

The UK A-Level ‘COVID-19 algorithm fiasco’ and lessons for the enterprise
source link: https://diginomica.com/uk-level-covid-19-algorithm-fiasco-and-lessons-enterprise
Go to the source link to view the article. You can view the picture content, updated content and better typesetting reading experience. If the link is broken, please click the button below to view the snapshot at that time.
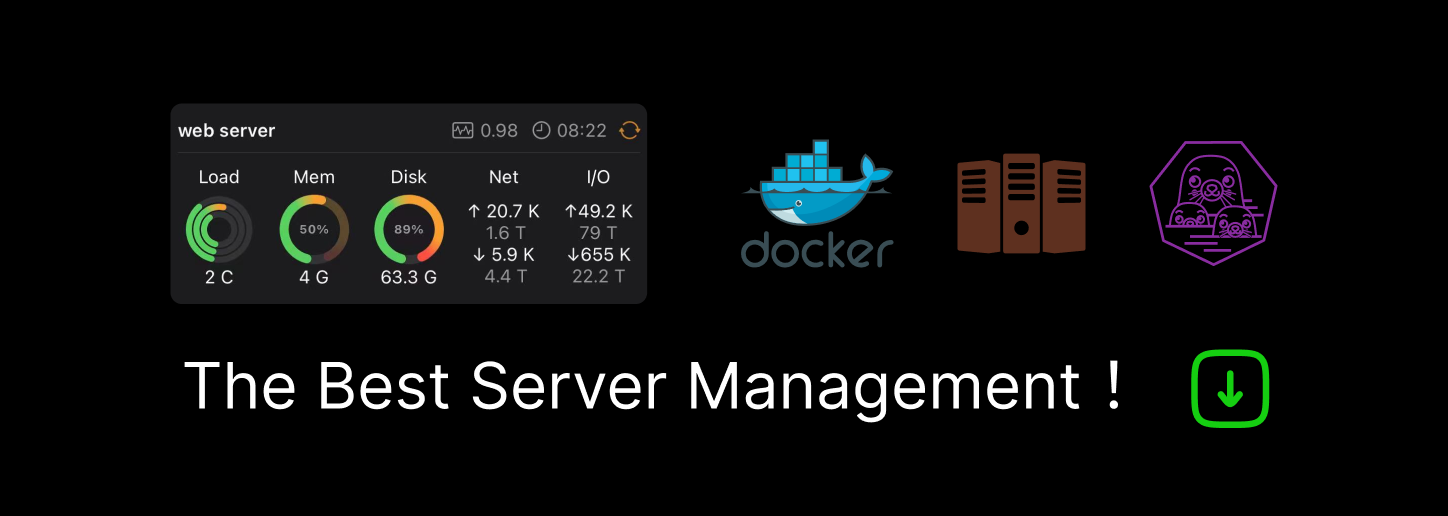
The UK A-Level ‘COVID-19 algorithm fiasco’ and lessons for the enterprise

There were always going to be mistakes made by governments in response to the COVID-19 pandemic. Such a wide-ranging Herculean effort was needed across so many parts of the country, it is unimaginable to think that governments could have gotten everything right. But it's arguable that the British government made some fundamental mishaps that will have long-term consequences for many.
One of the most notable, and jaw dropping, was its handling of education throughout the course of the past 18 months. Think tank Institute for Government has released a report breaking down all of the errors and poorly thought out decisions in a new report, which makes for rather depressing reading. The consequences for young peoples' learning may be seen for years to come.
However, the headline grabbing faux pas (we are being generous with that description) was how it decided that A-Level students not able to take exams should be graded for their studies. The big idea at the time was to use an ‘algorithm' - or to be more accurate, a statistical model - to standardize grades, based on a mixture of teacher assessment and adjustment.
Teachers would rank children within their class, with those results then put through a "process of standardization", taking into account the prior attainment of students at each school or college in recent years.
