

Opinion: Is your Business Ready for AI on the Edge? - Knoldus Blogs
source link: https://blog.knoldus.com/opinion-is-your-business-ready-for-ai-on-the-edge/
Go to the source link to view the article. You can view the picture content, updated content and better typesetting reading experience. If the link is broken, please click the button below to view the snapshot at that time.
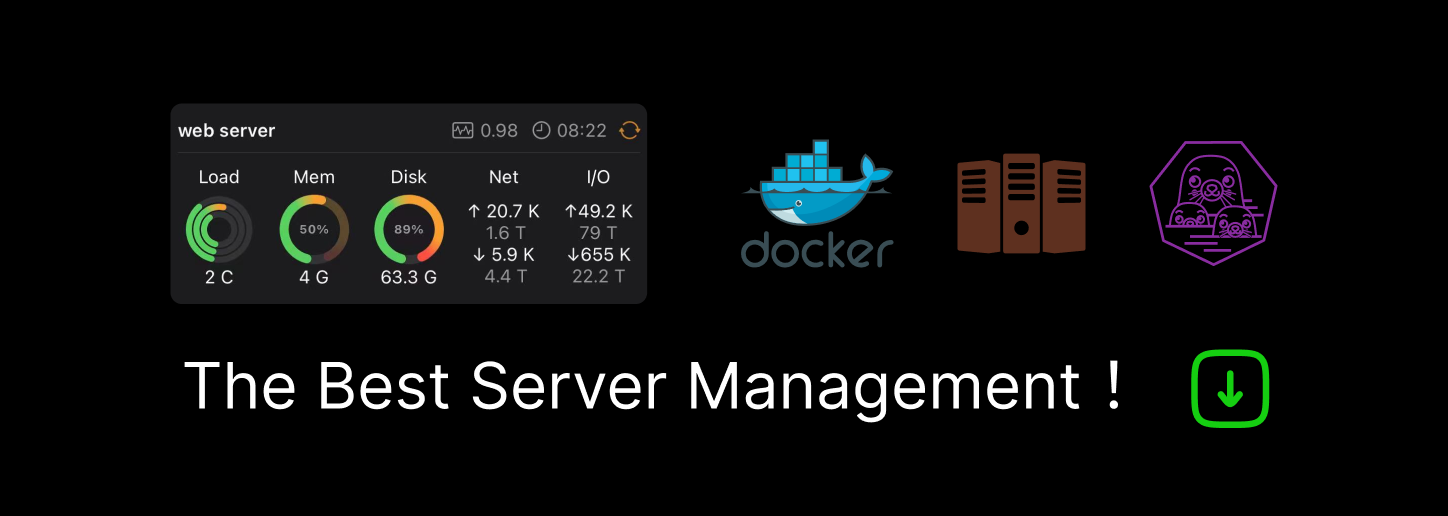
AI has traditionally been deployed in the cloud. AI algorithms crunch massive amounts of data and consume massive computing resources. But AI doesn’t only live in the cloud. In many situations, AI-based data crunching and decisions need to be made locally, on devices that are close to the edge of the network.
At the Edge
AI at the edge allows mission-critical and time-sensitive decisions to be made faster, more reliably and with greater security. Cloud-based AI suffers from latency. The delay as data moves to the cloud for processing and the results are transmitted back over the network to a local device. In many situations, latency can have serious consequences. For instance, when a security camera at an airport or a factory must recognise intruders and react immediately. An autonomous vehicle cannot wait even for a tenth of a second to activate emergency braking when the AI algorithm predicts an imminent collision. In these situations, AI must be located at the edge. This is where decisions can be made faster without relying on network connectivity and without moving massive amounts of data back and forth over a network.
