

REPL技术分析——Python的交互式
source link: https://chinomars.github.io/2020/02/12/REPL%E6%8A%80%E6%9C%AF%E5%88%86%E6%9E%90%E2%80%94%E2%80%94Python%E7%9A%84%E4%BA%A4%E4%BA%92%E5%BC%8F/
Go to the source link to view the article. You can view the picture content, updated content and better typesetting reading experience. If the link is broken, please click the button below to view the snapshot at that time.
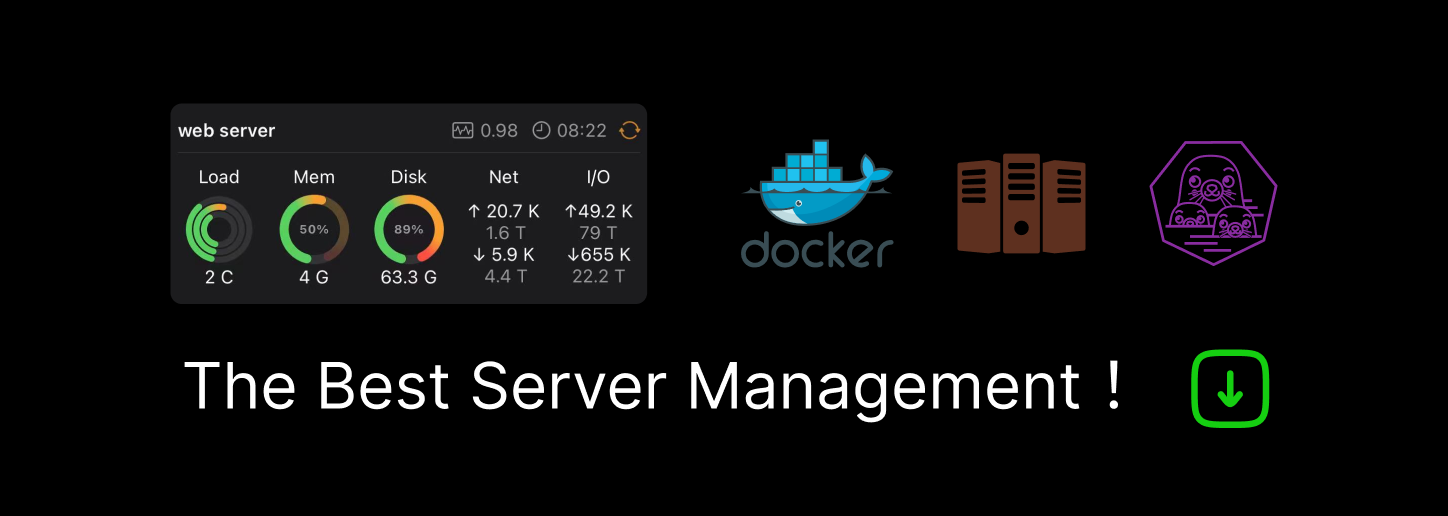
Python是一种解释型语言,通过解释器对代码进行逐行执行,一般的解释器也是这样实现,当然也存在一些优化方法,对代码进行JIT编译,提高执行速度。所以Python的REPL可以说是原生支持的。
Python语言有多种解释器,例如:
- CPython:C语言实现的Python解释器,一般情况下在Terminal中执行命令
python
,就会调用CPython解释器执行代码 - PyPy:前面提到的通过JIT技术提升Python代码执行速度
- IPython:Python的交互式解释器,底层也是通过调用CPython对代码进行解释执行
回到主题REPL,我们可以以IPython为入口进行分析,进一步对CPython进行分析
IPython
个人习惯,从源码出发分析。IPtyhon的github源码仓,链接,交互式开发的mainloop的代码在这个interactiveshell.py中,我们可以看到,IPython支持一个完整的代码块的交互式运行,采用异步的方式运行以保证一定的用户体验。一个完整的代码块,由用户输入,可以是一行完整的python代码,也可以是多行语法的代码块。
def _run_cell(self, raw_cell:str, store_history:bool, silent:bool, shell_futures:bool):
"""Internal method to run a complete IPython cell."""
