

Wolves make roadways safer, generating large economic returns to predator conser...
source link: https://www.pnas.org/content/118/22/e2023251118
Go to the source link to view the article. You can view the picture content, updated content and better typesetting reading experience. If the link is broken, please click the button below to view the snapshot at that time.
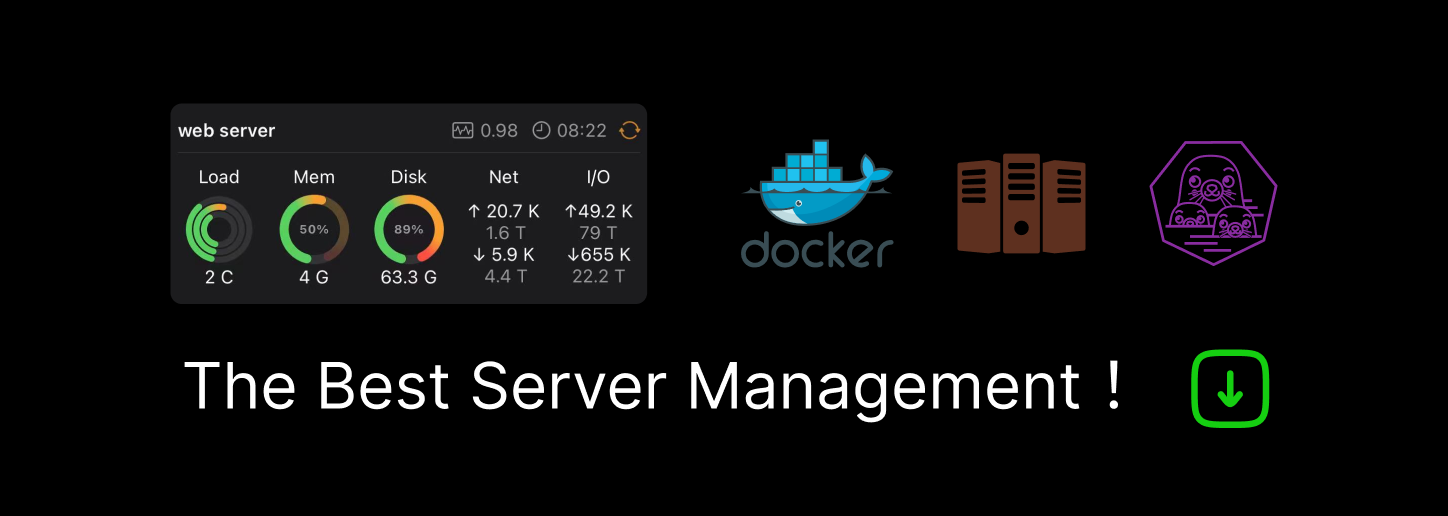
Wolves make roadways safer, generating large economic returns to predator conservation
See all authors and affiliations
Edited by Stephen R. Carpenter, University of Wisconsin–Madison, Madison, WI, and approved March 31, 2021 (received for review November 11, 2020)
Significance
Measuring the economic benefits conveyed by predators is difficult—often, effects are indirect and operate through complex ecological changes. As a result, debates about the expansion of predators have pit salient costs against more speculative estimates of benefits that might be dismissed as unreliable or ideologically motivated. We quantify the indirect benefits of wolves (Canis lupus) to human lives and property through reductions in deer-vehicle collisions. Moreover, we decompose the effect into two components: changes in prey behavior versus prey abundance. This decomposition is important when effective policy depends on whether hunters can replicate the effects of predators. In the case of wolves, we conclude that human deer hunters cannot.
Abstract
Recent studies uncover cascading ecological effects resulting from removing and reintroducing predators into a landscape, but little is known about effects on human lives and property. We quantify the effects of restoring wolf populations by evaluating their influence on deer–vehicle collisions (DVCs) in Wisconsin. We show that, for the average county, wolf entry reduced DVCs by 24%, yielding an economic benefit that is 63 times greater than the costs of verified wolf predation on livestock. Most of the reduction is due to a behavioral response of deer to wolves rather than through a deer population decline from wolf predation. This finding supports ecological research emphasizing the role of predators in creating a “landscape of fear.” It suggests wolves control economic damages from overabundant deer in ways that human deer hunters cannot.
