

8.深入TiDB:解析Hash Join实现原理
source link: https://www.luozhiyun.com/archives/631
Go to the source link to view the article. You can view the picture content, updated content and better typesetting reading experience. If the link is broken, please click the button below to view the snapshot at that time.
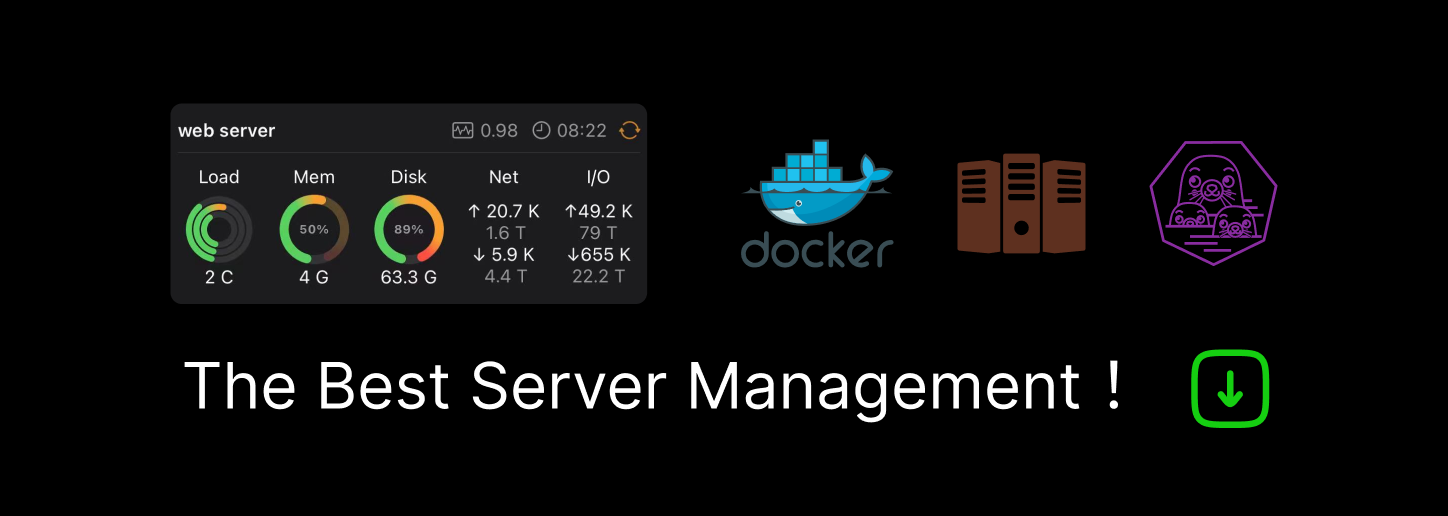
8.深入TiDB:解析Hash Join实现原理
本文基于 TiDB release-5.1进行分析,需要用到 Go 1.16以后的版本
所谓 Hash Join 就是在 join 的时候选择一张表作为 buildSide 表来构造哈希表,另外一张表作为 probeSide 表;然后对 probeSide 表的每一行数据都去这个哈希表中查找是否有匹配的数据。
根据上面的定义,看起来 Hash Join 貌似很好做,只需要弄一个大 map 然后遍历 probeSide 表的数据进行匹配就好了。但是作为一个高效的数据库, TiDB 会在这个过程做什么优化呢?
所以在阅读文章前先带着这几个疑问:
- 哪张表会成为 buildSide 表或 probeSide 表?
- buildSide 表来构造的哈希表是包含了 buildSide 表的所有数据吗?数据量太大会不会有问题?
- probeSide 表匹配 buildSide 表的时候是单线程匹配还是多线程匹配?如果是多线程匹配,那么如何分配匹配的数据呢?