As Gartner analyst Thomas Bittman points out his blog The Edge will Eat the Cloud:
“The agility of cloud computing is great – but it simply isn’t enough. Massive centralization, economies of scale, self-service and full automation get us most of the way there – but it doesn’t overcome physics – the weight of data, the speed of light. As people need to interact with their digitally-assisted realities in real-time, waiting on a data center miles (or many miles) away isn’t going to work,” writes Bittman. “Latency matters. I’m here, right now, and I’m gone in seconds. Put up the right appealing advertising before I look away, point out the store that I’ve been looking for as I drive, let me know that a colleague is heading my way, help my self-driving car avoid other cars through a busy intersection. And do it now.”
Moving AI to the edge has other strong reasons as well
- Across the plethora of industries, the edges are not usually connected to the cloud and they are not smart either. Every industry and every manufacturing unit might need to build smartness in the edge for its own needs. For example, a security camera in a chemical factory might need different set of intelligence tools than the one in manufacturing unit.
- AI will have more economic impact than AI in the cloud. The edge is where real money is being invested right now, and putting AI there even to get a small sliver of increased productivity will have a massive impact.
- While cloud computing offers unquestionable economies of scale, a distributed computing model is driven by the nature of the data itself. The volume of data will make it difficult or expensive to move due to bandwidth costs or availability. The velocity of data will catalyse more real-time applications that cannot be limited by network latency. And the variety of data will be governed by regulatory, privacy and security constraints.
Considerations
Processing Power
The edge needs more processing power. This will enable enterprises to run AI models at the edge, thereby bringing more intelligence to the edge.
Nowadays, a lot of edge devices have inbuilt compute power. A lot of IoT edge devices have GPU, TPU or VPU. For example, some of the high-end security cameras now feature GPU cards, which enables them to run AI-based image recognition models on the edge itself instead of sending all the HD video back to the cloud for processing. Moving the processing to the edge ensures better response times and reduced bandwidth usage.
Lifecycle on the edge
The heterogeneous nature of devices on the edge in an IoT world has its own set of challenges. Remote deployment of the models and monitoring the edge for performance is another big area that has immense potential. One has to have a robust mechanism to deploy and fine-tune the AI models remotely. It’s also critical to keep a close eye on the health of the hardware.
Continuous monitoring of the performance of these models is also a high ask. Managing the continuous deployment, debugging and fine-tuning of AI models on the edge is also an area in which few companies have made real advancements.
Security
Bringing the processing closer to the edge puts more pressure on having rock-solid security in and around the edge. Security at the edge has to be a multi-pronged strategy to ensure the safety of the hardware and software stack. You need to remain vigilant to detect rogue nodes entering the edge network. Once rogue nodes are detected, they need to be isolated and not be allowed to enter the edge network.
Learn
AI that learns at the edge is a paradigm-shifting technology that will finally empower AI to truly serve its purpose. This is by shifting intelligence to the compute edge where it is needed, at speeds, latency and costs that make it affordable for every device. This learning could be brought in by the cloud or better still the edge nodes learn themselves and pass on their learnings to the cloud to be propagated to other edges as well.
Synergies with Cloud
This is not to say that AI On the cloud is a thing of the past. Both edge and cloud would continue to co-exist.
There is a limit to the amount of computation power that can be put into a camera, sensor, or a smartphone. In addition, many of the devices at the edge of the network are not connected to a power source, which raises issues of battery life and heat dissipation. This gives a strong reason to train the model on the cloud and allow it to inference on the edge. Consider an AI-powered vehicle. AI at the edge powers decisions in real time such as braking, steering, and lane changes. At night, when the car is parked and connected to a Wi-Fi network, data is uploaded to the cloud to further train the algorithm. This is called call home data and this would allow the feedback loop to be completed to train the model better. Now consider that there are thousands of vehicles doing this in the night. The central server would take in all this data, work on it and send across a smarter algorithm back to the vehicles in a couple of days not if the next morning.
Conclusion
There will be a need for AI in the cloud, just as there will be more reasons to put AI at the edge. It is an AND depending on where the intelligence needs to live. In this vision of the future, intelligence at the edge will complement intelligence in the cloud, for better balance between the demands of centralised computing and localised decision making.
Recommend
About Joyk
Aggregate valuable and interesting links.
Joyk means Joy of geeK