

How Hugging Face is tackling bias in NLP
source link: https://venturebeat.com/2021/08/25/how-hugging-face-is-tackling-bias-in-nlp/
Go to the source link to view the article. You can view the picture content, updated content and better typesetting reading experience. If the link is broken, please click the button below to view the snapshot at that time.
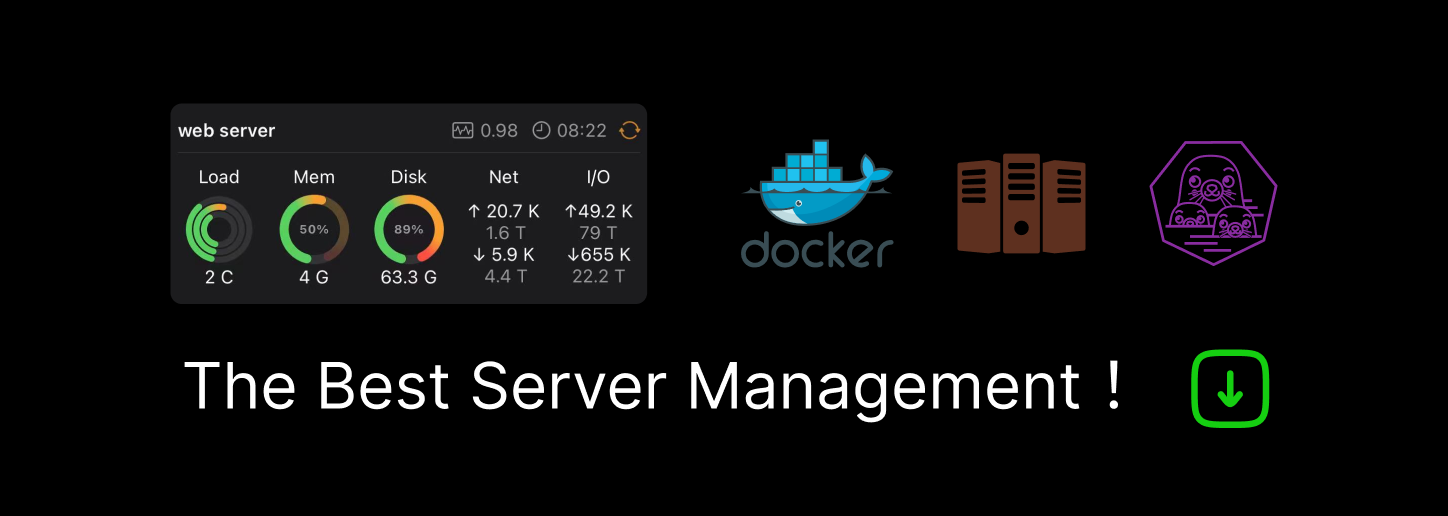
How Hugging Face is tackling bias in NLP
How open API standards will transform financial services
Open standards will have a huge impact on driving innovation in banking. Learn the status in the U.S. – and the bold new opportunities open standards are set to usher in.
Register hereThe Transform Technology Summits start October 13th with Low-Code/No Code: Enabling Enterprise Agility. Register now!
Given that natural language processing (NLP) is a subset of artificial intelligence (AI), models need to train on large volumes of data. Unfortunately, many researchers are unable to access or develop the models and datasets necessary for robust systems — they are mostly the purview of large technology giants.
Hugging Face, the winner of VentureBeat’s Innovation in Natural Language Process/Understanding Award for 2021, is looking to level the playing field. The team, launched by Clément Delangue and Julien Chaumond in 2016, was recognized for its work in democratizing NLP, the global market value for which is expected to hit $35.1 billion by 2026. This week, Google’s former head of Ethical AI Margaret Mitchell joined the team.
There are many reasons to democratize access to NLP, says Alexander (Sasha) Rush, associate professor at Cornell University and a researcher at Hugging Face. In addition to the technology being shaped and developed by just a few large tech companies, the language can be overly focused on English, he pointed out in an email interview. Also, “text data can be particularly sensitive to privacy or security concerns,” Rush said, “users often want to run their own version of a model,” he added.