coro = self.run_cell_async(
raw_cell,
store_history=store_history,
silent=silent,
shell_futures=shell_futures,
)
# run_cell_async is async, but may not actually need an eventloop.
# when this is the case, we want to run it using the pseudo_sync_runner
# so that code can invoke eventloops (for example via the %run , and
# `%paste` magic.
if self.trio_runner:
runner = self.trio_runner
elif self.should_run_async(raw_cell):
runner = self.loop_runner
else:
runner = _pseudo_sync_runner
try:
return runner(coro)
except BaseException as e:
info = ExecutionInfo(raw_cell, store_history, silent, shell_futures)
result = ExecutionResult(info)
result.error_in_exec = e
self.showtraceback(running_compiled_code=True)
return result
return
继续分析这个run_cell_async:
async def run_cell_async(self, raw_cell: str, store_history=False, silent=False, shell_futures=True) -> ExecutionResult:
info = ExecutionInfo(
raw_cell, store_history, silent, shell_futures)
result = ExecutionResult(info)
...
# If any of our input transformation (input_transformer_manager or
# prefilter_manager) raises an exception, we store it in this variable
# so that we can display the error after logging the input and storing
# it in the history.
try:
cell = self.transform_cell(raw_cell)
...
# Store raw and processed history
...
# Display the exception if input processing failed.
...
# Our own compiler remembers the __future__ environment. If we want to
# run code with a separate __future__ environment, use the default
# compiler
compiler = self.compile if shell_futures else CachingCompiler()
_run_async = False
with self.builtin_trap:
cell_name = self.compile.cache(cell, self.execution_count)
with self.display_trap:
# Compile to bytecode
try:
if sys.version_info < (3,8) and self.autoawait:
if _should_be_async(cell):
# the code AST below will not be user code: we wrap it
# in an `async def`. This will likely make some AST
# transformer below miss some transform opportunity and
# introduce a small coupling to run_code (in which we
# bake some assumptions of what _ast_asyncify returns.
# they are ways around (like grafting part of the ast
# later:
# - Here, return code_ast.body[0].body[1:-1], as well
# as last expression in return statement which is
# the user code part.
# - Let it go through the AST transformers, and graft
# - it back after the AST transform
# But that seem unreasonable, at least while we
# do not need it.
code_ast = _ast_asyncify(cell, 'async-def-wrapper')
_run_async = True
else:
code_ast = compiler.ast_parse(cell, filename=cell_name)
else:
code_ast = compiler.ast_parse(cell, filename=cell_name)
...
# Apply AST transformations
try:
code_ast = self.transform_ast(code_ast)
...
# Execute the user code
interactivity = "none" if silent else self.ast_node_interactivity
if _run_async:
interactivity = 'async'
has_raised = await self.run_ast_nodes(code_ast.body, cell_name,
interactivity=interactivity, compiler=compiler, result=result)
...
# Reset this so later displayed values do not modify the
# ExecutionResult
self.displayhook.exec_result = None
...
return result
我把一些异常处理的代码省略了,不关键的跳过。删除不关键的处理流程后我们可以分析下源码:
- 首先是将cell代码块(raw_cell),执行历史(store_history),以及一些设置运行模式的参数(silent和shell_futures)来示例化成ExecutionInfo,然后将它塞到ExecutionResult这个方法中,做进一步的封装,方便后续进行执行过程中关键信息的存储
tramsform_cell
的工作主要是做一些行的分割以及其他处理,例如确保每一个输入cell最后有一个空行,这个也是编译器的常规操作,方便parsing的时候计算报错行号_ast_asyncify
是一个异步方法,将输入cell(源码)解析为ast,同样其他两个分支都是将输入解析为ast,这里的分支是为了区分版本,解决版本兼容性。这里的compiler
我们没有在IPython源码中找到定义,推测是CPython的封装,后续再分析run_ast_nodes
也是调用了compiler的能力,下面我们就可以去CPython中进一步分析了
IPython主要对CPython进行封装,将ast导入给CPython进行执行。并且,部分情况下并没有调用compiler封装的run_code方法,而是直接使用Python内置的exec()方法执行python代码,处理也比较简单。
CPython
CPython是python解释器的c语言实现,也是Python的官方解释器。按照惯例我们还是从源码入手,cpython托管在github上,项目链接。
首先从main函数出发,找到Programs/python.c
中的main函数,在进入到repl loop之前,我们快速过一下执行流程。当然,对于c/c++项目而言,最万能的方式还是通过调试,一步一步地借助断点和查看调用栈来分析。在只关注一个具体的功能的时候,个人还是比较偏向于直接看源码,聚焦关键的函数。
执行流程:
Programs/python.c:16 => Py_BytesMain
Modules/main.c:679 => pymain_main
Modules/main.c:627 Py_RunMain => pymain_run_python
到pymain_run_python()函数,我们可以具体看一下这个函数里的构成:
static void
pymain_run_python(int *exitcode)
{
...