下面我用这个例子来进行讲解:
CREATE TABLE test1 (a int , b int, c int, d int);
CREATE TABLE test2 (a int , b int, c int, d int);
然后查询执行计划:
explain select * from test1 t1 join test1 t2 on t1.a= t2.a ;
+-----------------------+--------+---------+-------------+--------------------------------------------------+
|id |estRows |task |access object|operator info |
+-----------------------+--------+---------+-------------+--------------------------------------------------+
|HashJoin_8 |12487.50|root | |inner join, equal:[eq(test.test1.a, test.test1.a)]|
|├─TableReader_15(Build)|9990.00 |root | |data:Selection_14 |
|│ └─Selection_14 |9990.00 |cop[tikv]| |not(isnull(test.test1.a)) |
|│ └─TableFullScan_13 |10000.00|cop[tikv]|table:t2 |keep order:false, stats:pseudo |
|└─TableReader_12(Probe)|9990.00 |root | |data:Selection_11 |
| └─Selection_11 |9990.00 |cop[tikv]| |not(isnull(test.test1.a)) |
| └─TableFullScan_10 |10000.00|cop[tikv]|table:t1 |keep order:false, stats:pseudo |
+-----------------------+--------+---------+-------------+--------------------------------------------------+
构建 Hash Join 执行器
-
TiDB 首先会根据 SQL 来构建相应的 Logic Plan;
-
然后将 Logic Plan 转成 Physical Plan,这里是转成 PhysicalHashJoin 作为 Physical Plan;
-
通过比较 Physical Plan 的代价,最后选择一个代价最小的 Physical Plan 构建执行器 executor;
之所以要讲一下这里是因为通过 Physical Plan 构建执行器的时候会判断是哪张表来做 buildSide 表 或 probeSide 表;
构建 Physical Plan
构建 Physical Plan 在exhaust_physical_plans.go文件的 getHashJoins 方法中:
func (p *LogicalJoin) getHashJoins(prop *property.PhysicalProperty) []PhysicalPlan {
...
joins := make([]PhysicalPlan, 0, 2)
switch p.JoinType {
case SemiJoin, AntiSemiJoin, LeftOuterSemiJoin, AntiLeftOuterSemiJoin:
joins = append(joins, p.getHashJoin(prop, 1, false))
case LeftOuterJoin:
joins = append(joins, p.getHashJoin(prop, 1, false))
joins = append(joins, p.getHashJoin(prop, 1, true))
case RightOuterJoin:
joins = append(joins, p.getHashJoin(prop, 0, false))
joins = append(joins, p.getHashJoin(prop, 0, true))
case InnerJoin:
joins = append(joins, p.getHashJoin(prop, 1, false))
joins = append(joins, p.getHashJoin(prop, 0, false))
}
return joins
}
这个方法会根据 Join 的类型分别调用 getHashJoin 方法创建 Physical Plan。 这里会创建多个 PhysicalHashJoin ,后面会选择一个代价最小的 Physical Plan 构建执行器。
需要注意的是 getHashJoin 后面两个参数:
func (p *LogicalJoin) getHashJoin(prop *property.PhysicalProperty, innerIdx int, useOuterToBuild bool) *PhysicalHashJoin
后面会根据 innerIdx 和 useOuterToBuild 决定哪张会成为 buildSide 表 或 probeSide 表;
选择效率最高的执行计划
构建好 Physical Plan 之后会遍历创建的 Plan 获取它的代价:
func (p *baseLogicalPlan) enumeratePhysicalPlans4Task(physicalPlans []PhysicalPlan, prop *property.PhysicalProperty, addEnforcer bool, planCounter *PlanCounterTp) (task, int64, error) {
var bestTask task = invalidTask
childTasks := make([]task, 0, len(p.children))
for _, pp := range physicalPlans {
childTasks = childTasks[:0]
for j, child := range p.children {
childTask, cnt, err := child.findBestTask(pp.GetChildReqProps(j), &PlanCounterDisabled)
...
childTasks = append(childTasks, childTask)
}
// Combine best child tasks with parent physical plan.
curTask := pp.attach2Task(childTasks...)
...
// Get the most efficient one.
if curTask.cost() < bestTask.cost() || (bestTask.invalid() && !curTask.invalid()) {
bestTask = curTask
}
}
return bestTask, ...