Today, Hugging Face has expanded to become a robust NLP startup, known primarily for making open-source software such as Transformers and Datasets, used for building NLP systems. “The software Hugging Face develops can be used for classification, question answering, translation, and many other NLP tasks,” Rush said. Hugging Face also hosts a range of pretrained NLP models, on GitHub, that practitioners can download and apply for their problems, Rush added.
The datasets challenge
One of the many projects that Hugging Face works on, is related to datasets. Given that datasets are essential to NLP — “Every system from translation to question answering to dialogue starts with a dataset for training and evaluation,” Rush said — their numbers have been growing.
“As NLP systems have started to become more accurate there has been a large growth in the number and size of datasets produced by the NLP community, both by academics and community practitioners,” Rush pointed out. According to Rush, chief scientist Thomas Wolf developed Datasets “to help standardize the distribution, documentation and versioning of these datasets, while also making them easy and efficient to access.”
Hugging Face’s Datasets project is a community library of natural language processing, which has collected 650 unique datasets from more than 250 global contributors. Datasets has facilitated a large variety of research projects. “In particular we are seeing new use cases where users run the same system across dozens of different datasets to test generalization of models and robustness on new tasks. For instance, models like OpenAI’s GPT-3 use a benchmark of many different tasks to test ability to generalize, a style of benchmarking that Datasets makes possible and easy to do,” Rush said.
Addressing diversity and bias
Datasets is just one of the many projects Hugging Face is working on; the startup also tackles larger questions related to the field of AI. To address the challenge of increasing diversity of language-related datasets, the startup is making adding datasets as easy as possible so that any community member can do so, Rush said. Hugging Face is also hosting joint community events with interest groups such as Bengali NLP and Masakhane, a grassroots NLP community for Africa.
Bias in AI datasets is a known problem, and Hugging Face is tackling the challenge by strongly encouraging users to write extensive documentation, including known biases, when they add a dataset. Hugging Face provides users with a template and guide for this process. “We do sometimes ask users to reconsider adding datasets if they are problematic,” said Yacine Jernite, a research scientist at Hugging Face, via email. “This has only happened in rare cases and through a direct conversation with the person who suggested the dataset.” In one instance, a community member was looking to add problematic jokes from Reddit, so Hugging Face talked to the user, who took them down.
Hugging Face is also knee-deep in a project called BigScience, an international, multi-company, multi-university research project with over 500 researchers, designed to better understand and improve results on large language models. “The project is multi-faceted as well, incorporating both engineering aspects of how to produce larger, more accurate models with groups studying social and environmental impact and data governance,” Rush said.
VentureBeat
VentureBeat's mission is to be a digital town square for technical decision-makers to gain knowledge about transformative technology and transact.
Our site delivers essential information on data technologies and strategies to guide you as you lead your organizations. We invite you to become a member of our community, to access:
- up-to-date information on the subjects of interest to you
- our newsletters
- gated thought-leader content and discounted access to our prized events, such as Transform 2021: Learn More
- networking features, and more
Virtual financial assistants: What’s taking so long?

How open API standards will transform financial services
Open standards will have a huge impact on driving innovation in banking. Learn the status in the U.S. – and the bold new opportunities open standards are set to usher in.
Register herePresented by Abe.ai
For those of us that are regulars at fintech and artificial intelligence (AI) conferences and follow the development and innovation around AI, virtual financial assistants (VFAs) have gotten a lot of attention at these events for a few years now. But lately it seems that VFAs might be becoming old news — conferences have started moving onto other topics after focusing on conversational AI for several years in a row.
So the questions many people have are: “What’s taking so long? Where are these VFAs? Why doesn’t my bank have one yet?”
