

[2112.02089] Regularized Newton Method with Global $O(1/k^2)$ Convergence
source link: https://arxiv.org/abs/2112.02089
Go to the source link to view the article. You can view the picture content, updated content and better typesetting reading experience. If the link is broken, please click the button below to view the snapshot at that time.
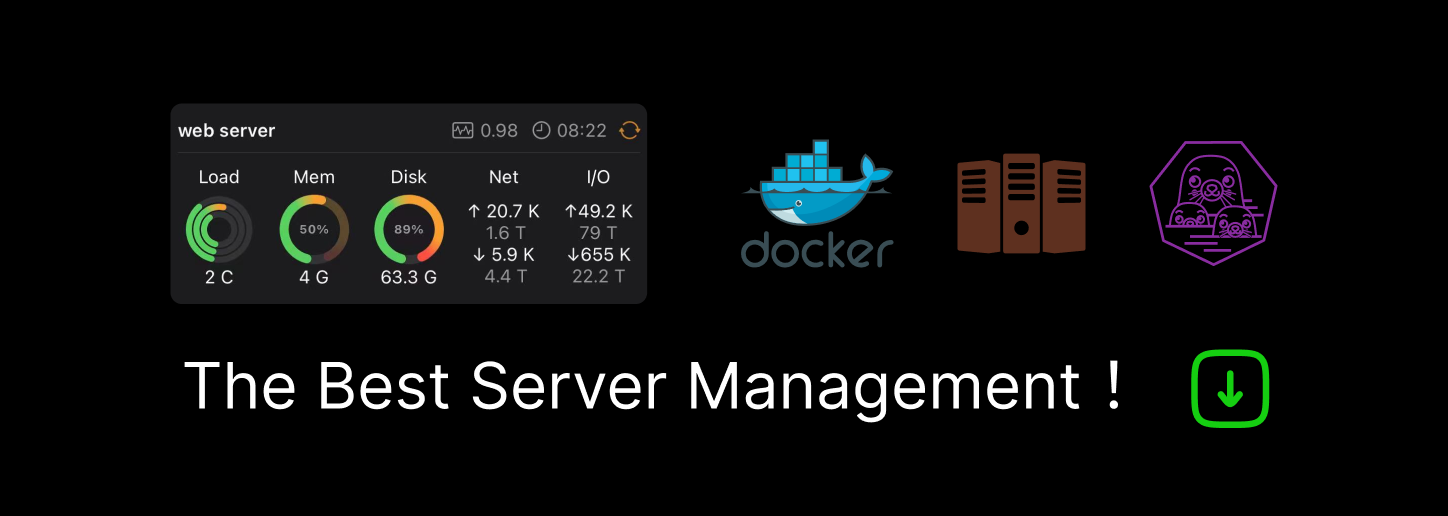
[Submitted on 3 Dec 2021]
Regularized Newton Method with Global O(1/k2) Convergence
We present a Newton-type method that converges fast from any initialization and for arbitrary convex objectives with Lipschitz Hessians. We achieve this by merging the ideas of cubic regularization with a certain adaptive Levenberg--Marquardt penalty. In particular, we show that the iterates given by xk+1=xk−(∇2f(xk)+H∥∇f(xk)∥−−−−−−−−−√I)−1∇f(xk), where H>0 is a constant, converge globally with a O(1k2) rate. Our method is the first variant of Newton's method that has both cheap iterations and provably fast global convergence. Moreover, we prove that locally our method converges superlinearly when the objective is strongly convex. To boost the method's performance, we present a line search procedure that does not need hyperparameters and is provably efficient.
Recommend
About Joyk
Aggregate valuable and interesting links.
Joyk means Joy of geeK