

DeepMind open-sources AlphaFold 2 for protein structure predictions
source link: https://venturebeat.com/2021/07/16/deepmind-open-sources-alphafold-2-for-protein-structure-predictions/
Go to the source link to view the article. You can view the picture content, updated content and better typesetting reading experience. If the link is broken, please click the button below to view the snapshot at that time.
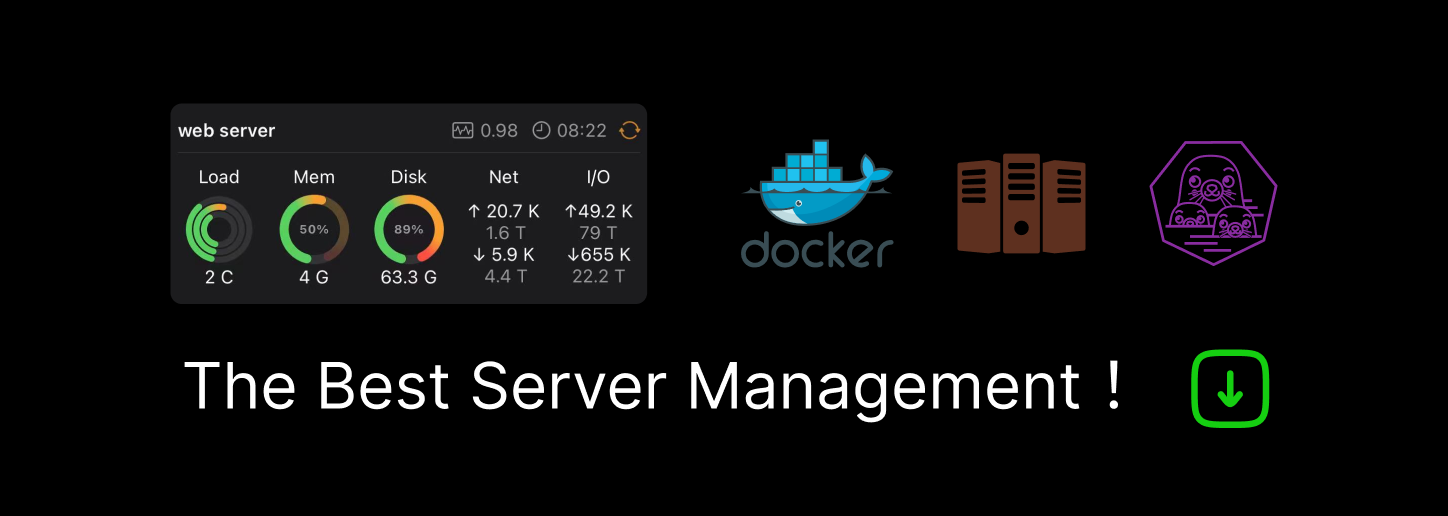
DeepMind open-sources AlphaFold 2 for protein structure predictions

AI is turning the tables on malicious cyberattacks
Learn how AI network security has become the best defense
Register Now!All the sessions from Transform 2021 are available on-demand now. Watch now.
Let the OSS Enterprise newsletter guide your open source journey! Sign up here.
DeepMind this week open-sourced AlphaFold 2, its AI system that predicts the shape of proteins, to accompany the publication of a paper in the journal Nature. With the codebase now available, DeepMind says it hopes to broaden access for researchers and organizations in the health care and life science fields.
The recipe for proteins — large molecules consisting of amino acids that are the fundamental building blocks of tissues, muscles, hair, enzymes, antibodies, and other essential parts of living organisms — are encoded in DNA. It’s these genetic definitions that circumscribe their three-dimensional structures, which in turn determine their capabilities. But protein “folding,” as it’s called, is notoriously difficult to figure out from a corresponding genetic sequence alone. DNA contains only information about chains of amino acid residues and not those chains’ final form.
In December 2018, DeepMind attempted to tackle the challenge of protein folding with AlphaFold, the product of two years of work. The Alphabet subsidiary said at the time that AlphaFold could predict structures more precisely than prior solutions. Its successor, AlphaFold 2, announced in December 2020, improved on this to outgun competing protein-folding-predicting methods for a second time. In the results from the 14th Critical Assessment of Structure Prediction (CASP) assessment, AlphaFold 2 had average errors comparable to the width of an atom (or 0.1 of a nanometer), competitive with the results from experimental methods.