if (config->run_command) {
*exitcode = pymain_run_command(config->run_command, &cf);
}
else if (config->run_module) {
*exitcode = pymain_run_module(config->run_module, 1);
}
else if (main_importer_path != NULL) {
*exitcode = pymain_run_module(L"__main__", 0);
}
else if (config->run_filename != NULL) {
*exitcode = pymain_run_file(config, &cf);
}
else {
*exitcode = pymain_run_stdin(config, &cf);
}
pymain_repl(config, &cf, exitcode);
goto done;
...
}
在这个函数中,通过函数最开始构建的运行环境配置(config),来决定后续的分支:
- run_command分支:调用
pymain_run_command()
函数,来执行命令 - run_module分支:调用
pymain_run_module()
函数,运行一个python模块 - run_filename分支:调用
pymain_run_file()
函数,运行一个python文件 - 其他:调用
pymain_run_stdin()
函数,来执行一个标准输入 - 最后:调用
pymain_repl()
函数,启动repl
上述执行分支中,估计大家对于最后两个分支(其他和最后)会感到十分疑惑,看起来逻辑有重复,其他分支中,调用pymain_run_stdin()
函数后,再启动repl。实际上最后两个分支最终调用的函数都是一样的:
pymain_run_stdin()
:
static int
pymain_run_stdin(PyConfig *config, PyCompilerFlags *cf)
{
...
int run = PyRun_AnyFileExFlags(stdin, "<stdin>", 0, cf);
return (run != 0);
}
pymain_repl()
:
static void
pymain_repl(PyConfig *config, PyCompilerFlags *cf, int *exitcode)
{
...
int res = PyRun_AnyFileFlags(stdin, "<stdin>", cf);
*exitcode = (res != 0);
}
实际上,在pymain_repl()
中调用的PyRun_AnyFileFlags()
,在include/pythonrun.h
中定义为:
#define PyRun_AnyFileFlags(fp, name, flags) \
PyRun_AnyFileExFlags(fp, name, 0, flags)
是一毛一样的呢。最终就执行到了我们的重头戏:Python/pythonrun.c:91 PyRun_InteractiveLoopFlags()
interactive loop
PyRun_InteractiveLoopFlags(stdin, "<stdin>", 0, cf)
中,从stdin
标准输入流中读取用户输入,进行执行:
int
PyRun_InteractiveLoopFlags(FILE *fp, const char *filename_str, PyCompilerFlags *flags)
{
...
err = 0;
do {
ret = PyRun_InteractiveOneObjectEx(fp, filename, flags);
if (ret == -1 && PyErr_Occurred()) {
...
PyErr_Print();
flush_io();
} else {
nomem_count = 0;
}
} while (ret != E_EOF);
Py_DECREF(filename);
return err;
}
调用PyRun_InteractiveOneObjectEx()
函数执行用户输入。
我们可以看到对于一个Python Object的执行流程如下:
_PyUnicode_FromId
:造一个modulename_PySys_GetObjectId
:从stdin中读取用户输入PyImport_AddModuleObject
:加载import模块run_mod
:运行module
run_mod()
中:
static PyObject *
run_mod(mod_ty mod, PyObject *filename, PyObject *globals, PyObject *locals,
PyCompilerFlags *flags, PyArena *arena)
{
PyCodeObject *co;
PyObject *v;
co = PyAST_CompileObject(mod, filename, flags, -1, arena);
if (co == NULL)
return NULL;
if (PySys_Audit("exec", "O", co) < 0) {
Py_DECREF(co);
return NULL;
}
v = run_eval_code_obj(co, globals, locals);
Py_DECREF(co);
return v;
}
先将python moduleParse成AST(调用PyAST_CompileObject()
函数),再编译成Python的ByteCode,最后塞给run_eval_code_obj()
函数进行执行。
基本上repl的执行流程就讲完了,有点困了,有(bu)时(xiang)间(nong)再细化补充,欢迎留言。
源码分析地比较粗糙,找到一片详细debug,介绍cpython中的编译执行流程的博客,见最后一片参考文章(Internals of CPython),写得比较详细,甚至还简单介绍了gdb的使用方式,很贴心。
Reference
Recommend
About Joyk
Aggregate valuable and interesting links.
Joyk means Joy of geeK