}
从这些 Plan 里面挑选出代价最小的返回。
通过执行计划构建执行器
获取到执行计划之后,会通过一系列的调用到 buildHashJoin 构建 HashJoinExec 作为 hash join 执行器:
我们来看一下 buildHashJoin:
func (b *executorBuilder) buildHashJoin(v *plannercore.PhysicalHashJoin) Executor {
// 构建左表 executor
leftExec := b.build(v.Children()[0])
if b.err != nil {
return nil
}
// 构建右表 executor
rightExec := b.build(v.Children()[1])
if b.err != nil {
return nil
}
// 构建
e := &HashJoinExec{
baseExecutor: newBaseExecutor(b.ctx, v.Schema(), v.ID(), leftExec, rightExec),
concurrency: v.Concurrency,
// join 类型
joinType: v.JoinType,
isOuterJoin: v.JoinType.IsOuterJoin(),
useOuterToBuild: v.UseOuterToBuild,
}
...
//选择 buildSideExec 和 probeSideExec
if v.UseOuterToBuild {
if v.InnerChildIdx == 1 { // left join InnerChildIdx =1
e.buildSideExec, e.buildKeys = leftExec, v.LeftJoinKeys
e.probeSideExec, e.probeKeys = rightExec, v.RightJoinKeys
e.outerFilter = v.LeftConditions
} else {
e.buildSideExec, e.buildKeys = rightExec, v.RightJoinKeys
e.probeSideExec, e.probeKeys = leftExec, v.LeftJoinKeys
e.outerFilter = v.RightConditions
}
} else {
if v.InnerChildIdx == 0 {
e.buildSideExec, e.buildKeys = leftExec, v.LeftJoinKeys
e.probeSideExec, e.probeKeys = rightExec, v.RightJoinKeys
e.outerFilter = v.RightConditions
} else {
e.buildSideExec, e.buildKeys = rightExec, v.RightJoinKeys
e.probeSideExec, e.probeKeys = leftExec, v.LeftJoinKeys
e.outerFilter = v.LeftConditions
}
}
childrenUsedSchema := markChildrenUsedCols(v.Schema(), v.Children()[0].Schema(), v.Children()[1].Schema())
e.joiners = make([]joiner, e.concurrency)
for i := uint(0); i < e.concurrency; i++ {
// 创建 joiner 用于 Join 匹配
e.joiners[i] = newJoiner(b.ctx, v.JoinType, v.InnerChildIdx == 0, defaultValues,
v.OtherConditions, lhsTypes, rhsTypes, childrenUsedSchema)
}
...
return e
}
这段主要的逻辑就是根据最优的 Physical Plan 来构建 HashJoinExec。
其中需要主要的是,这里会根据 UseOuterToBuild 和 InnerChildIdx 来决定 buildSide 表和 probeSide 表。
比如在构建 left join 的 Physical Plan 的时候:
func (p *LogicalJoin) getHashJoins(prop *property.PhysicalProperty) []PhysicalPlan {
...
joins := make([]PhysicalPlan, 0, 2)
switch p.JoinType {
case LeftOuterJoin:
joins = append(joins, p.getHashJoin(prop, 1, false))
joins = append(joins, p.getHashJoin(prop, 1, true))
...
}
return joins
}
传入的 getHashJoin 方法中第一个参数代表 InnerChildIdx,第二个参数代表 UseOuterToBuild。这里会生成两个 Physical Plan ,然后会根据代价计算出最优的那个;
进入到 buildHashJoin 方法的时候,可以发现 buildSide 表和 probeSide 表是最后和 Physical Plan 有关:
func (b *executorBuilder) buildHashJoin(v *plannercore.PhysicalHashJoin) Executor {
...
//选择 buildSideExec 和 probeSideExec
if v.UseOuterToBuild {
if v.InnerChildIdx == 1 { // left join InnerChildIdx =1
e.buildSideExec, e.buildKeys = leftExec, v.LeftJoinKeys
e.probeSideExec, e.probeKeys = rightExec, v.RightJoinKeys
e.outerFilter = v.LeftConditions
} else {
...
