viswanathan et al., 2015).
No association was found for distance to most frequently utilised pharmacy in this study. This finding aligns with two other studies, one involving n=6532 T2DM patients enrolled in Medicaid in the Greater Chicago region, which focused on adherence to angiotensin-converting enzyme inhibitors and angiotensin II receptor blockers (Syed et al., 2016), and another which focused on n = 174,146 persons enrolled in commercial insurance products in Michigan taking statin medication (Erickson et al., 2020). Walgreens®, a nationwide U.S. pharmacy chain, claims that 76 percent of the entire U.S. population is within 5 miles of a Walgreens® pharmacy, and 40 percent of Medically Underserved Areas are within a 15-min drive-time of a Walgreens® pharmacy (Taitel, 2019). If this is correct, then distance and geographic access to medication may not be a critical factor in influencing adherence, however, access to and cost of transport remain possible barriers. We note that lack of vehicle access is incorporated into the CDC/ATSDR SVI and thus may be reflected in our overall findings with social vulnerability. Another study identified lack of vehicle access as a risk factor for non-adherence (Hensley et al., 2018). Evidence also exists that tailored community pharmacy-based interventions designed to improve medication adherence are effective (Presley, Groot, & Pavlova, 2019), and thus expansion and spatial targeting of these services to pharmacies that are spatially proximal to socially vulnerable populations may be effective in improving medication adherence.
Non-adherent patients with T2DM were found to be younger (18–34 years) and have fewer comorbidities, as would be expected at a younger age. Early onset diabetes occurs in high risk individuals having high needs for effective management and support (Wilmot & Idris, 2014). This represents significant individual and health system costs. The majority of individuals with early onset diabetes are obese (Wilmot & Idris, 2014). Our findings reflect the social gradient of health and support addressing social vulnerability to improve medication adherence in addition to weight-management, diet and physical activity.
Most studies exploring medication adherence do not adopt geospatial techniques (Hu et al., 2019). This research applied geospatial techniques to explore small area geographic variation, spatial clustering, and spatial convergence in medication adherence. Importantly, this enabled an examination of where concentrated populations at high risk of non-adherence exist. Of studies that have undertaken a spatial hotspot analysis of medication adherence, few have adopted a scale as fine as the census tract (as done here) due to challenges including small sample sizes (Hoang et al., 2011). Caution is needed when comparing studies which use different geographic scales of analysis (Fotheringham & Wong, 1991). Researchers must carefully consider the advantages and disadvantages of adopting a particular geographic level unit of analysis as well as issues of privacy (Grubesic & Matisziw, 2006; Kilgore, Teigland, & Pulungan, 2018). Limitations of this study have been identified. Firstly, it is possible that patients who have experienced a longer duration since diagnosis of T2DM may have better medication adherence, although one review did not support this (Krass et al., 2015). This issue was not investigated here as a patient's first diagnosis may have been prior to the earliest year of access to the diagnostic claims data available for this study. In the absence of information on the duration of disease, a criterion was adopted for calculating PDC for each patient based on a diagnostic claim of T2DM in the current or previous year for which PDC was calculated. Secondly, our measurement of medication adherence was derived from pharmacy prescription claims data reflecting medication refills but not medication use. Non-adherence behaviour to prescribed medication may take a number of forms and may not invariably be a deliberate action (Sabate, 2003). The experience of side effects is one potential reason for choosing not to maintain a medication regimen but was not captured in the claims database used for this study. Digestive disorders are common and may cause one patient in 20 to discontinue metformin treatment (Bouchoucha, Uzzan, & Cohen, 2011), thus patients in our study may have ceased taking Metformin or changed to a different medication for this reason. Thirdly, our measure of medication adherence focused on one class of oral medication, biguanides, of which metformin is currently the only medication available. In most international guidelines on the management of hyperglycaemia, metformin is the recommended first-line glucose-lowering agent in patients with T2DM (Zaccardi et al., 2020). However, patients with more complex medication regimens may have difficulty adhering to their medication instructions (Pantuzza, Ceccato, Silveira, Junqueira, & Reis, 2017). This research did not consider adherence to multiple drug classes or medications and excluded claims for insulin injection (due to the complexity of determining the physician's recommended dosing regimen (Cooper, Hall, Penland, Krueger, & May 2009)). By focusing on one primary first line drug commonly taken once per day, this study aimed to provide an indicator of the extent to which medication non-adherence is a problem amongst Medicaid enrolees with T2DM in the State of Ohio. We acknowledge each state operates its own Medicaid program within federal guidelines, thus, caution is recommended when making state-level comparisons due to variations in state Medicaid eligibility. Fourthly, we are unable to confirm our assumption that our sample contained only Medicaid enrolees living independently in the community as opposed to residing in a nursing facility or other treatment centre. This poses a risk of bias to our findings, however, those aged 65 or older made up only 2.5% of our sample and those patients who frequently filled their prescription from a long-term care pharmacy represented only 0.4 percent compared to 95 percent via retail pharmacies (Supplement Table B). Finally, our study design is cross-sectional with limited ability to account for temporal trends in medication adherence or to attribute causality.
6. Conclusion
The findings from this study contribute to an evidence-based framework that links social-contextual factors to medication adherence and supports their further investigation as relevant to medication adherence. The application of geospatial techniques to locate and quantify relationships between social-contextual factors and medication adherence is a strength of this research. Adoption of these techniques in this field is warranted given the potential for practical application in identifying areas of risk. Interventions designed to address medication adherence can be tailored based on a further understanding of specific factors that may mediate or moderate the relationship between social vulnerability and medication adherence. The issue of medication adherence was recognised globally almost 20 years ago by the World Health Organization (Sabate, 2003), yet a continued rise in rates of T2DM in both developed and developing countries ensures that addressing medication adherence remains appropriate more than ever for improving health and quality of life outcomes for patients diagnosed with this condition.
Author statement
Concept and design: All authors Acquisition, analysis, or interpretation of the data: All authors Drafting of the manuscript: VLCritical revision of the manuscript for important intellectual content: All authors Statistical and spatial analyses: VL.
Funding and acknowledgements
This research was conducted as part of research project 3348 - Spatial Management of Health Risk funded by the Australian Digital Health Cooperative Research Centre (DHCRC) in collaboration with industry partner HMS (since acquired by Gainwell Technologies). We would like to acknowledge Donna Price, Dr Gary Call, MD, Ellen Harrison, Jason Cooper, Evan Caten and Prof Rachel Davey for their contributions to this research.
Ethical statement
This research was approved by the University of Canberra Human Research Ethics Review Panel (Approval #3348). The pharmacy claim data on which this study is based were used under agreement with HMS for the current study and are not publicly available. Exposure data including CDC ATSDR SVI and RUCA are publicly available from the sources listed in the manuscript.
Declaration of competing interest
None declared.
Appendix A. Supplementary data
The following is the Supplementary data to this article:Download : Download Word document (803KB)
Multimedia component 1.
Cited by (0)
Recommend
About Joyk
Aggregate valuable and interesting links.
Joyk means Joy of geeK