

【加速】multiprocessing多线程、多进程、并行、numba
source link: https://www.guofei.site/2021/02/13/python_speedup.html
Go to the source link to view the article. You can view the picture content, updated content and better typesetting reading experience. If the link is broken, please click the button below to view the snapshot at that time.
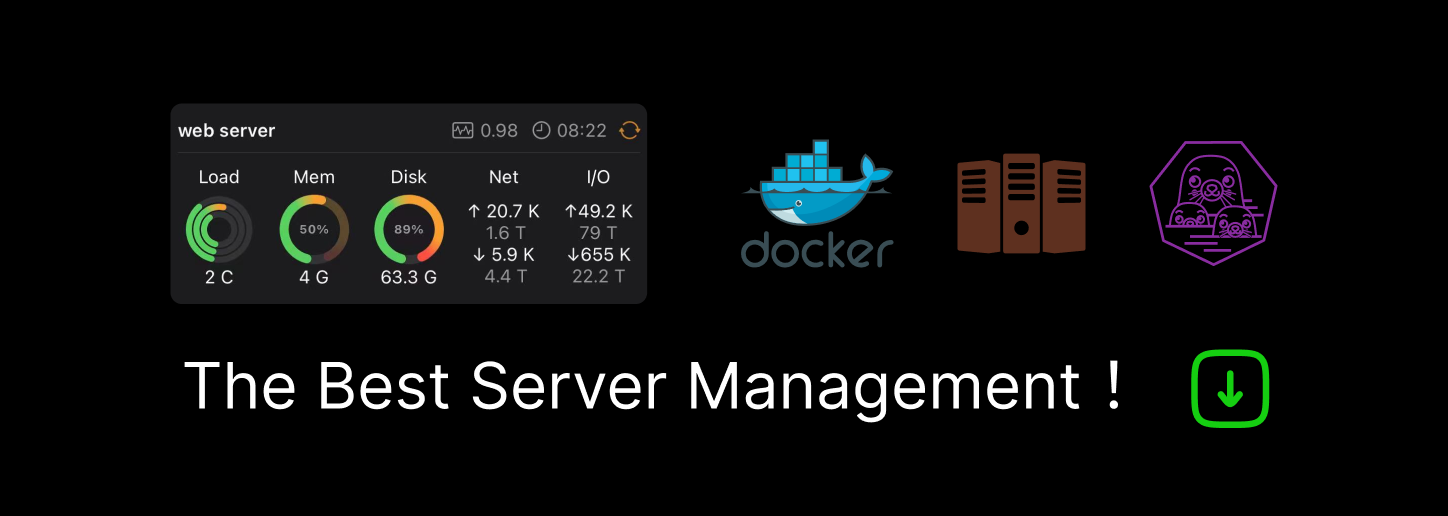
【加速】multiprocessing多线程、多进程、并行、numba
2021年02月13日Author: Guofei
文章归类: Python语法 ,文章编号: 1208
版权声明:本文作者是郭飞。转载随意,但需要标明原文链接,并通知本人
原文链接:https://www.guofei.site/2021/02/13/python_speedup.html
前言
- 多线程。(python 提供了2个多线程接口
thread
提供底层接口。threading
提供高等级接口。)- 一个进程中启动多个线程
- 一般来说使用多线程可以达到并行的目的,
- 但由于Python中使用了全局解释锁GIL的概念,导致Python中的多线程并不是真并行,而是“交替执行” 。所以 Python 多线程适合IO密集型任务,而不适合计算密集型任务。
- 甚至在多核CPU上用多线程执行计算密集任务,由于 GIL 的存在,会导致多核争抢1个GIL,让任务比普通的更慢。
- 多进程(Python 提供
mutliprocess
作为多进程接口。)- 由于Python中GIL的原因,对于计算密集型任务,Python下比较好的并行方式是使用多进程,这样可以非常有效的使用CPU资源。
- 同一时间执行的进程数量取决你电脑的CPU核心数。
测试函数:
import time
import datetime
import os
import multiprocessing
from multiprocessing.dummy import Pool as ThreadPool
from multiprocessing import Pool
import numpy as np
multiprocessing.set_start_method('fork')
print(__name__, os.getpid())
def costly_task(inputs):
task_num, task_type = inputs
# task_mode can be 'io_costly', 'cpu_costly'
ppid = os.getppid()
pid = os.getpid()
start_time = datetime.datetime.now().strftime('%S.%f')
if task_type == 'io_costly':
time.sleep(1)
task_res = 0
else:
n = 500000
step1 = [np.log(i + 1) for i in range(n)] + [np.power(i, 1.1) for i in range(n)]
task_res = sum(step1)
end_time = datetime.datetime.now().strftime('%S.%f')
# print(
# 'task_num={task_num}, task_type={task_type}, __name__={__name__}, pid={pid}, ppid ={ppid}, start_time={start_time}, end_time={end_time}\n'.
