

PyTorch之对类别张量进行one-hot编码
source link: https://segmentfault.com/a/1190000041291494
Go to the source link to view the article. You can view the picture content, updated content and better typesetting reading experience. If the link is broken, please click the button below to view the snapshot at that time.
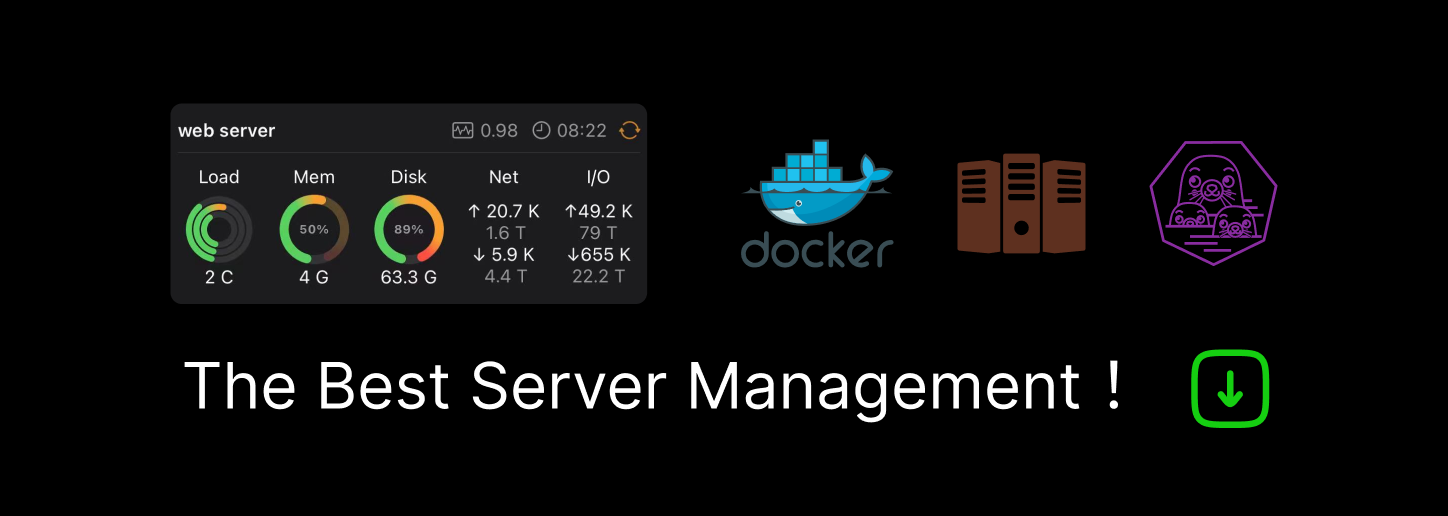
PyTorch之对类别张量进行one-hot编码
本文已授权极市平台, 并首发于极市平台公众号. 未经允许不得二次转载.