Footnotes
- ↵1To whom correspondence may be addressed. Email: [email protected].
Author contributions: J.L.R., C.A.G., and D.P.P. designed research; J.L.R., C.A.G., and D.P.P. performed research; J.L.R. and D.P.P. analyzed data; J.L.R., C.A.G., and D.P.P. wrote the paper; J.L.R. conceptualized the study and collected and curated the data; and D.P.P. acquired financial support for the project.
Competing interest statement: S.R.C., C.A.G., and D.P.P. are affiliated with University of Wisconsin–Madison. C.A.G. and D.P.P. did not request S.R.C. as an editor, and S.R.C. did not invite submission of this article.
This article is a PNAS Direct Submission.
This article contains supporting information online at https://www.pnas.org/lookup/suppl/doi:10.1073/pnas.2023251118/-/DCSupplemental.
Data Availability
The datasets generated during and/or analyzed during the current study are available in Dryad at https://doi.org/10.5061/dryad.g4f4qrfp8. This paper does not use any custom algorithms.
Published under the PNAS license.
References
- ↵
- J. A. Estes et al
- ↵
- W. J. Ripple et al
- ↵
- B. E. McLaren,
- R. O. Peterson
- ↵
- W. J. Ripple,
- E. J. Larsen,
- R. A. Renkin,
- D. W. Smith
- ↵
- R. Callan,
- N. P. Nibbelink,
- T. P. Rooney,
- J. E. Wiedenhoeft,
- A. P. Wydeven
- ↵
- J.-L. Martin,
- S. Chamaillé-Jammes,
- D. M. Waller
- ↵
- S. Gilbert,
- N. Carter,
- R. Naidoo
- ↵
- J. A. Estes,
- L. P. Carswell
- ↵
- E. J. Gregr et al
- ↵
- L. Boitani
- ↵
- G. Chapron et al
- ↵
- US Fish and Wildlife Service
- ↵
- ↵
- C. M. Chambers,
- J. C. Whitehead
- ↵
- C. L. Kling,
- D. J. Phaneuf,
- J. Zhao
- ↵
- W. S. Alverson,
- D. M. Waller,
- S. L. Solheim
- ↵
- E. S. Long,
- D. R. Diefenbach,
- C. S. Rosenberry,
- B. D. Wallingford,
- M. D. Grund
- ↵
- A. Leopold,
- L. K. Sowls,
- D. L. Spencer
- ↵
- S. D. Côté,
- T. P. Rooney,
- J.-P. Tremblay,
- C. Dussault,
- D. M. Waller
- ↵
- K. M. Averill et al
- ↵
- M. R. Conover,
- W. C. Pitt,
- K. K. Kessler,
- T. J. DuBow,
- W. A. Sanborn
- ↵
- M. P. Huijser et al
- ↵
- G. W. T. A. Groot Bruinderink,
- E. Hazebroek
- ↵
- W. J. Ripple,
- R. L. Beschta
- ↵
- F. Messier
- ↵
- A. J. DeNicola,
- S. C. Williams
- ↵
- A. Hussain,
- J. B. Armstrong,
- D. B. Brown,
- J. Hogland
- ↵
- S. L. Gilbert, et al
- ↵
- J. L. Atkins et al
- ↵
- J. W. Laundré,
- L. Hernández
- ↵
- K. M. Gaynor,
- J. S. Brown,
- A. D. Middleton,
- M. E. Power,
- J. S. Brashares
- ↵
- J. Whittington et al
- ↵
- M. Dickie,
- R. Serrouya,
- R. S. McNay,
- S. Boutin
- ↵
- A. R. C. James,
- A. K. Stuart-Smith
- ↵
- K. Bojarska et al
- ↵
- E. J. Newton et al