# format(task_num=task_num, task_type=task_type, __name__=__name__, pid=pid, ppid=ppid, start_time=start_time,
# end_time=end_time))
return task_res
if __name__ == '__main__':
for task_type in ('io_costly', 'cpu_costly'):
start = datetime.datetime.now()
list(map(costly_task, [[i, task_type] for i in range(10)]))
print(task_type, ', 普通任务', datetime.datetime.now() - start)
start = datetime.datetime.now()
pool = ThreadPool() # ThreadPool(4), 不指定进程数,则使用全部线程
pool.map(costly_task, [[i, task_type] for i in range(10)]) # 返回list,就是结果
print(task_type, ', 多线程', datetime.datetime.now() - start)
start = datetime.datetime.now()
pool = Pool()
pool.map(costly_task, [[i, task_type] for i in range(10)]) # 返回list,就是结果
print(task_type, ', 多进程', datetime.datetime.now() - start)
io_costly , 普通任务 0:00:10.077721
io_costly , 多线程 0:00:03.075839
io_costly , 多进程 0:00:04.180210
cpu_costly , 普通任务 0:00:39.668068
cpu_costly , 多线程 0:00:43.041522
cpu_costly , 多进程 0:00:25.812865
输出符合预期:
- 普通任务是最慢的
- IO密集型任务,多线程最快,多进程因为会抢CPU锁所以比多线程稍慢一些。
- CPU密集型任务,本身已经占满CPU了,所以多线程不会更快,反而因为反复切换资源更慢一些。多进程能加快速度。
另外,多进程下,需要加一行,multiprocessing.set_start_method('fork')
:
spawn
: default on windows,父进程开启一个新进程,新进程只继承父进程 run() 方法相关的必须资源fork
: available on unix, default on unix. 使用 os.fork() 来 forkforkserver
: 同上,但更安全。
如果用 spawn
模式,且不在 if __name__ == '__main__':
下,会进入无限递归然后报错。windows 只有 spawn
模式,目前无法解决,考虑用 sys.platform == 'win32'
判断一下转多线程)
代码解释
from multiprocessing.dummy import Pool as ThreadPool
from multiprocessing import Pool
pool = ThreadPool(processes=4) # 这个是多线程
pool = Pool(processes=4) # 这个是多进程
# 然后两个 pool 都有以下方法(都很有用):
pool.map(func1, range(5)) # 返回list,就是结果
pool.imap(func1, range(10)) # 返回 generator
pool.imap_unordered(func1, range(10)) # 返回generator,并且不要求按顺序
显示可用的cpu数量
import multiprocessing as mp
mp.cpu_count()
另外
以下实现并不能实现并且耗时差不多(很多博客有误导)
a=[func1(i) for i in range(5)]
b=list(map(func1,range(5)))
c=[]
for i in range(5):
c.append(func1(i))
其它实用方法
启动一个子进程
from multiprocessing import Process
p = Process(target=func1, args=('test',))
print(os.getpid()) # 获取主进程的号码
p.start() # 启动子进程
p.join() # 等待到进程结束
批量启动子进程
from multiprocessing import Pool
print('Parent process %s.' % os.getpid())
p = Pool(4)
results=[p.apply_async(func1, args=(i,)) for i in range(5)]
p.close()
p.join()
apply_async
def func(x1, x2):
time.sleep(1)
return x1 + x2 + 1
results = [pool.apply_async(func, args=(x1, x2)) for (x1, x2) in np.random.rand(100, 2)] # 会立即开始运行
results = [p.get() for p in results] # 如果还没运行完,get() 会阻塞等待。
进一步阅读:
多线程:https://zhuanlan.zhihu.com/p/90180209
多进程:https://zhuanlan.zhihu.com/p/93305921
Threading
Threading
封装了 Thread
,提供了更方便的用法。
from threading import Thread
import time
def my_fun(arg1, arg2):
for i in range(10):
print(i)