one-hot 形式的编码在深度学习任务中非常常见,但是却并不是一种很自然的数据存储方式。所以大多数情况下都需要我们自己手动转换。虽然思路很直接,就是将类别拆分成一一对应的 0-1 向量,但是具体实现起来确实还是需要思考下的。实际上 pytorch 自身在nn.functional
中已经提供了one_hot
方法来快速应用。但是这并不能影响我们的思考与实践:>!所以本文尽可能将基于 pytorch 中常用方法来实现one-hot
编码的方式整理了下,希望有用。
主要的方式有这么几种:
for
循环scatter
index_select
for
循环
这种方法非常直观,说白了就是对一个空白(全零)张量中的指定位置进行赋值(赋 1)操作即可。
关键在于如何设定索引。
下面设计了两种本质相同但由于指定维度不同而导致些许差异的方案。
def bhw_to_onehot_by_for(bhw_tensor: torch.Tensor, num_classes: int): """ Args: bhw_tensor: b,h,w num_classes: Returns: b,h,w,num_classes """ assert bhw_tensor.ndim == 3, bhw_tensor.shape assert num_classes > bhw_tensor.max(), torch.unique(bhw_tensor) one_hot = bhw_tensor.new_zeros(size=(num_classes, *bhw_tensor.shape)) for i in range(num_classes): one_hot[i, bhw_tensor == i] = 1 one_hot = one_hot.permute(1, 2, 3, 0) return one_hot def bhw_to_onehot_by_for_V1(bhw_tensor: torch.Tensor, num_classes: int): """ Args: bhw_tensor: b,h,w num_classes: Returns: b,h,w,num_classes """ assert bhw_tensor.ndim == 3, bhw_tensor.shape assert num_classes > bhw_tensor.max(), torch.unique(bhw_tensor) one_hot = bhw_tensor.new_zeros(size=(*bhw_tensor.shape, num_classes)) for i in range(num_classes): one_hot[..., i][bhw_tensor == i] = 1 return one_hot
scatter
该方法应该是网上大多数简洁的one_hot
写法的常用形式了。其实际上主要的作用是向 tensor 中指定的位置上赋值。
由于其可以使用专门构造的索引矩阵来作为索引,所以更加灵活。当然,灵活带来的也就是理解上的困难。官方文档中提供的解释非常直观:
''' https://pytorch.org/docs/stable/generated/torch.Tensor.scatter_.html * (int dim, Tensor index, Tensor src) * (int dim, Tensor index, Tensor src, *, str reduce) * (int dim, Tensor index, Number value) * (int dim, Tensor index, Number value, *, str reduce) ''' self[index[i][j][k]][j][k] = src[i][j][k] # if dim == 0 self[i][index[i][j][k]][k] = src[i][j][k] # if dim == 1 self[i][j][index[i][j][k]] = src[i][j][k] # if dim == 2
文档中使用的是原地置换(in-place
)版本,并且基于替换值为src
,即 tensor 的情况下来解释。实际上在我们的应用中主要基于原地置换版本并搭配替换值为标量浮点数value
的形式。
上述的形式中,我们可以看到,通过指定参数 tensor index
,我们就可以将src
中(i,j,k)
的值放置到方法调用者(这里是self
)的指定位置上。该指定位置由index
的(i,j,k)
处的值替换坐标(i,j,k)
中的dim
位置的值来构成(这里也反映出来了index
tensor 的一个要求,就是维度数量要和self
、src
(如果src
为 tensor 的话。后文中使用的是具体的标量值 1,即src
替换为value
)一致)。这倒是和one-hot
的概念非常吻合。因为one-hot
本身形式上的含义就是对于第i
类数据,第i
个位置为 1,其余位置为 0。所以对全零 tensor 使用scatter_
是可以非常容易的构造出one-hot
tensor 的,即对对应于类别编号的位置放置 1 即可。
对于我们的问题而言,index
非常适合使用输入的包含类别编号的 tensor(形状为B,H,W
)来表示。基于这样的思考,可以构思出两种不同的策略:
def bhw_to_onehot_by_scatter(bhw_tensor: torch.Tensor, num_classes: int): """ Args: bhw_tensor: b,h,w num_classes: Returns: b,h,w,num_classes """ assert bhw_tensor.ndim == 3, bhw_tensor.shape assert num_classes > bhw_tensor.max(), torch.unique(bhw_tensor) one_hot = torch.zeros(size=(math.prod(bhw_tensor.shape), num_classes)) one_hot.scatter_(dim=1, index=bhw_tensor.reshape(-1, 1), value=1) one_hot = one_hot.reshape(*bhw_tensor.shape, num_classes) return one_hot def bhw_to_onehot_by_scatter_V1(bhw_tensor: torch.Tensor, num_classes: int): """ Args: bhw_tensor: b,h,w num_classes: Returns: b,h,w,num_classes """ assert bhw_tensor.ndim == 3, bhw_tensor.shape assert num_classes > bhw_tensor.max(), torch.unique(bhw_tensor) one_hot = torch.zeros(size=(*bhw_tensor.shape, num_classes)) one_hot.scatter_(dim=-1, index=bhw_tensor[..., None], value=1) return one_hot