- ↵
- C. A. DeMars,
- S. Boutin
- ↵
- J. A. Dellinger et al
- ↵
- Wisconsin Department of Natural Resources
- ↵
- L. D. Mech
- ↵
- J. E. Wiedenhoeft,
- D. M. MacFarland,
- N. S. Libal,
- J. Bruner
- ↵
- T. R. Van Deelen
- ↵
- National Archives and Records Administration
- ↵
- D. J. Mladenoff,
- T. A. Sickley
- ↵
- Wisconsin Department of Natural Resources
- ↵
- Wisconsin Department of Natural Resources
- ↵
- J. D. Angrist,
- J.-S. Pischke
- ↵
- A. Olden,
- J. Moen
- ↵
- T. L. Brown et al
- ↵
- T. Crocker,
- J. Tschirhart
- ↵
- P. J. Baker,
- L. Boitani,
- S. Harris,
- G. Saunders,
- P. C. White
- ↵
- I. Laporte,
- T. B. Muhly,
- J. A. Pitt,
- M. Alexander,
- M. Musiani
- ↵
- J. R. Steele,
- B. S. Rashford,
- T. K. Foulke,
- J. A. Tanaka,
- D. T. Taylor
- ↵
- M. Widman,
- M. Steen,
- K. Elofsson
- ↵
- L. Naughton-Treves,
- R. Grossberg,
- A. Treves
- ↵
- J. F. Shogren et al
- ↵
- A. W. Ando
- ↵
- ↵
- M. P. Huijser,
- J. W. Duffield,
- A. P. Clevenger,
- R. J. Ament,
- P. T. McGowen
- ↵
- W. J. Hans,
- N. Venkateswaran,
- V. S. Solomi
- ↵
- J. Chen et al
- ↵
- T. L. DeVault,
- T. W. Seamans,
- B. F. Blackwell
- ↵
- A. P. Wywialowski
- ↵
- J. S. Mao et al
- ↵
- D. Fortin et al
- ↵
- Wisconsin Department of Natural Resources
- ↵
- J. Yoder
- ↵
- T. Levi,
- A. M. Kilpatrick,
- M. Mangel,
- C. C. Wilmers
- ↵
- H. J. Kilpatrick,
- A. M. LaBonte,
- K. C. Stafford
- ↵
- E. R. Adrion,
- J. Aucott,
- K. W. Lemke,
- J. P. Weiner
- ↵
- K. Berry,
- J. Bayham,
- S. R. Meyer,
- E. P. Fenichel
- ↵
- T. R. Van Deelen et al
- ↵
- D. R. Diefenbach,
- W. L. Palmer,
- W. K. Shope
- ↵
- B. Blossey,
- P. Curtis,
- J. Boulanger,
- A. Dávalos
- ↵
- M. Fuller
- ↵
- US Department of the Interior
- ↵
- S. Ciuti et al
- ↵
- J. C. Kilgo,
- R. F. Labisky,
- D. E. Fritzen
- ↵
- ↵
- H. L. Beyer,
- E. H. Merrill,
- N. Varley,
- M. S. Boyce
- ↵
- W. J. Ripple,
- E. J. Larsen
- ↵
- W. J. Ripple,
- R. L. Beschta
- ↵
- E. Marris
- ↵
- F. Messier
- ↵
- L. L. Eberhardt,
- R. A. Garrott,
- D. W. Smith,
- P. J. White,
- R. O. Peterson
- ↵
- J. A. Vucetich,
- R. O. Peterson
- ↵
- J. A. Vucetich,
- M. Hebblewhite,
- D. W. Smith,
- R. O. Peterson
- ↵
- R. P. Thiel
- ↵
- D. J. Mladenoff,
- T. A. Sickley,
- R. G. Haight,
- A. P. Wydeven
- ↵
- D. J. Mladenoff,
- T. A. Sickley,
- A. P. Wydeven
- ↵
- B. E. Kohn,
- E. M. Anderson,
- R. P. Thiel
- ↵
- A. P. Wydeven et al
- ↵
- R. Michener,
- C. Tighe
- ↵
- S. D. Levitt,
- J. Porter
- ↵
- M. R. Jacobsen
- ↵, Traffic safety and vehicle choice: Quantifying the effects of the ‘arms race’ on American roads. J. Appl. Econ. 27, 34–62 (2012).