AlphaFold draws inspiration from the fields of biology, physics, and machine learning. It takes advantage of the fact that a folded protein can be thought of as a “spatial graph,” where amino acid residues (amino acids contained within a peptide or protein) are nodes and edges connect the residues in close proximity. AlphaFold leverages an AI algorithm that attempts to interpret the structure of this graph while reasoning over the implicit graph it’s building using evolutionarily related sequences, multiple sequence alignment, and a representation of amino acid residue pairs.
In the open source release, DeepMind says it significantly streamlined AlphaFold 2. Whereas the system took days of computing time to generate structures for some entries to CASP, the open source version is about 16 times faster. It can generate structures in minutes to hours, depending on the size of the protein.
Real-world applications
DeepMind makes the case that AlphaFold, if further refined, could be applied to previously intractable problems in the field of protein folding, including those related to epidemiological efforts. Last year, the company predicted several protein structures of SARS-CoV-2, including ORF3a, whose makeup was formerly a mystery. At CASP14, DeepMind predicted the structure of another coronavirus protein, ORF8, that has since been confirmed by experimentalists.
Beyond aiding the pandemic response, DeepMind expects AlphaFold will be used to explore the hundreds of millions of proteins for which science currently lacks models. Since DNA specifies the amino acid sequences that comprise protein structures, advances in genomics have made it possible to read protein sequences from the natural world, with 180 million protein sequences and counting in the publicly available Universal Protein database. In contrast, given the experimental work needed to translate from sequence to structure, only around 170,000 protein structures are in the Protein Data Bank.
DeepMind says it’s committed to making AlphaFold available “at scale” and collaborating with partners to explore new frontiers, like how multiple proteins form complexes and interact with DNA, RNA, and small molecules. Earlier this year, the company announced a new partnership with the Geneva-based Drugs for Neglected Diseases initiative, a nonprofit pharmaceutical organization that used AlphaFold to identify fexinidazole as a replacement for the toxic compound melarsoprol in the treatment of sleeping sickness.
VentureBeat
VentureBeat's mission is to be a digital town square for technical decision-makers to gain knowledge about transformative technology and transact.
Our site delivers essential information on data technologies and strategies to guide you as you lead your organizations. We invite you to become a member of our community, to access:
- up-to-date information on the subjects of interest to you
- our newsletters
- gated thought-leader content and discounted access to our prized events, such as Transform 2021: Learn More
- networking features, and more
Can AI and cloud automation slash a cloud bill in half?

AI is turning the tables on malicious cyberattacks
Learn how AI network security has become the best defense
Register Now!Presented by CAST.AI
Many companies are accelerating their cloud plans right now, and most say that their cloud usage will exceed prior estimates due to the new demands posed by the global pandemic. Cloud computing is becoming a must-have resource, especially for young tech companies. And most of them are migrating to Amazon, Google, or Azure, lured by seemingly attractive offers.
What many companies don’t realize is how dramatically the cloud spend can increase given that those expenses aren’t charged up-front. Organizations are often unaware of how easy it is to become locked into service at hard-to-understand prices, says Laurent Gil, co-founder and Chief Product Officer at CAST AI.
“Vendor lock-in starts whenever you start using a service in a way that serves the purpose of the cloud provider,” he explains. “You have to choose cloud providers carefully and understand that the decision you make today will impact your operation for at least a few years because leaving this service is going to be very hard.”
The biggest challenge in managing cloud costs
Complexity is a real challenge for startups trying to make DevOps work in the cloud. But what they face is complexity by design on the part of cloud service providers, Gil says, simply because making it easy isn’t in the interest of a cloud provider.
“How do you manage your cloud infrastructure with simple tools that will tell you what’s happening at a glance and whether you’re doing a good job managing it when your cloud bill is 80 pages long?” he asks. “ By design, cloud bills only tell you how much you spent, not why you’re spending that much.”
