

Avoiding selection bias in your usability tests
source link: https://uxdesign.cc/avoiding-selection-bias-in-your-usability-tests-5ee7a6a3e7c0
Go to the source link to view the article. You can view the picture content, updated content and better typesetting reading experience. If the link is broken, please click the button below to view the snapshot at that time.
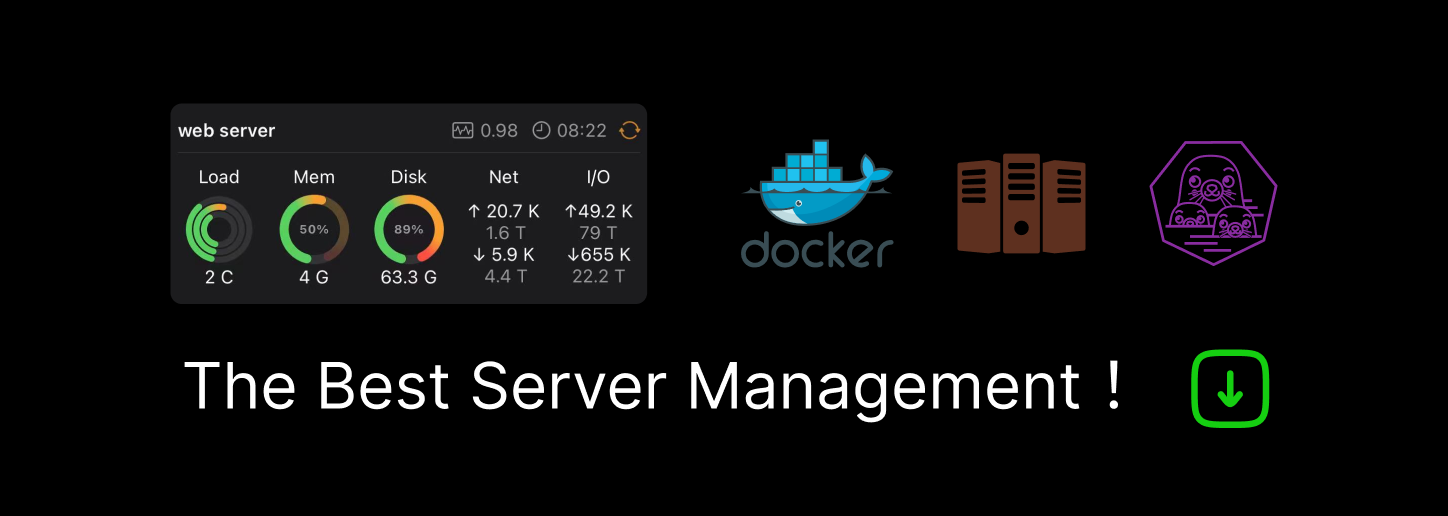
Avoiding selection bias in your usability tests
Improve your usability testing skills using the power of statistics

It is both irritating and fascinating to watch a user discover a design flaw. Even your most meticulous designs will get feedback you never expected. This is why usability studies are such an essential research method for a UX designer. They help you to identify those issues in a structured process. But how meaningful are the results of your study? If you don’t choose your participants with care, the outcomes might not be representative at all.
Selection bias occurs when you only recruit participants with little to no depiction of your user group. This generates misleading results. Take the example of an internationally distributed product with an English interface. If you only recruit native English speakers, you miss feedback from non-native users. You will most likely overlook significant pitfalls.
Usability studies are fueled by statistics
Usability tests are powerful for one fundamental reason: the central limit theorem. The concept behind is quite easy to understand. In a usability test, a percentage of participants face a certain challenge. This issue will affect about the same percentage of all people in your user group. The prerequisite to applying this principle is that you select a large, representative user sample.
The central limit theorem only holds true if you have a limited number of possible outcomes for each task in your test. This would not pertain to studies only tracking open-ended data. Yet, most usability tests use a mix of data types. Participants “think out loud” while trying to complete a task (qualitative, open-ended data). The completion rate tracks whether your participants have been successful (quantitative data). More complex studies also capture categorical data points like error type or severity.
How to achieve a large, representative sample?
First, you have to recruit the right amount of people. Whilst there is no ‘one-size-fits-all’ number for every usability test, to apply the central limit theorem you need at least 30 participants. This may sound like a very high number at first, but it is a justified selection size. One out of three users (33%) unable to complete a task in a test can distort your results. There is a high chance this one user is an outlier. Yet, one user having difficulties within a sample of 30 people (3%) does not have a significant impact.
Second, your participants need to be representative of your target group. Hence, recruitment via UserZoom or UserTesting might not be the best option. Any tool that depends on people to select into the study, will capture only the views of those who make the effort. These are likely people who have a strong opinion about an issue, or those who need the extra money.
Recruit like this instead
To achieve a representative sample, professional research institutes resort to the following measures:
1. Select people from your user group at random
The foundation is that you have a clear understanding of the anatomy of your user group. If you have no idea who you want to represent in the test, you can’t prevent selection bias. I’m not going into detail on user analytics, but there are a lot of great resources on the web that can help you get started.
Next, you need to select which users are a target group for the test. Not every usability test is relevant for every user. Are you trying to target a specific age group, profession, or industry? Make sure to create a separate user database for your usability study. Google Analytics and other analytics tools can help you with the segmentation.
Once you have the segment, create a random selection of people from that database. One option to ask them for participation can be within the UI of your product. Another option is to contact them via email or phone if you have permission. Whatever option makes the most sense for your target group.
2. Give them more than one chance to participate
If the selected person is not available at first, wait until they are. Of course, it would be easier to keep on selecting people until you reach 30 participants. But that would make your data biased towards a specific group of people. This might exclude people from other time zones or users who are otherwise occupied.
3. Track your participation rate
Even if you give everyone a very generous period of time to take part, not all will. The best way to see if your study is subject to selection bias is to track the participation rate. It tells you how many of the people you selected as participants completed the test. A low participation rate can be an alarm signal for selection bias. Some users might have not have permitted you to contact them, refuse to take part, or do not reply to your request. The larger this user group is, the more likely it is to be different from your participants in some material way.
Further analysis of this group will help determine if you have a skewed sample. Do you have contact permission from a representative group of users? Are they refusing to take part for a particular reason? Do they belong to a specific demographic? Collecting further data (within applicable data protection laws) leads to a better understanding of the significance of your outcome.
One step at a time
Avoiding all selection bias in a usability study can be difficult to impossible. Time restrictions and a limited pool of participants are challenges for most teams. Instead of trying to overturn the whole process, start with one improvement.
Conducting studies with 30 participants is demanding, even for the most experienced teams. As an alternative, you might want to consider testing in batches. For example, start conducting a study with ten participants. If necessary, you then repeat the test with ten more participants. One reason could be that a serious design error was only experienced by one participant. This way, you increase the confidence level of your results as needed with minimal cost.
Another great place to start would be to do a bit more demographic analysis on your participants. How do they compare to your total user group? What tweaks can you make to bring the two closer together? Even tiny changes to your recruitment can have a huge impact on creating better products.
Recommend
About Joyk
Aggregate valuable and interesting links.
Joyk means Joy of geeK