}
} else {
if v.InnerChildIdx == 0 {
...
} else {
e.buildSideExec, e.buildKeys = rightExec, v.RightJoinKeys
e.probeSideExec, e.probeKeys = leftExec, v.LeftJoinKeys
e.outerFilter = v.LeftConditions
}
}
...
return e
}
运行Hash Join 执行器
在构建完 HashJoinExec 之后就到了获取数据的环节,TiDB 会通过 Next 方法一次性从执行器里面获取一批数据,具体获取数据的方法在 HashJoinExec 的 Next 里面。
func (e *HashJoinExec) Next(ctx context.Context, req *chunk.Chunk) (err error) {
if !e.prepared {
e.buildFinished = make(chan error, 1)
// 异步根据buildSide表中数据, 构建 hashtable
go util.WithRecovery(func() {
defer trace.StartRegion(ctx, "HashJoinHashTableBuilder").End()
e.fetchAndBuildHashTable(ctx)
}, e.handleFetchAndBuildHashTablePanic)
// 读取probeSide表和构建的hashtable做匹配,获取数据放入joinResultCh
e.fetchAndProbeHashTable(ctx)
e.prepared = true
}
if e.isOuterJoin {
atomic.StoreInt64(&e.requiredRows, int64(req.RequiredRows()))
}
req.Reset()
// 获取结果数据
result, ok := <-e.joinResultCh
if !ok {
return nil
}
if result.err != nil {
e.finished.Store(true)
return result.err
}
// 将数据返回放入到 req Chunk 中
req.SwapColumns(result.chk)
result.src <- result.chk
return nil
}
Next 方法获取数据分为三步:
- 调用 fetchAndBuildHashTable 方法异步根据buildSide表中数据, 构建 hashtable;
- 调用 fetchAndProbeHashTable 方法读取probeSide表和构建的hashtable做匹配,获取数据放入joinResultCh;
- 从 joinResultCh 中获取数据;
fetchAndBuildHashTable 构建 hash table
func (e *HashJoinExec) fetchAndBuildHashTable(ctx context.Context) {
...
buildSideResultCh := make(chan *chunk.Chunk, 1)
doneCh := make(chan struct{})
go util.WithRecovery(
func() {
defer trace.StartRegion(ctx, "HashJoinBuildSideFetcher").End()
// 获取 buildSide 表中的数据,将数据放入到 buildSideResultCh 中
e.fetchBuildSideRows(ctx, buildSideResultCh, doneCh)
}, ...,
)
// 从 buildSideResultCh 中读取数据构建 rowContainer
err := e.buildHashTableForList(buildSideResultCh)
if err != nil {
e.buildFinished <- errors.Trace(err)
close(doneCh)
}
...
}
这里构建 hash map 的过程分为两部分:
- 异步调用 fetchBuildSideRows 循环获取buildSide表中数据,放入到 buildSideResultCh 中;
- 从 buildSideResultCh 中读取数据构建 rowContainer,rowContainer 相当于 hash map 存放数据的地方。
我们下面来看一下 buildHashTableForList:
func (e *HashJoinExec) buildHashTableForList(buildSideResultCh <-chan *chunk.Chunk) error {
e.rowContainer = newHashRowContainer(e.ctx, int(e.buildSideEstCount), hCtx)
...
// 读取 channel 数据
for chk := range buildSideResultCh {
if e.finished.Load().(bool) {
return nil
}
if !e.useOuterToBuild {
// 将数据存入到 rowContainer 中
err = e.rowContainer.PutChunk(chk, e.isNullEQ)
} else {
...