The outcome, as we know now, is that some students dropped two grades, children from disadvantaged backgrounds were disproportionately affected, and the ‘algorithm' could not, or did not, take into account that some schools were improving.
We've covered this story before, but upon reading the Institute for Government report today, which goes into greater detail, it struck me that there are lessons to be learned here for the enterprise, when relying upon data modelling to deliver results.
Bias and preconceived notions about the outcome
I've said it before and I'll say it again - it is virtually impossible to remove bias from data, as all data is inherently biased. If your organization has historically looked over people of colour for job applications because of bias on the part of recruiters, that historical data and its biases will be fed into an algorithm and churn out similar results.
But what was telling about England and Scotland's A-Level fiasco, is that much of the ‘bias' (as we will call it here) was driven by those in senior positions having a preconceived idea about what the result should be.
For instance, ministers responsible for making decisions about how to grade students were desperate to avoid grade inflation - something the government had strived for in recent years with exams and marked as a success. One official told Institute for Government:
One of the things that the government has been proudest of since 2010, and one to which they are most committed in respect of school standards, was the work they had done to tackle what they saw as grade inflation under the previous regime. It was a first order principle that was baked in from the beginning.
The outcome was that Ofqual - the body responsible for qualifications and exams regulations - decided to place more weight on the previous performance of schools, rather than teacher assessments. But unsurprisingly, this disadvantaged schools that were improving and excellent students in poor performing schools.
The lesson here for organizations is that those in power wanting a certain outcome to win political points, or seeking to achieve a result that avoids nuance, may well be negatively impacting certain users or customers. Be warned.
Not trusting those in the know
Closely linked to the point above, it was clear that there was a preference to err on the side of caution (maybe that's sensible?), but also not trust those in the know (teachers).
Data is an incredible asset, but past data doesn't always dictate what's going to occur in the future - particularly when you're relying on standardization. And assuming that it will, can often be a dangerous game, as this situation shows.
The government was so worried about teachers overestimating their students' grades that they chose to put more emphasis on past performance of the schools as a whole. One could argue that the data provided by teachers, those that knew the students well, should have been prioritised.
The lesson being, if your organization is operating statistical models at scale and you've got people who know the users or know the data well telling you that the outcome isn't going to be fair - listen to them.
‘Standardization' will always negatively impact some
What's probably most shocking about the A-level disaster is that those in charge, ministers and officials, knew that the standardization process would negatively impact some. It's common sense that going through a process of standardization means that there will be outliers from the average that haven't been treated fairly.
Again, organizations that play this game need to be very careful and not assume, like the British government did, that they can get away with it. The consequence for reputation and trust could be incredibly damaging. As one Department for Education insider told the Institute for Government:
One could have had a different debate, and allowed for some grade inflation. But preventing or at least restricting grade inflation was totemic. It did not need clever civil servants to point out to the secretary of state that when faced, on the results day, with young people disappointed that they had not got what they thought they would get - what their teachers thought on the one hand and what the algorithm had produced - he was not going to win that argument on the news.
He had worked it out for himself. He did not think, none of us thought, that it was impossible to sustain the position, despite the onslaught of attack there would be.
We thought we were in extraordinary times. But with hindsight that was a poor decision. Up to the top of government, politicians understood the hit they would be taking. Though not, obviously, as bad as it was.
My take
Throughout the government's response to developing a system to replace exams, those at the most senior levels branded the algorithm as "fair". Whilst these were incredibly difficult circumstances, it's clear that it could have been predicted from the start that the outcome was not going to be ‘fair' for many. Bias, political agendas, and poor decisions - under the guise of ‘trust the data' - meant that the government not only lost a great deal of trust with the students and parents, but that the futures of these people were hung in the balance. Organizations that want to use AI, algorithms, statistical models - whatever you want to call them - need to be very careful when doing it at scale, because the consequences can be far reaching. Sometimes manual processes and human intervention have their place, particularly when standardization can have such wide ranging negative consequences.
Recommend
About Joyk
Aggregate valuable and interesting links.
Joyk means Joy of geeK