

Similarities between crime and health insurance data | Andrew Wheeler
source link: https://andrewpwheeler.com/2021/08/04/similarities-between-crime-and-health-insurance-data/
Go to the source link to view the article. You can view the picture content, updated content and better typesetting reading experience. If the link is broken, please click the button below to view the snapshot at that time.
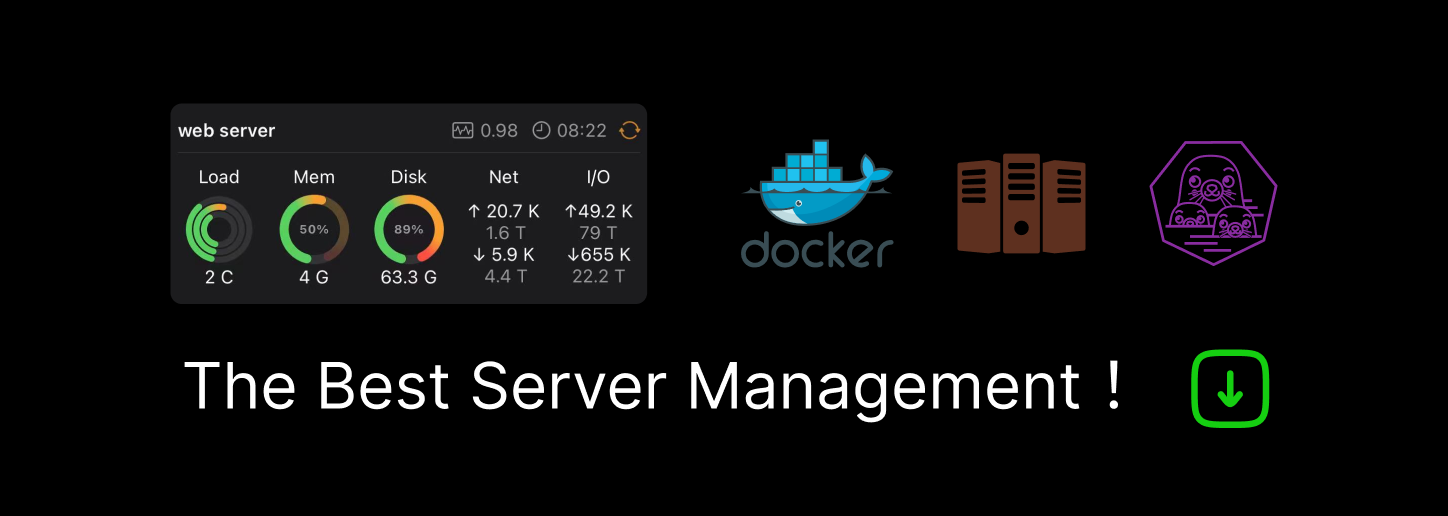
Similarities between crime and health insurance data
One of the things I was mildly worried about when making the jump to the private sector was that the knowledge I had built up from my work in crime analysis over the years would not be transferable. I had basically 10+ year experience working with crime data (directly as a crime analyst at Troy, or when I was a research analyst at the Finn Institute, or when I was doing other collaborations with PDs).
PDs all basically have a similar records management set up. Typical tables are CAD, incident reports, arrests, charges, etc. PDs will have somewhat different fields – but the way they all related to each other are very similar.
Because the company I work for now aggregates health insurance claims from multiple insurance agencies it is a bit more complicated, but there are similarities between how people analyze health insurance claims that in broad strokes are similar to issues with crime data. Below are my musings on that front.
Classifying Events: UCR vs DRG
Historically the predominate way in which people classify what type of crime occurs in a particular incident is via the Uniform Crime Report (UCR) hierarchy. Imagine a crime incident in which someone breaks into a house (burglary), and then also assaults the individual within the home (aggravated assault). When we count these crimes for reporting purposes, we typically take ‘the top charge’, and analyze the event strictly as an assault.
Inpatient health insurance claims (when someone goes to a hospital) have a somewhat unifying classification, Diagnostic Related Groupings, DRG for short. Unlike UCR for general crime reporting though, these are used to bill insurance claims. The idea being that instead of itemizing your hospital bill, insurance companies broadly compensate according to the DRG. This purportedly discourages tacking on extra medical procedures, although brings with it some other problems instead (see the later section in this post on discretion).
Unlike the UCR, DRGs have quite a few more categories, check out the APR DRG weights for New York State for example. For the APR DRG, the DRG also includes a severity category. This I think would be a neat idea for crime incidents – it is somewhat codified in penal laws, but not so much in typical crime reporting. It is somewhat accomplished by folks creating harm weights for crimes (e.g. Ratcliffe, 2015). (There is also a second major DRG used by insurance agencies here in the states, the MS-DRG. That is not a good idea to take from medical records, having multiple common ways to group events!)
One major difference between crimes and health insurance claims are ICD codes. One insurance claim can have multiple ICD codes. For example a claim with an APR DRG of 161 could have ICD codes for:
- I214: Heart Attack
- E119: Diabetes
- I2510: Heart Disease
- E785: High Cholesterol
So there are a mix of chronic conditions (that for billing purposes can modify the severity of the claim), but are not directly related to the current claim/incident/hospital stay.