time.sleep(0.5)
print(arg1, arg2)
t1 = Thread(target=my_fun, args=(1, 1))
t1.setDaemon(True) # 如果主线程结束,子线程 t1 也立即结束。
t1.start()
# t1.join() # 等待 t1 执行完,主进程然后才向下执行
t1.join(timeout=2) # t1 执行 3 秒后,主线程同时向下执行
print(t1.getName())
print('end')
setDaemon
如果主线程结束,子线程 t1 也立即结束。(pycharm 的 scientific 模式不生效,命令行启动py脚本时生效)join
主进程等待子进程 n 秒,然后主进程往下执行setDaemon
和join
可以一起用,效果是子线程执行 n 秒继续执行from multiprocessing import Process
是多进程,使用方法和Thread
一样
面向对象实现多线程:
from threading import Thread
class MyThread(Thread):
def run(self):
print('我是线程')
t1 = MyThread()
t1.start()
重写了 Thread.run
方法。其实原本的 Thread
方法就是调用 target 的。
生产者-消费者模型
这个模式中,生产者多线程的生产包子,消费者多线程的吃包子。
import threading
import time
def producer(name, stack):
while True:
time.sleep(1)
if len(stack) < 10:
stack.append('包子')
print(name + '生产了一个包子' + '\n')
else:
print('篮子满了,停止生产')
def consumer(name, stack):
while True:
time.sleep(1)
if stack:
item = stack.pop()
print(name + '消费了一个' + item + '\n')
else:
print(name + '没拿到包子')
stack = list()
# 3个厨师3线程去做包子
cookers = [threading.Thread(target=producer, args=(cooker_name, stack)) for cooker_name in ['厨师张三', '厨师李四', '厨师王五']]
[cooker.start() for cooker in cookers]
# 20 个消费者多线程去吃包子
consumers = [threading.Thread(target=consumer, args=('consumer' + str(i), stack)) for i in range(5)]
[consumer.start() for consumer in consumers]
也可以用类来实现:
from threading import Thread
import time
class Producer(Thread):
def __init__(self, name, stack):
super().__init__()
self.name = name
self.stack = stack
def run(self):
while True:
time.sleep(1)
if not self.stack:
self.stack.append('包子')
print(self.name + '生产了一个包子' + '\n')
class Consumer(Thread):
def __init__(self, name, stack):
super().__init__()
self.name = name
self.stack = stack
def run(self) -> None:
while True:
time.sleep(1)
if self.stack:
item = self.stack.pop()
print(self.name + '消费了一个' + item + '\n')
stack = list()
# 3个厨师3线程去做包子
cookers = [Producer(name=cooker_name, stack=stack) for cooker_name in ['厨师张三', '厨师李四', '厨师王五']]
[cooker.start() for cooker in cookers]
# 20 个消费者多线程去吃包子
consumers = [Consumer(name='consumer' + str(i), stack=stack) for i in range(10)]
[consumer.start() for consumer in consumers]
多线程中的事件
import threading
import time
def producer():
print('等人来买包子')
event.wait() # 这里会等待 event
print('卖掉包子')
def consumer():
time.sleep(2)
print('买包子')
event.set()
event = threading.Event()
p = threading.Thread(target=producer)
c = threading.Thread(target=consumer)
p.start()
c.start()
等人来买包子
买包子
卖掉包子
进程/线程间通信
线程之间通信很简单,借用类似 list 的特性即可
import threading
def my_func(i, lst):
lst.append(i)
print(lst)
lst = list()
ts = [threading.Thread(target=my_func, args=(i, lst)) for i in range(5)]
[t.start() for t in ts]
print(lst)
[0, 1, 2, 3, 4]
如果把上面的 threading.Thread
换成 multiprocessing.Process