这两种形式的差异的根源在于对形状的处理上。由此带来了scatter
不同的应用形式。
对于第一种形式,将B,H,W
三个维度合并,这样的好处是对通道(类别)的索引的理解变得直观起来。
one_hot = torch.zeros(size=(math.prod(bhw_tensor.shape), num_classes)) one_hot.scatter_(dim=1, index=bhw_tensor.reshape(-1, 1), value=1)
这里将类别维度和其他维度直接分离,移到了末位。通过dim
指定该维度,于是就有了这样的对应关系:
zero_tensor[abc, index[abc][d]] = value # d=0
而在第二种情况下仍然保留了前面的三个维度,类别维度依然移动到最后一位。
one_hot = torch.zeros(size=(*bhw_tensor.shape, num_classes)) one_hot.scatter_(dim=-1, index=bhw_tensor[..., None], value=1)
此时的对应关系是这样的:
zero_tensor[a,b,c, index[a][b][c][d]] = value # d=0
另外在 pytorch 分类模型库 timm
中,也使用了类似的方法:
# https://github.com/rwightman/pytorch-image-models/blob/2c33ca6d8ce5d9257edf8cab5ab7ece81780aaf7/timm/data/mixup.py#L17-L19 def one_hot(x, num_classes, on_value=1., off_value=0., device='cuda'): x = x.long().view(-1, 1) return torch.full((x.size()[0], num_classes), off_value, device=device).scatter_(1, x, on_value)
index_select
torch.index_select(input, dim, index, *, out=None) → Tensor - input (Tensor) – the input tensor. - dim (int) – the dimension in which we index - index (IntTensor or LongTensor) – the 1-D tensor containing the indices to index
该函数如其名,就是用索引来选择 tensor 的指定维度的子 tensor 的。
想要理解这一方法的动机,实际上需要反过来,从类别标签的角度看待one-hot
编码。
对于原始从小到大排布的类别序号对应的one-hot
编码成的矩阵就是一个单位矩阵。所以每个类别对应的就是该单位矩阵的特定的列(或者行)。这一需求恰好符合index_select
的功能。所以我们可以使用其实现one_hot
编码,只需要使用类别序号索引特定的列或者行即可。下面就是一个例子:
def bhw_to_onehot_by_index_select(bhw_tensor: torch.Tensor, num_classes: int): """ Args: bhw_tensor: b,h,w num_classes: Returns: b,h,w,num_classes """ assert bhw_tensor.ndim == 3, bhw_tensor.shape assert num_classes > bhw_tensor.max(), torch.unique(bhw_tensor) one_hot = torch.eye(num_classes).index_select(dim=0, index=bhw_tensor.reshape(-1)) one_hot = one_hot.reshape(*bhw_tensor.shape, num_classes) return one_hot
整体代码可见GitHub。
下面展示了不同方法的大致的相对性能(因为后台在跑程序,可能并不是十分准确,建议大家自行测试)。可以看到,pytorch 自带的函数在 CPU 上效率并不是很高,但是在 GPU 上表现良好。其中有趣的是,基于index_select
的形式表现非常亮眼。
1.10.0 GeForce RTX 2080 Ti cpu ('bhw_to_onehot_by_for', 0.5411529541015625) ('bhw_to_onehot_by_for_V1', 0.4515676498413086) ('bhw_to_onehot_by_scatter', 0.0686192512512207) ('bhw_to_onehot_by_scatter_V1', 0.08529376983642578) ('bhw_to_onehot_by_index_select', 0.05156970024108887) ('F.one_hot', 0.07366824150085449) gpu ('bhw_to_onehot_by_for', 0.005235433578491211) ('bhw_to_onehot_by_for_V1', 0.045584678649902344) ('bhw_to_onehot_by_scatter', 0.0025513172149658203) ('bhw_to_onehot_by_scatter_V1', 0.0024869441986083984) ('bhw_to_onehot_by_index_select', 0.002012014389038086) ('F.one_hot', 0.0024051666259765625)
Recommend
About Joyk
Aggregate valuable and interesting links.
Joyk means Joy of geeK