This could be a neat idea for crime records – say a domestic incident happens, and there is a field to record prior history of domestic incidents. I can see how that would be useful both in the immediate term for the officer handling the call, as well as for an analyst crunching numbers/trends. That being said, ICD codes are crazy in their specificity, so that is not a good thing.
You could also maybe do some other crunching to create your own crime categories based on the individual crime types, see for example Kuang et al. (2017). This is sort of like creating your own DRG for crimes.
Aggregate vs Individual
The point of creating high level groupings is to aggregate multiple events together. In policing, UCR statistics are commonly used to evaluate crime trends over time. Health insurance claims are typically not used for monitoring disease outcomes – since there isn’t any standardized location where they are all collated it would be pretty difficult to use them in that manner for the general pop.
But, overall aggregate statistics pooling claims from particular healthcare providers (e.g. hospitals) are sometimes used for different reimbursement policies. For examples, MIPS is intended as a metric for healthcare providers to promote value based care (Liao & Navathe, 2021), or the CaseMix system (Steinbusch et al., 2007). If you checked out the prior APR DRG list I linked to, you can see they had weights, and higher weights have higher standard billing. The idea behind CaseMix is that if a provider takes on many high weight cases, they get a modifier that ups the weights/billing by a certain percent.
You could maybe consider MIPS to be similar to agencies that give PDs scorecards, aggregating many different metrics together. I rather look at individual metrics though, such as this funnel chart example I give for monitoring use of force. I don’t see much point in aggregating different metrics all together into one final score.
Currently in policing many agencies are migrating from the UCR system, which is just an aggregate tally of events, to NIBRS, which is a database that reports individual events (Kaplan, 2021a, 2021b).
Discretion
Police departments and health care providers (the ones creating the incidents/claims) both have discretion. For PDs, they often want to downgrade the severity of crime incidents, see Thomas and Wolff (2021) for example. Health providers have incentives going the other way though, they have incentives to upcode claims to increase insurance payouts (Farbmacher et al., 2020). Some claims are more fuzzy than others, for example CPT codes that determine a doctors time on a particular office visit are one good example – doctors can just claim they spent larger amounts of time on the office visit (Brunt, 2011).
Like I said previously, health insurance claims are not typically used to monitor overall health outcomes, so non-reporting is not something people really worry about (although researchers should be cognizant of non reporting if they are using insurance claims to look at say policy analysis). The dark figure of crime though is a perpetual threat to the validity of interpreting crime trends.
Health insurance claims have a somewhat opposite problem – submitting claims for when events actually did not happen. One example this occurs is ambulance ghost rides, ambulance billing for events that appear to not have occurred at all (Sanghavi et al., 2021).
Similar to crime events, these reporting/claim errors can either be the result of unintentional accidents, or they can be malicious. Often times, even in retrospect if you know something was in error, it can be difficult to impossible to tell the difference between the two scenarios.
The big difference is $$
The scale of healthcare insurance in the US is massive. Because of this, there is a market to audit these health insurance claims. For example, Georgia is likely to recover nearly half a billion in medical overpayments for the past year. Some of the work I am doing at HMS is related to using machine learning to identify these overpaid Medicare claims. My work is spread across multiple states, but I have easily identified over 8 digits of medical overpayments based on that work in the past year.
There is nothing equivalent to this for policing. There is no monetary incentive for individuals to audit how crime complaints are handled/recorded/resolved.
I wonder if there were a market how much criminal justice would look differently in the United States? For example, say if you had victimization insurance, and detectives worked for the insurance agencies instead of the public sector. This could maybe improve clearance rates, but of course would place more economic burdens on individuals to be insured. That is pure speculation though.
References
- Brunt, C. S. (2011). CPT fee differentials and visit upcoding under Medicare Part B. Health Economics, 20(7), 831-841.
- Farbmacher, H., Löw, L., & Spindler, M. (2020). An explainable attention network for fraud detection in claims management. Journal of Econometrics.
- Kaplan, J. (2021a). National Incident-Based Reporting System (NIBRS) Data: A Practitioner’s Guide.
- Kaplan, J. (2021b). Uniform Crime Reporting (UCR) Program Data: A Practitioner’s Guide.
- Kuang, D., Brantingham, P. J., & Bertozzi, A. L. (2017). Crime topic modeling. Crime Science, 6(12).
- Liao J.M., & Navathe A.S. (2021). Medicare should transform MIPS, not scrap it. Health Affairs Blog.
- Ratcliffe, J. H. (2015). Towards an index for harm-focused policing. Policing: a Journal of Policy and Practice, 9(2), 164-182.
- Sanghavi, P., Jena, A. B., Newhouse, J. P., & Zaslavsky, A. M. (2021). Identifying outlier patterns of inconsistent ambulance billing in Medicare. Health Services Research, 56(2), 188-192.
- Steinbusch, P. J., Oostenbrink, J. B., Zuurbier, J. J., & Schaepkens, F. J. (2007). The risk of upcoding in casemix systems: a comparative study. Health Policy, 81(2-3), 289-299.
- Thomas, A. L., & Wolff, K. T. (2021). Crime distortion within the NYPD: A potential method for estimating crime misclassification within CompStat statistics. Police Practice and Research, 22(4), 1390-1407.
Recommend
About Joyk
Aggregate valuable and interesting links.
Joyk means Joy of geeK