
4

美团搜索多业务商品排序探索与实践
source link: https://tech.meituan.com/2021/11/19/exploration-and-practice-of-multi-business-commodities-ranking-in-meituan-search.html
Go to the source link to view the article. You can view the picture content, updated content and better typesetting reading experience. If the link is broken, please click the button below to view the snapshot at that time.
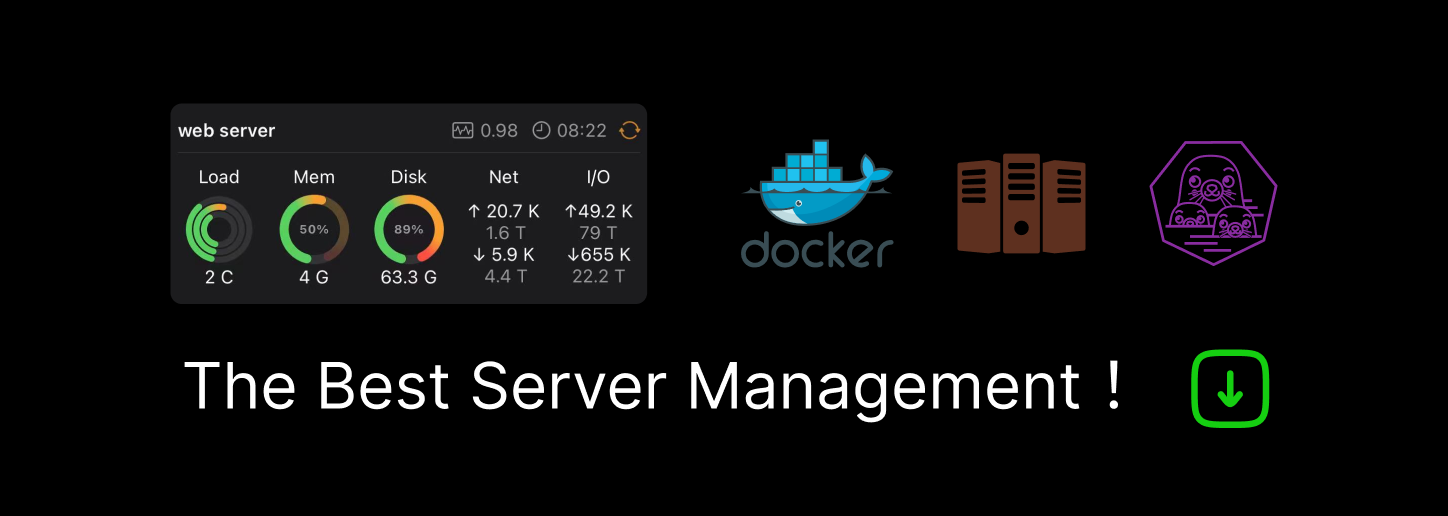
美团搜索多业务商品排序探索与实践
2021年11月19日
作者: 曹越 瑶鹏 诗晓 李想等
文章链接
3182字
7分钟阅读
- [1] 多业务建模在美团搜索排序中的实践
- [2] Ma X, Zhao L, Huang G, et al. Entire space multi-task model: An effective approach for estimating post-click conversion rate[C]//The 41st International ACM SIGIR Conference on Research & Development in Information Retrieval. 2018: 1137-1140.
- [3] Friedman et al., A note on the group lasso and a sparse group lasso.
- [4] Kendall et al., Multi-Task Learning Using Uncertainty to Weigh Losses for Scene Geometry and Semantics. In CVPR, 2018.
- [5] Guo et al., Dynamic Task Prioritization for Multitask Learning. In ECCV, 2018.
- [6] Sheng et al., One Model to Serve All: Star Topology Adaptive Recommender for Multi-Domain CTR Prediction. In CIKM, 2021.
- [7] Zhou G, Zhu X, Song C, et al. Deep interest network for click-through rate prediction[C]//Proceedings of the 24th ACM SIGKDD International Conference on Knowledge Discovery & Data Mining. ACM, 2018: 1059-1068.
- [8] Zhou G, Mou N, Fan Y, et al. Deep interest evolution network for click-through rate prediction[C]//Proceedings of the AAAI Conference on Artificial Intelligence. 2019, 33: 5941-5948.
- [9] Feng Y, Lv F, Shen W, et al. Deep Session Interest Network for Click-Through Rate Prediction[J]. arXiv preprint arXiv:1905.06482, 2019.
- [10] Chen Q, Zhao H, Li W, et al. Behavior sequence transformer for e-commerce recommendation in Alibaba[C]//Proceedings of the 1st International Workshop on Deep Learning Practice for High-Dimensional Sparse Data. 2019: 1-4
- [11] Kan Ren, Jiarui Qin, Yuchen Fang, Weinan Zhang, Lei Zheng, Weijie Bian, Guorui Zhou, Jian Xu, Yong Yu, Xiaoqiang Zhu, et al. Lifelong sequential modeling with personalized memorization for user response prediction. In SIGIR, 2019.
- [12] Qi Pi, Weijie Bian, Guorui Zhou, Xiaoqiang Zhu, and Kun Gai. Practice on long sequential user behavior modeling for click-through rate prediction. In KDD, 2019.
- [13] Jiarui Qin, W. Zhang, Xin Wu, Jiarui Jin, Yuchen Fang, and Y. Yu. User behavior retrieval for click-through rate prediction. In SIGIR, 2020.
- [14] Search-based User Interest Modeling with Lifelong Sequential Behavior Data for Click-Through Rate Prediction.
- [15] Transformer 在美团搜索排序中的实践
- [16] Ma J, Zhao Z, Yi X, et al. Modeling task relationships in multi-task learning with multi-gate mixture-of-experts[C]//Proceedings of the 24th ACM SIGKDD International Conference on Knowledge Discovery & Data Mining. 2018: 1930-1939.
- [17] Xi D, Chen Z, Yan P, et al. Modeling the Sequential Dependence among Audience Multi-step Conversions with Multi-task Learning in Targeted Display Advertising[J]. arXiv preprint arXiv:2105.08489, 2021.
- [18] Burges C J C. From ranknet to lambdarank to lambdamart: An overview[J]. Learning, 2010, 11(23-581): 81.
- [19] https://en.wikipedia.org/wiki/Huber_loss
- [20] Liu et al., AutoFIS: Automatic Feature Interaction Selection in Factorization Models for Click-Through Rate Prediction, In ADS-KDD, 2020.
- [21] Khawar et al., AutoFeature: Searching for Feature Interactions and Their Architectures for Click-through Rate Prediction, In CIKM, 2020.
- [22] Tang et al., Progressive Layered Extraction (PLE): A Novel Multi-Task Learning (MTL) Model for Personalized Recommendations, In Recsys, 2020.
曹越、瑶鹏、诗晓、李想、家琪、可依、晓江、肖垚、培浩、达遥、陈胜、云森、利前均来自美团平台搜索与 NLP 部。
Recommend
About Joyk
Aggregate valuable and interesting links.
Joyk means Joy of geeK