}
if err != nil {
return err
}
}
return nil
}
这里会将 chunk 的数据通过 PutChunk 存入到 rowContainer 中。
func (c *hashRowContainer) PutChunk(chk *chunk.Chunk, ignoreNulls []bool) error {
return c.PutChunkSelected(chk, nil, ignoreNulls)
}
func (c *hashRowContainer) PutChunkSelected(chk *chunk.Chunk, selected, ignoreNulls []bool) error {
start := time.Now()
defer func() { c.stat.buildTableElapse += time.Since(start) }()
chkIdx := uint32(c.rowContainer.NumChunks())
// 将数据存放到 RowContainer 中,内存中放不下会存放到磁盘中
err := c.rowContainer.Add(chk)
if err != nil {
return err
}
numRows := chk.NumRows()
c.hCtx.initHash(numRows)
hCtx := c.hCtx
// 根据chunk中的column值构建hash值
for keyIdx, colIdx := range c.hCtx.keyColIdx {
ignoreNull := len(ignoreNulls) > keyIdx && ignoreNulls[keyIdx]
err := codec.HashChunkSelected(c.sc, hCtx.hashVals, chk, hCtx.allTypes[colIdx], colIdx, hCtx.buf, hCtx.hasNull, selected, ignoreNull)
if err != nil {
return errors.Trace(err)
}
}
// 根据hash值构建hash table
for i := 0; i < numRows; i++ {
if (selected != nil && !selected[i]) || c.hCtx.hasNull[i] {
continue
}
key := c.hCtx.hashVals[i].Sum64()
rowPtr := chunk.RowPtr{ChkIdx: chkIdx, RowIdx: uint32(i)}
c.hashTable.Put(key, rowPtr)
}
return nil
}
对于 rowContainer 来说,数据存放分为两部分:一部分是存放 chunk 数据到 rowContainer 的 records 或 recordsInDisk 里面;另一部分是构建 hash table 存放 key 值以及将数据的索引作为 value。
func (c *RowContainer) Add(chk *Chunk) (err error) {
...
// 如果内存已经满了,那么会写入到磁盘中
if c.alreadySpilled() {
if c.m.spillError != nil {
return c.m.spillError
}
err = c.m.recordsInDisk.Add(chk)
} else {
// 否则写入内存
c.m.records.Add(chk)
}
return
}
RowContainer 会根据内存使用量来判断是否要存磁盘还是存内存。
多线程执行 hash Join
hash Join 的过程是通过 fetchAndProbeHashTable 方法来执行的,这个方法比较有意思,向我们展示了如何在多线程中使用 chanel 进行数据传递。
func (e *HashJoinExec) fetchAndProbeHashTable(ctx context.Context) {
// 初始化数据传递的 channel
e.initializeForProbe()
e.joinWorkerWaitGroup.Add(1)
// 循环获取 ProbeSide 表中的数据,将数据存放到 probeSideResult channel中
go util.WithRecovery(func() {
defer trace.StartRegion(ctx, "HashJoinProbeSideFetcher").End()
e.fetchProbeSideChunks(ctx)
}, e.handleProbeSideFetcherPanic)
probeKeyColIdx := make([]int, len(e.probeKeys))
for i := range e.probeKeys {
probeKeyColIdx[i] = e.probeKeys[i].Index
}
// 启动多个 join workers 去buildSide表和ProbeSide 表匹配数据
for i := uint(0); i < e.concurrency; i++ {
e.joinWorkerWaitGroup.Add(1)
workID := i
go util.WithRecovery(func() {
defer trace.StartRegion(ctx, "HashJoinWorker").End()
e.runJoinWorker(workID, probeKeyColIdx)
}, e.handleJoinWorkerPanic)
}
go util.WithRecovery(e.waitJoinWorkersAndCloseResultChan, nil)
}
整个 hash Join 的执行分为三个部分:
- 由于在 hash Join 过程中是通过多线程处理的,所以会用到 channel 进行数据传递,所以第一步是调用 initializeForProbe 初始化数据传递的 channel;
- 然后会异步的调用 fetchProbeSideChunks 从 ProbeSide 表获取数据;
- 接下来会启动多个线程调用 runJoinWorker 方法启动多个 Join Worker 来进行 hash Join ;
需要注意的是,这里我们将查询probeSide表数据的线程称作 probeSideExec worker;将执行 join 匹配的线程称作 join worker,它的数量由 concurrency 决定,默认是5个。
initializeForProbe
