

[2006.10803] Supervision Accelerates Pre-training in Contrastive Semi-Supervised...
source link: https://arxiv.org/abs/2006.10803
Go to the source link to view the article. You can view the picture content, updated content and better typesetting reading experience. If the link is broken, please click the button below to view the snapshot at that time.
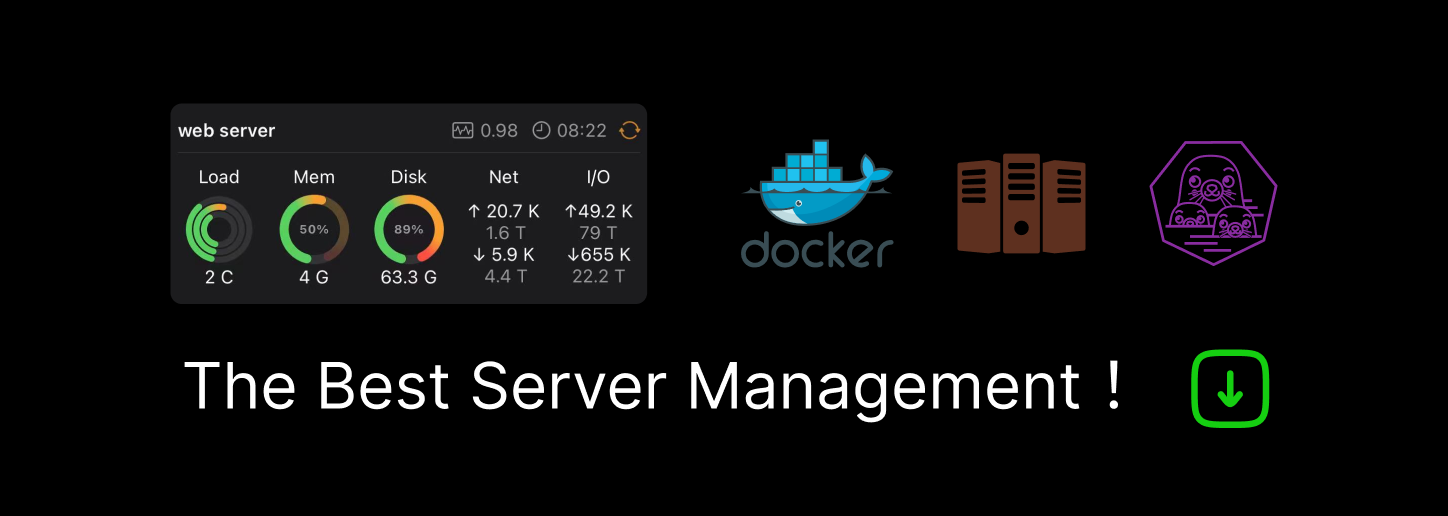
[Submitted on 18 Jun 2020 (v1), last revised 1 Dec 2020 (this version, v2)]
Supervision Accelerates Pre-training in Contrastive Semi-Supervised Learning of Visual Representations
We investigate a strategy for improving the efficiency of contrastive learning of visual representations by leveraging a small amount of supervised information during pre-training. We propose a semi-supervised loss, SuNCEt, based on noise-contrastive estimation and neighbourhood component analysis, that aims to distinguish examples of different classes in addition to the self-supervised instance-wise pretext tasks. On ImageNet, we find that SuNCEt can be used to match the semi-supervised learning accuracy of previous contrastive approaches while using less than half the amount of pre-training and compute. Our main insight is that leveraging even a small amount of labeled data during pre-training, and not only during fine-tuning, provides an important signal that can significantly accelerate contrastive learning of visual representations. Our code is available online at this http URL.
Recommend
About Joyk
Aggregate valuable and interesting links.
Joyk means Joy of geeK