Virtual financial assistants take time to build
The hype around VFAs and their benefits the last few years may seem new but it is not, it just got louder. We have seen plenty of primitive iterations of chatbots over the last decade promising similar benefits, but in 2018, Bank of America actually delivered.
They came out with what some called the first “full-featured” VFA, “Erica,” delivering personalized, timely advice and assistance. “Erica” has enjoyed tremendous success (55% adoption as of February 2021), but they were very much on the bleeding edge of this technology when it comes to financial institutions (FIs). They invested multiple years, a large team and a chunk of their technology budget — something not many FIs have been willing to do.
A chatbot in 2014 felt stale and rigid using decision trees to guide you, while the advanced natural language processing/understanding technology of today that we and some others like Bank of America use to build a VFA, is significantly more complex. It requires a team of expert data scientists and several years of development.
While FIs execute their VFA project, it will take time, and how much time depends on the path they choose — build versus buy or a combination of both.
Where the financial institution focuses matters
While many conversational banking projects with FIs often started with every VFA functionality on the table (some combination of customer service, transactions, product offerings, financial insights, etc.), things changed over the past 18 months.
As FI’s budgets were strained, conversations with technology decision-makers quickly focused on cost-cutting features. So while full-featured VFAs may be on the roadmaps of most mid- to large-tier FIs, many are deploying an initial phase with service functionality first, and that is a strategy we have seen work well and recommend.
While a VFA focused solely on assisting the service center is not glamorous, it has been vital in reducing service center costs at many FIs at a time when many were seeing peak demand during the pandemic.
You may not see the VFA pop up in your app with helpful advice on what or when to do it just yet, but many FIs do have the VFAs in the help section of the app, on IVR, or even helping the agent you are talking with get the answer you need.
While FIs execute their VFA projects, branching out into the other functions beyond assisting the service center will take time — how much depends on when technology budgets loosen with a broader focus on innovation again.
The VFA is the mouthpiece, but you need the data
While the perceived delay in next generation VFAs can be chalked up to development time of advanced technologies and the high prioritization of cost-cutting service functionality, there is another factor keeping the hype of full-featured VFAs from realizing full potential.
The VFA may be the perfect delivery method for service, advice, and the nudge towards financial wellness, but without accurate data and timely insights to trigger those conversations, the experience is significantly less powerful. Delivering a personalized conversation to a customer with general information, even if it contains their authenticated data, is not enough.
What makes a personalized conversation with a VFA engaging is the feeling that your FI knows you, your habits, and what you need. Then having the tools to not only engage through a personalized conversation, but also take action directly in the VFA session as if you were speaking to a personal banker.
In order to do this, an FI needs to have their data house in order and work with vendors who can help enrich the data and deliver valuable insights. Once this need for the right data/insights becomes clear to FIs looking to deliver a full-featured VFA, this data work sometimes gets prioritized, extensively delaying the VFA implementation. While FIs execute their VFA projects, they will need to do significant work on their data and insights capability in order to deliver a real full-featured VFA, and that will take time.
So when asking what’s taking so long for you to get a full-featured VFA at your FI, it’s likely that your bank did build or has been building for some time now. We often see FIs come to us after spending two or three years building on their own, looking to partner now to supplement that work or even start over. So, while it still might be some time before you see a full-featured VFA, the good news is more FIs are recognizing the importance of partnering with a conversational AI provider and yep, you guessed it, that will take time.
Download The Next Generation of Conversational Banking to learn how financial institutions can leverage conversational artificial intelligence to go beyond simple reactive use cases and instead generate proactive interactions that engage customers on meaningful money matters and support financial wellness.
Juan Romera is Product Evangelist at Abe.ai (an Envestnet | Yodlee solution).
Sponsored articles are content produced by a company that is either paying for the post or has a business relationship with VentureBeat, and they’re always clearly marked. Content produced by our editorial team is never influenced by advertisers or sponsors in any way. For more information, contact [email protected].
Recommend
About Joyk
Aggregate valuable and interesting links.
Joyk means Joy of geeK