我们先来看看 initializeForProbe:
func (e *HashJoinExec) initializeForProbe() {
// 用于probeSideExec worker保存probeSide表数据,用来给join worker做关联使用
e.probeResultChs = make([]chan *chunk.Chunk, e.concurrency)
for i := uint(0); i < e.concurrency; i++ {
e.probeResultChs[i] = make(chan *chunk.Chunk, 1)
}
// 用于将已被join workers使用过的chunks给probeSideExec worker复用
e.probeChkResourceCh = make(chan *probeChkResource, e.concurrency)
for i := uint(0); i < e.concurrency; i++ {
e.probeChkResourceCh <- &probeChkResource{
chk: newFirstChunk(e.probeSideExec),
dest: e.probeResultChs[i],
}
}
// 用于将可以重复使用的join result chunks从main thread传递到join worker
e.joinChkResourceCh = make([]chan *chunk.Chunk, e.concurrency)
for i := uint(0); i < e.concurrency; i++ {
e.joinChkResourceCh[i] = make(chan *chunk.Chunk, 1)
e.joinChkResourceCh[i] <- newFirstChunk(e)
}
// 用于将join结果chunks从 join worker传递到 main thread
e.joinResultCh = make(chan *hashjoinWorkerResult, e.concurrency+1)
}
这个方法主要就是初始化4个 channel 对象。
probeResultChs:用于保存probeSide表查出来的数据;
probeChkResourceCh:用于将已被join workers使用过的chunks给probeSideExec worker复用;
joinChkResourceCh:也是用于传递 chunks,主要是给 join worker 复用;
joinResultCh:用于传递 join worker 匹配的结果给 main thread;
fetchProbeSideChunks
下面我们再来看看异步 fetchProbeSideChunks的过程:
func (e *HashJoinExec) fetchProbeSideChunks(ctx context.Context) {
for {
...
var probeSideResource *probeChkResource
select {
case <-e.closeCh:
return
case probeSideResource, ok = <-e.probeChkResourceCh:
}
// 获取可用的 chunk
probeSideResult := probeSideResource.chk
if e.isOuterJoin {
required := int(atomic.LoadInt64(&e.requiredRows))
probeSideResult.SetRequiredRows(required, e.maxChunkSize)
}
// 获取数据存入到 probeSideResult
err := Next(ctx, e.probeSideExec, probeSideResult)
...
//将有数据的chunk.Chunk放入到dest channel中
probeSideResource.dest <- probeSideResult
}
}
在理清楚各个 channel 的作用之后就可以很容易的理解,这里主要就是获取可用的 chunk,然后调用 Next 将数据放入到 chunk 中,最后将 chunk 放入到dest channel中。
runJoinWorker
最后我们来看看 Join Worker 的实现:
func (e *HashJoinExec) runJoinWorker(workerID uint, probeKeyColIdx []int) {
...
var (
probeSideResult *chunk.Chunk
selected = make([]bool, 0, chunk.InitialCapacity)
)
// 获取 hashjoinWorkerResult
ok, joinResult := e.getNewJoinResult(workerID)
if !ok {
return
}
emptyProbeSideResult := &probeChkResource{
dest: e.probeResultChs[workerID],
}
hCtx := &hashContext{
allTypes: e.probeTypes,
keyColIdx: probeKeyColIdx,
}
// 循环获取 probeSideResult
for ok := true; ok; {
if e.finished.Load().(bool) {
break
}
select {
case <-e.closeCh:
return
// probeResultChs 里存放的是probeSideExec worker查询出来的数据
case probeSideResult, ok = <-e.probeResultChs[workerID]:
}
if !ok {
break
}
// 将join匹配的数据放入到joinResult的chunk里面
ok, joinResult = e.join2Chunk(workerID, probeSideResult, hCtx, joinResult, selected)
if !ok {
break
}
// 使用完之后,将chunk重置,重新放回 probeChkResourceCh 给probeSideExec worker使用
probeSideResult.Reset()
emptyProbeSideResult.chk = probeSideResult
e.probeChkResourceCh <- emptyProbeSideResult
}
...
