

Dear designer: Data analysis doesn’t have to be hard
source link: https://uxdesign.cc/dear-designer-data-analysis-doesnt-have-to-be-hard-bc2e5f0dfbdf
Go to the source link to view the article. You can view the picture content, updated content and better typesetting reading experience. If the link is broken, please click the button below to view the snapshot at that time.
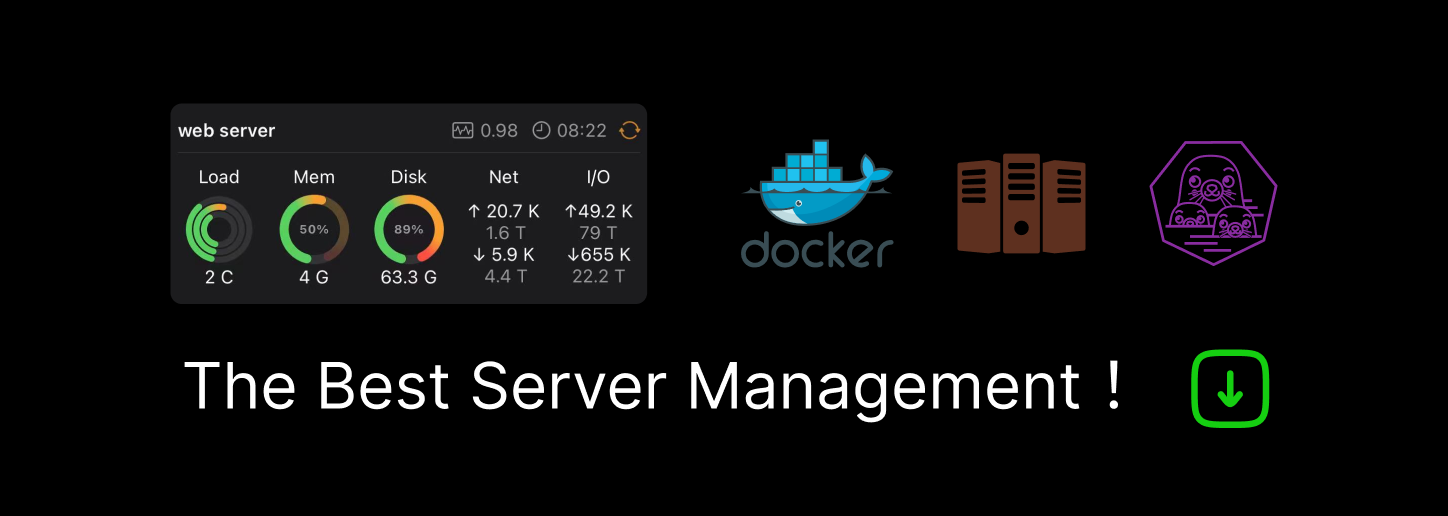
Dear designer: Data analysis doesn’t have to be hard
Useful terms anyone can understand to get the basics down
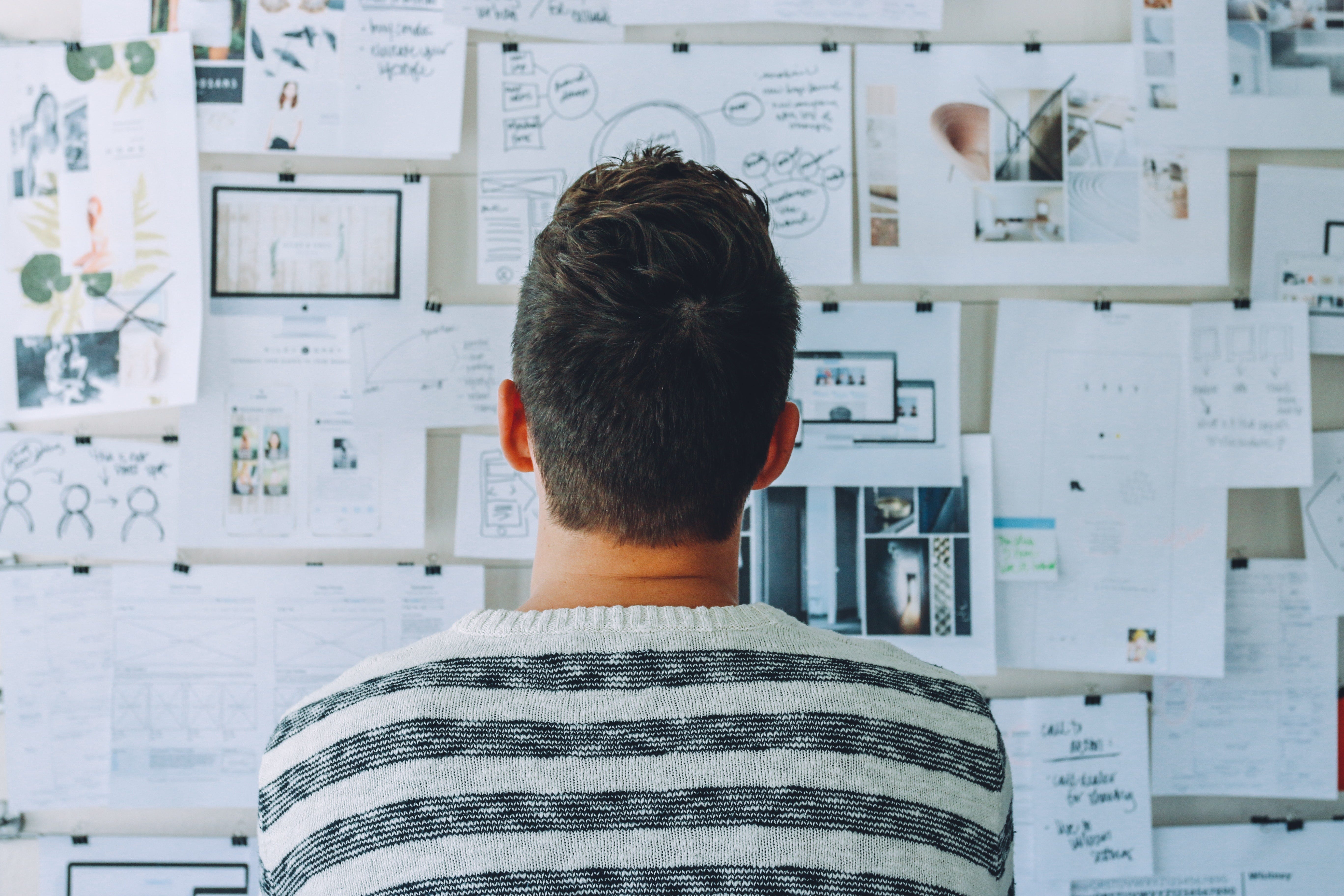
When it comes to UX, everyone loves looking at beautiful software. And while we all enjoy the way our favorite software platforms work, there’s one crucial component to the equation that makes such functionality possible: data.
As designers, we all know this. But why do we love the visual part of design so much more than the rigorous research? Even the folks at Intercom are aware of the “Dribbblisation” that UX Design has trended toward as time goes on. Sadly, we tend to overlook the simple fact that the best designs are often the least visually pleasing ones. It’s actually a form of confirmation bias.
It’s human nature to take the path of least resistance when it comes to learning something unfamiliar. And I believe that’s chiefly because we’re often presented with so much information that we subconsciously resist what we’re supposed to learn.
But as much as us design-first types find research repulsive, I have good news for us: there’s light at the end of the tunnel. Research can be just as fun as building that dream UI you’ve had in your head this whole time. All it takes is understanding the basics of what makes it possible.
Here’s a helpful, clickable index you can use to refer back to these terms if need be:
The Basics: Confidence Intervals
Measuring Decision Making with Metrics
The Basics: Confidence Intervals
When you’re dealing with user data, you’ll often find that ranges play a pretty big role in how that data is seen. This means that the results can be higher or lower than expected, given that you have the right means of getting these results. The range of these results is known as your confidence interval.
To understand the confidence interval, you’ll need four essential ingredients: the Confidence Level, Sample Size, Sample Mean, and Standard Deviation.
Let’s use an example scenario. You’re conducting a user test on a website measuring whether how long users take to create a profile. Your website’s main selling point is that it takes just 30 seconds to sign up. Can we trust this assumption? Confidence intervals can help us find out.

Based on the above sample, we know that we obtained two ranges from the data we collected. One range from a set of 10 users suggests that they took between 15 and 25 seconds. Another set of 10, between 28 and 32 seconds. We could figure out what the standard deviation is based on the ranges given. However, we’d have to choose which one of these ranges is better to start with first.
To gather the most accurate data, we’ll have to start with the smaller range. Why? Because smaller ranges mean a narrow confidence interval, meaning that the average time it takes for someone to sign up doesn’t deviate too far from the norm (30 seconds, as we discovered before.)