(就是多线程换成多进程),发现每个进程复制了1份,各不相同,主进程的那一份 lst
保持空的。(简单来说,进程会把内存都复制一份,并且每一份相互不影响)
为解决多进程的通信问题, multiprocessing
提供了两个数据类型 Value
, Array
,这两个数据类型的特点是:在一个进程中更改,在所有进程生效
from multiprocessing import Process
import multiprocessing
multiprocessing.set_start_method('fork')
def my_func(value1, arr1):
value1.value += 1 # 要点1:Value 对象可以在一个进程中更改,在所有进程生效
arr1[value1.value] += 1 # 要点2:Array 对象同上
print(list(arr1))
value1 = multiprocessing.Value('i', 0) # d 是小数类型,i是整数类型
arr1 = multiprocessing.Array('d', [1, 1, 1, 1, 1])
ts = [Process(target=my_func, args=(value1, arr1)) for i in range(3)]
[t.start() for t in ts]
print(list(arr1))
[1.0, 2.0, 1.0, 1.0, 1.0]
[1.0, 2.0, 2.0, 1.0, 1.0]
[1.0, 2.0, 2.0, 1.0, 1.0]
[1.0, 2.0, 2.0, 2.0, 1.0]
manager = multiprocessing.Manager()
功能更多:d = manager.dict()
l = manager.list(range(10))
- d 和 l 可以作为入参传入,就像上面的
value1
,arr1
一样 Manager
相比稍慢
另一个并行工具 mpi4py,基于MPI-1/MPI-2
subprocess
用来并行启动外部进程
lru_cache
如果某一个函数多次执行某一类输入,例如,用递归计算斐波那契数列f(5)时,会频繁计算 f(2),那么我们需要一个机制,可以把之前的计算缓存下来,下次优先去找缓存里面的结果,性能就得到优化。
实现方法很多:
- 最简单的可以做一个
dict
- 使用 decorator
- 使用 functools.lru_cache,这个最优雅
import functools
@functools.lru_cache(maxsize=None, typed=None)
def add(x, y):
print('计算 {x} + {y}'.format(x=x, y=y))
return x + y
print(add(1, 2))
print(add(1, 2))
print(add(3, 5))
打印结果:
计算 1 + 2
3
3
计算 3 + 5
8
看到,第二次调用 add(1,2)
,没有真正执行函数。
参数说明:
maxsize
: cache 容量,None
表示无限大typed
: 如果为 True,会按照type分类,例如,f(3.0)
和f(3)
会被当成不同的调用
numba 专题
numba为什么能加速?
python编译过程有4步
- 词法分析:检查关键字是否正确
- 语法分析:检查语法是否正确
- 生成字节码:生成就是pyc文件。解释器的类型有cpython、IPython、PyPy、Jython、IronPython。
- 执行。常见的cpython解释器是用c语言的方式来解释字节码的,而numba则是使用LLVM编译技术来解释字节码的。LLVM是一个编译器,它采用代码的特殊中间表示(IR)并将其编译为本机(机器)代码。编译过程涉及许多额外的传递,其中LLVM编译器可以优化IR。LLVM工具链非常擅长优化IR,因此它不仅可以编译Numba的代码,还可以优化它。
import numpy as np
from numba import jit
a = np.arange(1, 10 ** 7)
b = np.arange(-10 ** 7, -1)
@jit(nopython=True)
def sum_sequence(a, b):
result = np.zeros_like(a)
for i in range(len(a)):
result[i] = a[i] - b[i]
return result
import dis
dis.dis(sum_sequence)
Numba有两种模式:nopython和object。前者不使用Python运行时并生成没有Python依赖的本机代码。本机代码是静态类型的,运行速度非常快。而对象模式使用Python对象和Python C API,而这通常不会显着提高速度。在这两种情况下,Python代码都是使用LLVM编译的。
Numba的优点:
- 自动并行化
- 支持numpy操作和对象
- 支持调用GPU
Numba的缺点:
- debug非常麻烦
- 无法在nopython模式下与Python及其模块进行交互,numba目前在nopython模式下支持python模块有限,比如pandas是不支持的,但是不支持意味着无法加速并不意味着不能运行。
- 对python中的类class支持有限
测试代码:
import numpy as np
import datetime
def sum_array1(arr):
length, height = arr.shape
sum_res = 0
for i in range(length):
for j in range(height):
sum_res += arr[i, j]
return sum_res
start_time = datetime.datetime.now()
for i in range(500):
arr = np.random.random((500, 500))
sum_array1(arr)
print('普通:', datetime.datetime.now() - start_time)
# %%
def sum_array2(arr):
return arr.sum()
