

GitHub - dair-ai/ML-YouTube-Courses: A repository to index and organize the late...
source link: https://github.com/dair-ai/ML-YouTube-Courses
Go to the source link to view the article. You can view the picture content, updated content and better typesetting reading experience. If the link is broken, please click the button below to view the snapshot at that time.
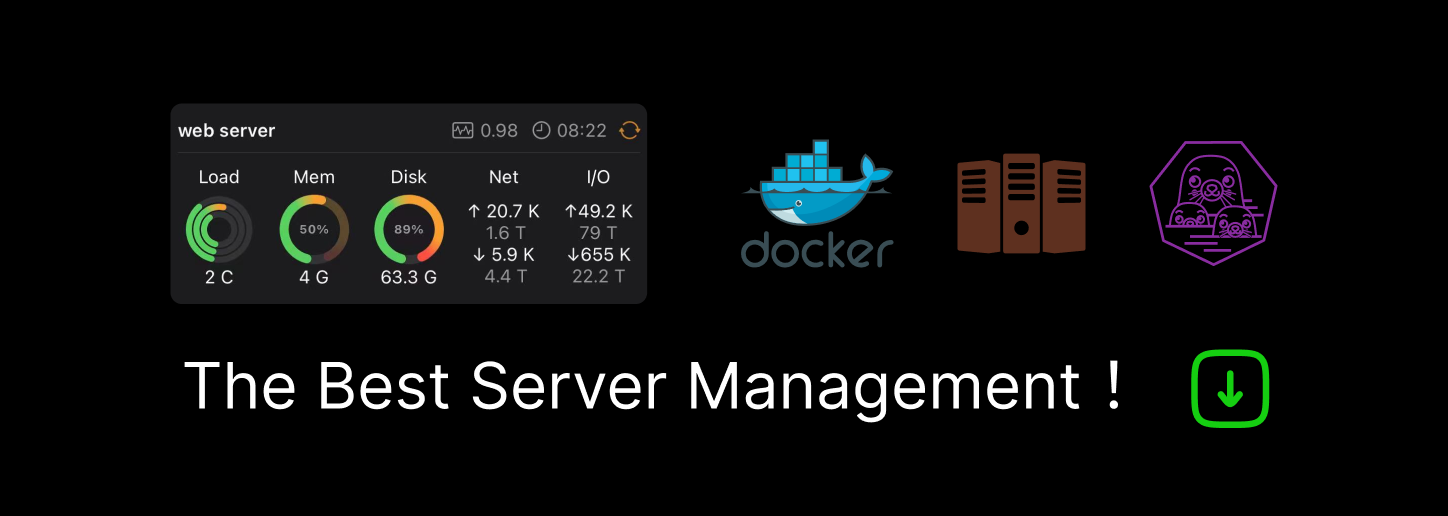
ML YouTube Courses
At dair.ai we open education. We are excited to share some of the best and most recent machine learning courses available on YouTube.
Course List:
Stanford CS229: Machine Learning
To learn some of the basics of ML:
• Linear Regression and Gradient Descent • Logistic Regression • Naive Bayes • SVMs • Kernels • Decision Trees • Introduction to Neural Networks • Debugging ML Models ...
Applied Machine Learning
To learn some of the most widely used techniques in ML:
• Optimization and Calculus • Overfitting and Underfitting • Regularization • Monte Carlo Estimation • Maximum Likelihood Learning • Nearest Neighbours ...
Machine Learning with Graphs (Stanford)
To learn some of the latest graph techniques in machine learning:
• PageRank • Matrix Factorizing • Node Embeddings • Graph Neural Networks • Knowledge Graphs • Deep Generative Models for Graphs ...
Probabilistic Machine Learning
To learn the probabilistic paradigm of ML:
• Reasoning about uncertainty • Continuous Variables • Sampling • Markov Chain Monte Carlo • Gaussian Distributions • Graphical Models • Tuning Inference Algorithms ...
Deep Learning: CS 182
To learn some of the widely used techniques in deep learning:
• Machine Learning Basics • Error Analysis • Optimization • Backpropagation • Initialization • Batch Normalization • Style transfer • Imitation Learning ...
Deep Unsupervised Learning
To learn the latest and most widely used techniques in deep unsupervised learning:
• Autoregressive Models • Flow Models • Latent Variable Models • Self-supervised learning • Implicit Models • Compression ...
NYU Deep Learning SP21
To learn some of the advanced techniques in deep learning:
• Neural Nets: rotation and squashing • Latent Variable Energy Based Models • Unsupervised Learning • Generative Adversarial Networks • Autoencoders ...
CMU Neural Networks for NLP
To learn the latest neural network based techniques for NLP: • Language Modeling • Efficiency tricks • Conditioned Generation • Structured Prediction • Model Interpretation • Advanced Search Algorithms ...
Multilingual NLP
To learn the latest concepts for doing multilingual NLP:
• Typology • Words, Part of Speech, and Morphology • Advanced Text Classification • Machine Translation • Data Augmentation for MT • Low Resource ASR • Active Learning ...
Advanced NLP
To learn advanced concepts in NLP:
• Attention Mechanisms • Transformers • BERT • Question Answering • Model Distillation • Vision + Language • Ethics in NLP • Commonsense Reasoning ...
Deep Learning for Computer Vision
To learn some of the fundamental concepts in CV:
• Introduction to deep learning for CV • Image Classification • Convolutional Networks • Attention Networks • Detection and Segmentation • Generative Models ...
Deep Reinforcement Learning
To learn the latest concepts in deep RL:
• Intro to RL • RL algorithms • Real-world sequential decision making • Supervised learning of behaviors • Deep imitation learning • Cost functions and reward functions ...
Full Stack Deep Learning
To learn full-stack production deep learning:
• ML Projects • Infrastructure and Tooling • Experiment Managing • Troubleshooting DNNs • Data Management • Data Labeling • Monitoring ML Models • Web deployment ...
What's Next?
There are many plans to keep improving this collection. For instance, I will be sharing notes and better organizing individual lectures in a way that provides a bit of guidance for those that are getting started with machine learning.
If you are interested to contribute, feel free to open a PR with links to all individual lectures for each course. It will take a bit of time, but I have plans to do many things with these individual lectures. We can summarize the lectures, include notes, provide additional reading material, include difficulty of content, etc.
Recommend
About Joyk
Aggregate valuable and interesting links.
Joyk means Joy of geeK