- ↵
- A. C. Smith
- ↵
- L. Blincoe,
- T. R. Miller,
- E. Zaloshnja,
- B. A. Lawrence,
- ↵
- National Highway Traffic Safety Administration
- ↵
- R. E. Allen,
- D. R. McCullough
- ↵
- T. L. Bashore,
- W. M. Tzilkowski,
- E. D. Bellis
- ↵
- A. P. Clevenger,
- M. Barrueto,
- K. E. Gunson,
- F. M. Caryl,
- A. T. Ford
- ↵
- M. C. Farrell,
- P. A. Tappe
- ↵
- R. A. Finder,
- J. L. Roseberry,
- A. Woolf
- ↵
- R. Found,
- M. S. Boyce
- ↵
- K. Gkritza,
- R. R. Souleyrette,
- M. J. Baird,
- B. J. Danielson
- ↵
- K. Gkritza,
- M. Baird,
- Z. N. Hans
- ↵
- T. W. Grovenburg et al
- ↵
- T. Hothorn,
- J. Müller,
- L. Held,
- L. Möst,
- A. Mysterud
- ↵
- M. W. Hubbard,
- B. J. Danielson,
- R. A. Schmitz
- ↵
- Y. Lao,
- G. Zhang,
- Y.-J. Wu,
- Y. Wang
- ↵
- J. J. Mckee,
- D. M. Cochran
- ↵
- W. J. McShea,
- C. M. Stewart,
- L. J. Kearns,
- S. Liccioli,
- D. Kocka
- ↵
- J. W. Ng,
- C. Nielson,
- C. C. St Clair
- ↵
- C. K. Nielsen,
- R. G. Anderson,
- M. D. Grund
- ↵
- K. Sudharsan,
- S. J. Riley,
- H. Campa III
- ↵
- J. Aguero-Valverde,
- P. P. Jovanis
- ↵
- M.-P. Andreescu,
- D. B. Frost
- ↵
- J. Andrey,
- S. Yagar
- ↵
- J. Bertness
- ↵
- D. Eisenberg
- ↵
- L. Fridstrøm,
- J. Ifver,
- S. Ingebrigtsen,
- R. Kulmala,
- L. K. Thomsen
- ↵
- B. Leard,
- K. Roth
- ↵
- N. Levine,
- K. E. Kim,
- L. H. Nitz
- ↵
- N. V. Malyshkina,
- F. L. Mannering
- ↵
- V. Shankar,
- F. Mannering,
- W. Barfield
- ↵
- L. A. Sherretz,
- B. C. Farhar
- ↵
- J. Biggs,
- S. Sherwood,
- S. Michalak,
- L. Hansen,
- C. Bare
- ↵
- C. M. Rolandsen,
- E. J. Solberg,
- I. Herfindal,
- B. Van Moorter,
- B.-E. Sæther
- ↵
- A. Mysterud
- ↵
- K. L. Parker,
- C. T. Robbins
- ↵
- K. E. Gunson,
- G. Mountrakis,
- L. J. Quackenbush
- ↵
- A. Treves,
- K. A. Martin,
- A. P. Wydeven,
- J. E. Wiedenhoeft
- ↵
- A. Treves,
- M. F. Rabenhorst
- ↵
- A. L. Iverson,
- L. R. Iverson
- ↵
- J. Raynor
- ↵
- K. A. Schwabe,
- P. W. Schuhmann,
- M. J. Tonkovich,
- ↵
- A. Seiler
- ↵
- Wisconsin Department of Natural Resources
- ↵2011 Wisconsin Act 69 (October 7, 2020).
- ↵
- A. P. Wydeven,
- J. E. Wiedenhoeft,
- R. N. Schultz,
- J. Bruner,
- S. Boles
- ↵
- Wisconsin Department of Natural Resources
- ↵
- B. Dennis,
- M. R. Otten
- ↵
- T. M. Hegel et al
- ↵
- US Department of Transportation
Log in using your username and password
Log in through your institution
Purchase access
Subscribers, for more details, please visit our Subscriptions FAQ.
Please click here to log into the PNAS submission website.
Article Classifications
- Social Sciences
- Economic Sciences
You May Also be Interested in
Recommend
About Joyk
Aggregate valuable and interesting links.
Joyk means Joy of geeK