

【NLP】文本分类
source link: https://www.guofei.site/2021/10/01/nlp_classify.html
Go to the source link to view the article. You can view the picture content, updated content and better typesetting reading experience. If the link is broken, please click the button below to view the snapshot at that time.
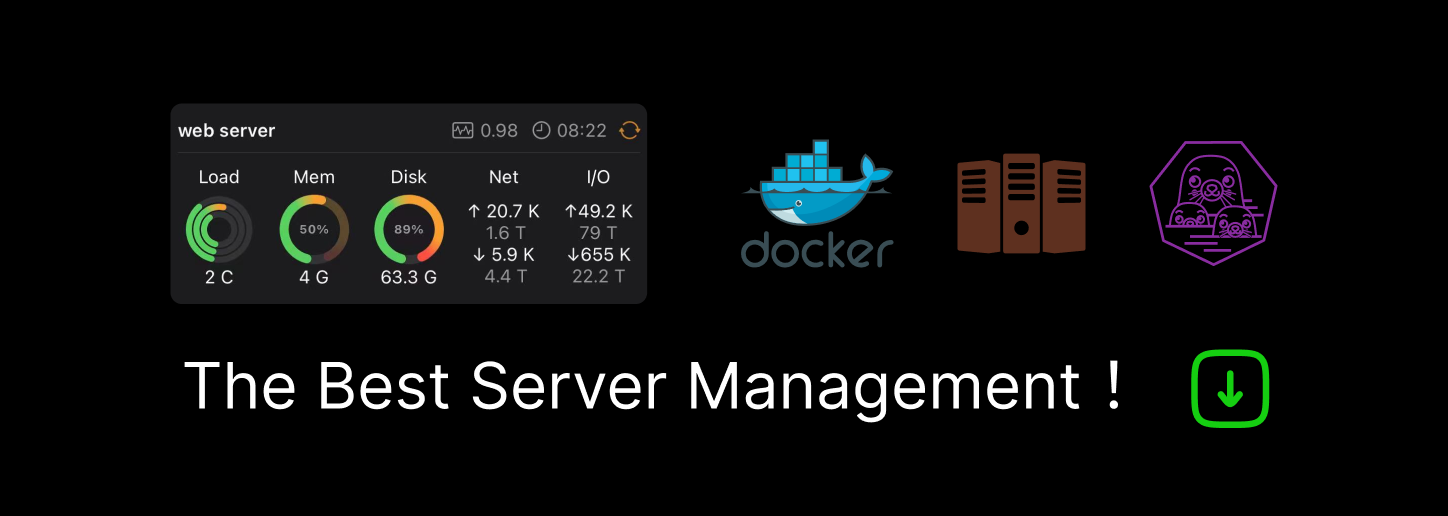
【NLP】文本分类
2021年10月01日Author: Guofei
文章归类: 2-4-NLP ,文章编号: 341
版权声明:本文作者是郭飞。转载随意,但需要标明原文链接,并通知本人
原文链接:https://www.guofei.site/2021/10/01/nlp_classify.html
方法
就完事了。
这里记一些其它要点。
方法的选择
第一步一般用 TfidfVectorizer
,这加入了 idf 信息,一般效果会更好。
如果第二步用 MultinomialNB
,它要求特征是离散的,第一步就只能用 CountVectorizer
特征重要性
如果第二步用树模型,就有对应的 feature_importance,可以看出是哪个词作为分类关键特征。
- 其它模型都没有这个功能。
- 缺点是:只能看到哪个词有关键 “分割” 作用,看不出哪个词导致分到哪个组。(例如,在做情感分析时,“好”,“不好” 可能都有关键的分割作用)
如果第二步用 MultinomialNB
,由于有 feature_log_prob_ ,所以可以用来看特征。
feature_log_prob_ 其实 是 P(xi∣y)P(xi∣y) 的对数,我们希望看到 P(xi∣y=0)/P(xi∣y=1)P(xi∣y=0)/P(xi∣y=1) 的最大值和最小值,因此就有以下代码:
feature_importance = best_model.feature_log_prob_[0, :] - best_model.feature_log_prob_[1, :]
feature_sort = feature_importance.argsort()
feature_names = count_vectorizer.get_feature_names()
for idx in feature_sort[:10]:
print(feature_names[idx], feature_importance[idx])
for idx in feature_sort[-10:]:
print(feature_names[idx], feature_importance[idx])
两类特征分别打印出来是:
非常感谢 -3.9134764940561295 大赞 -3.856318080216181 超好 -3.7956934583997466 稻香村 -3.7956934583997466 超快 -3.753133843980951 又快又好 -3.7311549372621755 很赞 -3.7311549372621755 给力 -3.662162065775224 赞赞赞 -3.662162065775224 棒棒 -3.5880540936215013
将近 2.984228449072506 差劲 3.1040296488851267 显示 3.1200299902315676 再也不会 3.151282533735672 投诉 3.151282533735672 凉皮 3.1815878832310007 迟到 3.1815878832310007 告诉 3.2253905058893944 米线 3.358921898513916 差评 4.5678822443508915
可以看出,特征还是很显著的。
您的支持将鼓励我继续创作!
Recommend
About Joyk
Aggregate valuable and interesting links.
Joyk means Joy of geeK