

Where we are headed and why it looks a lot like Julia (but not exactly like Juli...
source link: https://dev-discuss.pytorch.org/t/where-we-are-headed-and-why-it-looks-a-lot-like-julia-but-not-exactly-like-julia/276
Go to the source link to view the article. You can view the picture content, updated content and better typesetting reading experience. If the link is broken, please click the button below to view the snapshot at that time.
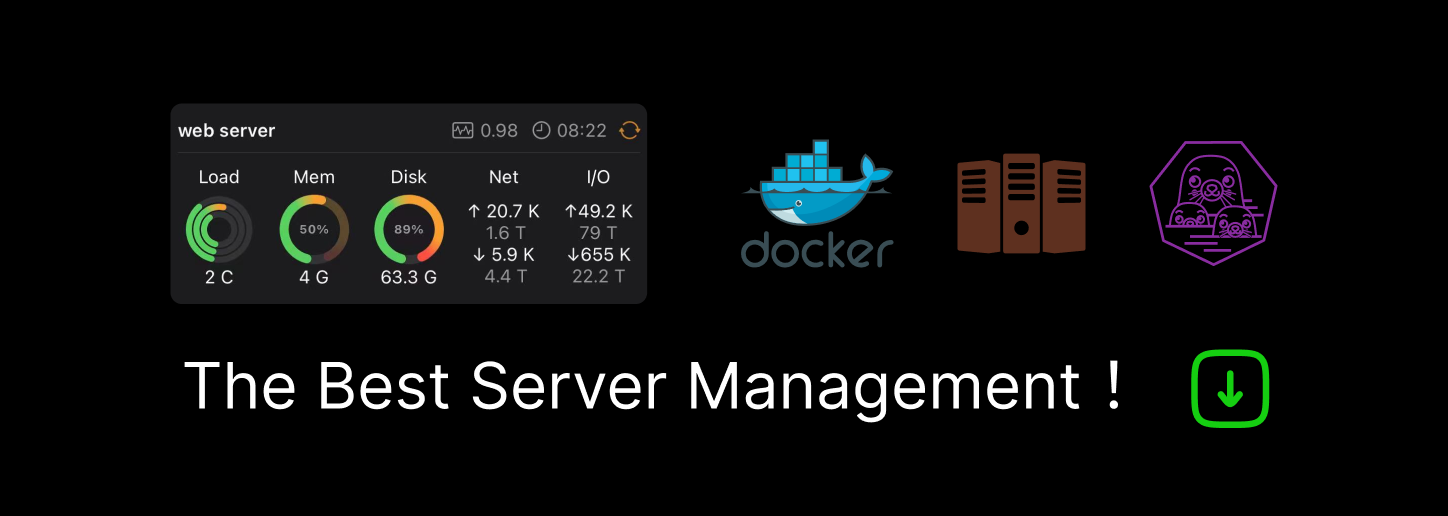
Where we are headed and why it looks a lot like Julia (but not exactly like Julia)Skip to main content
When trying to predict how PyTorch would itself get disrupted, we used to joke a bit about the next version of PyTorch being written in Julia. This was not very serious: a huge factor in moving PyTorch from Lua to Python was to tap into Python’s immense ecosystem (an ecosystem that shows no signs of going away) and even today it is still hard to imagine how a new language can overcome the network effects of Python.
However, recently, I have been thinking about various projects we have going on in PyTorch, including:
- functorch 44 - write transformations like vmap/grad directly in Python, previously only possible to do as C++ extensions to the dispatcher
- FX 18 for graph transformations, previously only possible to do as C++ TorchScript passes
- Python autograd implementation 35 for doing experimental changes to our autograd implementation, previously only possible in C++
What do all of these projects have in common? There’s some functionality that previously people could only do in C++, and the project in question makes it possible to do it in Python, increasing the hackability and ease of development. It’s important to remember that PyTorch used to be written in mostly Python, and we moved everything to C++ to make it run faster. So we are increasingly in a situation where we want to have our cake (hackability) and eat it too (performance).
This is the same story 24 that Julia has been telling for nearly a decade now. Julia says:
- A language must compile to efficient code, and we will add restrictions to the language (type stability) to make sure this is possible.
- A language must allow post facto extensibility (multiple dispatch), and we will organize the ecosystem around JIT compilation to make this possible.
- The combination of these two features gives you a system that has dynamic language level flexibility (because you have extensibility) but static language level performance (because you have efficient code)
We’ve already derived a lot of inspiration from Julia (for example, Zachary DeVito credits the original emphasis on multiple dispatch in our dispatcher to Julia), and I think in general Julia can serve as a very powerful vision of what could be possible, and also what we have to be careful about (e.g., time to first plot 82). There’s also opportunity to improve on Julia for our domain; e.g., Julia often advertises the fact that you can directly write loops with mathematical operations and have these compile into efficient code–we don’t need to try to pursue this because the cores of our kernels are quite complex and best implemented at a low level in any case.
Why not use Julia directly? We want the Julia vision, but we want it in Python (it’s the ecosystem!) There is tremendous potential in this direction, but also a lot of work and many unresolved design questions. I’m pretty excited about where we are headed next.
Credits to Gregory Chanan who has said many similar things in the past, including in his PTDC talk.
Recommend
About Joyk
Aggregate valuable and interesting links.
Joyk means Joy of geeK