Smaller ranges also mean that the data is more fruitful. So when comparing a larger range and a smaller range of data, choosing the smaller one closer to the mean, or average is usually best.
Measuring Decision Making With Metrics
Data analysis isn’t all about numbers. It’s also about metrics — how those numbers are measured depending on the context. Think about the difference between water and ice. Both are of the same makeup, as in reality, water and ice exist as the displacement of molecules. The difference between the two is discernible based on how those molecules flow, which is determined by the surrounding temperature.
Metrics work the same way. Let’s start with the simplest one first.
Binary metrics are simple. they’re measured in two values — yes and no. Either a user completed a task on your site, or they did not.
There are continuous metrics. These measure a changing variable over time. As a designer, you’ll likely see these when measuring:
- How long it takes for someone to complete something on your site
- The number of errors a user makes when completing a task of your site
- The amount of time it takes to read important copy (disclaimers, signups, etc.)
Lastly, there are discrete metrics, which measure the quality of a variable as it relates to its context. Measuring conversion of customers through your e-commerce website is a great example. A discrete metric would measure how often customers bought something from your website, and if not, then how far those customers got into the purchase process.
And while metrics might tell us the how behind user decisions, user satisfaction is another story. During user research, we use scales of measurement to determine the degree at which a user finds something useful or usable, for that matter.
Measuring User Satisfaction
User satisfaction is a tricky metric to measure. For example, you can’t just ask someone how much they like your idea for an app.. There is actually a form of bias in what you asked— it’s a leading question.
As designers and researchers, we have to watch out for all sorts of bias in our means of gathering qualitative data. Even though we have the best intentions, they can be misguided if we aren’t pragmatic in our judgements.
Luckily, there are ways you can measure user satisfaction without becoming ignorant of these potential pitfalls.
SEQS (Single-Ease Questions)
This post-task questionnaire measures how easy or difficult a task was to complete for a user. Usually measured on a scale of 1(Very Difficult) to 7(Very Easy), SEQs are used after running a user through a task.
The Good: They allow researchers to compare which tasks in a series are more more difficult to complete. It also allows participants more room to provide qualitative data without making them forget important details.
The Bad: Since it’s a fairly new method, collective data from other companies and resources is scarce. This means any data you collect will only be useful within the system you’re measuring.
The System Usability Scale
This 10-variable scale measures the ease-of-use and learnability of a given system. Used after a user test, it’s great for testing multiple versions of a given system.
The Good: This means of measuring user satisfaction works well with the Net Promoter Score, making it useful for understand the likelihood that future users would recommend your system.
The Bad: It’s pretty easy to take advantage of this system of measurement by exploiting acquiescence bias. This means people are more likely to agree with statements due to the binary nature of questions provided (true/false, agree/disagree).
The Net Promoter Score
Usually measured on a scale of 1–10, it’s measured based on three groups: detractors (participants who rate 0–6), promoters (participants who rate 9–10) and the passive population (participants who rate 7–8). All around the Net Promoter Score is a helpful means of measurement — users can respond in a non-lengthy nature (saving you time), and it’s also great to include within surveys.
By subtracting the number of promotersfrom the number of detractorsin your measurement, you can calculate the true net promoter score.
The Good: It’s great for presenting to upper management, as a strong net promoter score correlates with high potential profits and makes customer loyalty easier to quantify.
The Bad: The higher your user population the less precision this method of measurement has. And as this occurs, you’ll have to rely on calculating standard deviations and confidence intervals.
So as you can see, measuring user satisfaction is possible using many different methods. And understanding the methods outlined above is key to building a successful user experience.
What I hope you gained from this
Not only does well-informed research save you time, it also saves you money — and stress.
The most important point I want to drive home is that well-informed research is key to building any successful product — even the best designers can’t do without it. Building your skillset in this field is key, even if you don’t specialize in the field. This is because your ability to create a product that truly serves user needs depends upon your ability to collect the most effective data.
Creating a great design starts with knowing what problem to solve and building something to solve it. And knowing the what starts with the how — the means and methods of understanding.
References
Beyond the NPS: Measuring Perceived Usability with the SUS, NASA-TLX, and the Single Ease Question After Tasks and Usability Testsby Page Laubheimer. Nielson Norman Group, 02/2–18
Confidence Intervals: How To Find It The Easy Way by Stephanie Glen. Statistics How-To, 10/2014
Comparing Hypothesis Tests for Continuous, Binary, and Count Data by Jim Frost. Statistics by Jim, 11/2017.
Recommend
About Joyk
Aggregate valuable and interesting links.
Joyk means Joy of geeK