}
由于 probeSideExec worker 会将数据放入到 probeResultChs 中,所以这里会循环获取它里面的数据,然后调用 join2Chunk 进行数据匹配。
func (e *HashJoinExec) join2Chunk(workerID uint, probeSideChk *chunk.Chunk, hCtx *hashContext, joinResult *hashjoinWorkerResult,
selected []bool) (ok bool, _ *hashjoinWorkerResult) {
var err error
// 校验probeSide chunk查询到的数据是否可用来匹配
selected, err = expression.VectorizedFilter(e.ctx, e.outerFilter, chunk.NewIterator4Chunk(probeSideChk), selected)
if err != nil {
joinResult.err = err
return false, joinResult
}
//probeSide表的hash,用于匹配
hCtx.initHash(probeSideChk.NumRows())
for keyIdx, i := range hCtx.keyColIdx {
ignoreNull := len(e.isNullEQ) > keyIdx && e.isNullEQ[keyIdx]
err = codec.HashChunkSelected(e.rowContainer.sc, hCtx.hashVals, probeSideChk, hCtx.allTypes[i], i, hCtx.buf, hCtx.hasNull, selected, ignoreNull)
if err != nil {
joinResult.err = err
return false, joinResult
}
}
//遍历probeSide表查询到的行记录
for i := range selected {
...
if !selected[i] || hCtx.hasNull[i] { // process unmatched probe side rows
e.joiners[workerID].onMissMatch(false, probeSideChk.GetRow(i), joinResult.chk)
} else { // process matched probe side rows
// 获取行记录的 probeKey 和 probeRow
probeKey, probeRow := hCtx.hashVals[i].Sum64(), probeSideChk.GetRow(i)
ok, joinResult = e.joinMatchedProbeSideRow2Chunk(workerID, probeKey, probeRow, hCtx, joinResult)
if !ok {
return false, joinResult
}
}
// 如果joinResult的chunk已经满了,那么将数据放入到 joinResultCh,再重新获取 joinResult
if joinResult.chk.IsFull() {
e.joinResultCh <- joinResult
ok, joinResult = e.getNewJoinResult(workerID)
if !ok {
return false, joinResult
}
}
}
return true, joinResult
}
数据匹配这里也大致分为以下几个步骤:
- 校验probeSide chunk查询到的数据是否可用来匹配;
- 获取到 probeSide chunk 的数据行进行hash,用于匹配;
- 遍历probeSide chunk表可用于匹配的数据,并调用 joinMatchedProbeSideRow2Chunk 获取匹配成功数据填入到 joinResult 中;
func (e *HashJoinExec) join2Chunk(workerID uint, probeSideChk *chunk.Chunk, hCtx *hashContext, joinResult *hashjoinWorkerResult,
selected []bool) (ok bool, _ *hashjoinWorkerResult) {
var err error
// 校验probeSide chunk查询到的数据是否可用来匹配
selected, err = expression.VectorizedFilter(e.ctx, e.outerFilter, chunk.NewIterator4Chunk(probeSideChk), selected)
if err != nil {
joinResult.err = err
return false, joinResult
}
//probeSide表的hash,用于匹配
hCtx.initHash(probeSideChk.NumRows())
for keyIdx, i := range hCtx.keyColIdx {
ignoreNull := len(e.isNullEQ) > keyIdx && e.isNullEQ[keyIdx]
err = codec.HashChunkSelected(e.rowContainer.sc, hCtx.hashVals, probeSideChk, hCtx.allTypes[i], i, hCtx.buf, hCtx.hasNull, selected, ignoreNull)
if err != nil {
joinResult.err = err
return false, joinResult
}
}
//遍历probeSide表查询到的行记录
for i := range selected {
...