It’s an urgent question to tackle, particularly for small companies that can now use a few tools that allow you to understand exactly where the money goes, why you spent that much, and why your bill increases every month. And the more you pay for the cloud as the company grows, the more complex and difficult it becomes for humans to make decisions about cost optimization.
“You often don’t realize costs are mounting in the beginning, and then a year or two later, you’re confronted with a technical, financial, or operational debt,” Gil says. “It’s almost as if you inherit this situation. You don’t notice it in the beginning, but it catches up with you in a few months or years.”
To understand cloud costs, you have to go much deeper than the simple ratio of number of customers to the amount of spend. Do you need all these virtual machines or services? Can you use a service from another cloud provider? Will it run cheaper or with less compute in a different cloud? Is there a performance-cost tradeoff — and if so, where it is?
None of these questions are easy to answer unless you use some form of automation. And that’s traditionally been difficult — despite the fact that CPUs, memory, and storage are so readily available everywhere and should be extremely commoditized, Gil adds.
“Tackling these dangerously high cloud bills requires automation,” he says. “Machine learning is capable of rightsizing: adding, deleting, and moving machines on the fly, automatically.”
The role of AI in cloud management
Machine learning is a crucial component in cloud cost optimization because of its ability to recognize and act on patterns. For example, if a SaaS provider experiences a lot of human-based traffic over the course of 24 hours, an AI engine will recognize the pattern to requisition and automatically add machines during busier parts of the day and delete those machines when they’re no longer needed.
An airline may run a rare deep-discount promotion, and millions of people rush online to buy tickets in a wave so large that it looks like a DDoS attack. But since the AI uses a split-second decision-making process, it only needs a moment to recognize a swift and large acceleration in traffic and provision immediately, making the decision to add a virtual machine far faster than a human could have handled it, any time of the day.
“This is where machine learning works great,” explains Gil. “It can make these decisions based on independent business elements that determine how busy an application is.”
The AI engine will always check whether the machines are the right type and use the amount of compute you need. From a DevOps perspective, if you’re using 100 computers that are being used 80 or 90 percent of the time, you’re doing a great job. But an AI can calculate more precisely and check whether you need 100 8-core machines or 50 16-core machines, an ARM processor instead of an Intel processor.
“The AI engine is trained to not make any assumptions, but optimize using any means that it has learned,” Gil says. “If the image of this application is compiled for both Intel and ARM, the AI engine can slash your costs by half just by choosing the right machine at a given time.”
Another example is using spot instances; highly discounted VMs that almost all hyperscale cloud providers offer. The discount is usually between 60 and 80 percent, but the tradeoff is that you only get a short warning when the cloud provider takes those machines back. This is impossible to handle for a human — but an AI can quickly spin up another machine and look for any other available spot instances.
The good thing about using AI in cloud automation is that it can make decisions based on somewhat correlated variables with a limited amount of information.
“It’s a bit of a black box in the end, but as humans we see its results clearly,” Gil says. “We’re can easily judge whether our AI engine is doing a good job based on how much money we save or how much we optimize.”
Cutting customer costs in half
“AI and ML are great tools for reducing the complexity in managing a complex infrastructure for our customers,” Gil says. “If you replace something complex with something else that is also complex, you haven’t done your job.”
A recent CAST AI client, an online grocery store, started using the company’s new product that optimizes EKS applications from Amazon. The forecast report indicated that they could save 50 percent of their time by moving from one type of machine to another.
“Just by doing this, the client reduced their bill from $180,000/month worth of compute to $70,000 after one week, without affecting the performance at all,” Gil says.
“And it’s a good thing for the cloud providers too — whenever you commoditize a resource, customers use more rather than less of it,” he adds. “We’re ensuring that compute capacity is used the right way, democratizing it, and helping companies funnel those costs back into bigger and better projects.”
Sponsored articles are content produced by a company that is either paying for the post or has a business relationship with VentureBeat, and they’re always clearly marked. Content produced by our editorial team is never influenced by advertisers or sponsors in any way. For more information, contact [email protected].
Recommend
About Joyk
Aggregate valuable and interesting links.
Joyk means Joy of geeK