if !selected[i] || hCtx.hasNull[i] { // process unmatched probe side rows
e.joiners[workerID].onMissMatch(false, probeSideChk.GetRow(i), joinResult.chk)
} else { // process matched probe side rows
// 获取行记录的 probeKey 和 probeRow
probeKey, probeRow := hCtx.hashVals[i].Sum64(), probeSideChk.GetRow(i)
// 进行数据匹配
ok, joinResult = e.joinMatchedProbeSideRow2Chunk(workerID, probeKey, probeRow, hCtx, joinResult)
if !ok {
return false, joinResult
}
}
// 如果joinResult的chunk已经满了,那么将数据放入到 joinResultCh,再重新获取 joinResult
if joinResult.chk.IsFull() {
e.joinResultCh <- joinResult
ok, joinResult = e.getNewJoinResult(workerID)
if !ok {
return false, joinResult
}
}
}
return true, joinResult
}
join2Chunk 会根据过滤条件判断 probeSide chunk 返回的数据是不是都能进行匹配,减少数据的匹配量;
如果可以匹配,那么会将 probeSide chunk 记录行的probeKey与probeRow传入到 joinMatchedProbeSideRow2Chunk 进行数据匹配。
func (e *HashJoinExec) joinMatchedProbeSideRow2Chunk(workerID uint, probeKey uint64, probeSideRow chunk.Row, hCtx *hashContext,
joinResult *hashjoinWorkerResult) (bool, *hashjoinWorkerResult) {
// 从buildSide表中匹配数据
buildSideRows, _, err := e.rowContainer.GetMatchedRowsAndPtrs(probeKey, probeSideRow, hCtx)
if err != nil {
joinResult.err = err
return false, joinResult
}
//表示没有匹配到数据,直接返回
if len(buildSideRows) == 0 {
e.joiners[workerID].onMissMatch(false, probeSideRow, joinResult.chk)
return true, joinResult
}
iter := chunk.NewIterator4Slice(buildSideRows)
hasMatch, hasNull, ok := false, false, false
// 将匹配上的数据add到 joinResult chunk 中
for iter.Begin(); iter.Current() != iter.End(); {
matched, isNull, err := e.joiners[workerID].tryToMatchInners(probeSideRow, iter, joinResult.chk)
if err != nil {
joinResult.err = err
return false, joinResult
}
if joinResult.chk.IsFull() {
e.joinResultCh <- joinResult
ok, joinResult = e.getNewJoinResult(workerID)
if !ok {
return false, joinResult
}
}
}
...
return true, joinResult
}
joinMatchedProbeSideRow2Chunk 会从 rowContainer 去获取数据,获取不到数据直接返回,获取到数据会将数据存放到 joinResult chunk 中。
下面用一个流程图来解释一下整个hash匹配过程:
整体上Join Worker匹配逻辑是:
- 从 probeSide 表获取数据到 probeSideResource;
- 根据 probeSideResource 的数据查哈希表,将 probeSide 表和buildSide表进行匹配;
- 将匹配上的数据写入到joinResult chunk 中;
- 最后将joinResult的数据刷入到 joinResultCh 发送给 Main Thread;
这篇文章基本上从构建hash join执行器开始到运行 HashJoinExec 执行器进行了一个全面的解析。
回到开头提出的问题:
-
哪张表会成为 buildSide 表或 probeSide 表?
这个是由优化器决定的,创建 Physical Plan 的时候会创建多个,然后会遍历创建的 Plan 获取它的代价最小的那个。
-
buildSide 表来构造的哈希表是包含了 buildSide 表的所有数据吗?数据量太大会不会有问题?
buildSide 表构造的 hash 表包含了所有的数据,但是TiDB这里 hash表和数据项是分离的;数据是存放到 rowContainer 的 records ,数据量太大会通过 recordsInDisk 落盘;hash表是存放到 rowContainer的hashTable中;
-
probeSide 表匹配 buildSide 表的时候是单线程匹配还是多线程匹配?如果是多线程匹配,那么如何分配匹配的数据呢?
匹配是多线程匹配的,默认concurrency是5;它们之间传递数据是通过 channel 来传递数据,各自在获取数据的时候会根据自己的线程id从 probeResultChs 数组中获取 channel 并订阅其中的数据;
Reference
https://pingcap.com/zh/blog/tidb-source-code-reading-9
https://github.com/xieyu/blog/blob/master/src/tidb/hash-join.md
Recommend
About Joyk
Aggregate valuable and interesting links.
Joyk means Joy of geeK