start_time = datetime.datetime.now()
for i in range(500):
arr = np.random.random((500, 500))
sum_array2(arr)
print('numpy 计算:', datetime.datetime.now() - start_time)
# %%
from numba import jit
@jit
def sum_array3(arr):
length, height = arr.shape
sum_res = 0
for i in range(length):
for j in range(height):
sum_res += arr[i, j]
return sum_res
start_time = datetime.datetime.now()
for i in range(500):
arr = np.random.random((500, 500))
sum_array3(arr)
print('numba 加速', datetime.datetime.now() - start_time)
# %%
@jit(nopython=True)
def sum_array4(arr):
length, height = arr.shape
sum_res = 0
for i in range(length):
for j in range(height):
sum_res += arr[i, j]
return sum_res
start_time = datetime.datetime.now()
for i in range(500):
arr = np.random.random((500, 500))
sum_array4(arr)
print('numba 加速,nopython=True', datetime.datetime.now() - start_time)
普通: 0:00:30.612512
numpy 计算: 0:00:00.665852
numba 加速 0:00:00.917495
numba 加速,nopython=True 0:00:00.798196
numba 快了几十倍。任务正好是 numpy 擅长的,所以 numpy 最快,加上 nopython=True
后更快。
官方建议使用 nopython=True
,这样的话,遇到不能加速的函数会直接报错,让你知道。
@jit(nopython=True,parallel=True,fastmath=True)
@numba.jit(signature = None,nopython = False,nogil = False,cache = False,forceobj = False,parallel = False,error_model ='python',fastmath = False,locals = {} )
#
#
#
# fastmath=True,关闭一些严格的浮点运算标准,可以使速度提升1.8倍
- signature 类似这种形式 (numba.int32,numba.double),用于提前定义输入参数的数据类型,不过对于提速没啥用。和cython不一样,cython的静态数据类型能够提速非常多
- nopython=True,就是不用 python 解释器参与,略微提升效率。建议开启,因为这样的话,遇到不能加速的函数会直接报错,让你知道。
- nogil = False,用于去除python的gil锁,只有当Numba可以在nopython模式下编译函数时才会释放GIL,否则将打印编译警告。
- cache=False 是否缓存编译后的文件,缓存后的文件保存在
__pycache__
或者指定的地方例如$HOME/.cache/numba
。如果不缓存则每一次调用都需要重新编译,编译耗时比较长的程序建议设置cache为True,并非所有函数都可以缓存,无法缓存函数时,会发出警告。 - fastmath=False 前面也介绍过了,如果为true,则fastmath允许使用LLVM文档中描述的其他不安全的浮点变换。
- parallel=True,实测打开并行可以在已经提速的基础上把速度提高到十万倍。
- error_model 控制除以零行为。将它设置为’python’会导致被零除以引发像CPython这样的异常。将其设置为’numpy’会导致被零除以将结果设置为+/- inf或nan。
vectorize
# 全局设置多线程
# numba.config.NUMBA_NUM_THREADS=8
from numba import vectorize
@vectorize('float64(float64)',target='parallel')
def my_func4(x):
res = 0
for x in range(n):
res = 0
for i in range(x):
res += i
return res
# 如果入参是 array:
@guvectorize('float64[:,:], float64[:,:], float64[:,:]',
'(m,n),(n,p)->(m,p)')
def matmul(A, B, C):
m, n = A.shape
n, p = B.shape
for i in range(m):
for j in range(p):
C[i, j] = 0
for k in range(n):
C[i, j] += A[i, k] * B[k, j]
a = numpy.random.random((500, 500))
out = matmul(a, a, numpy.zeros_like(a))
另外,vectorize 和 jit 连用反而会降低速度(所以不建议)
一些测试结果
一些辅助测试的方法:
# numba 可以根据上下文推测数据类型,把推测的数据类型显示出来:
sum_array4.inspect_types()
# 测试每行代码的效率
import cProfile
cProfile.run('sum_array4(150)')
两层循环:
- 只jit内部循环,加速了500倍
- jit两层循环,加速了1400倍
- vectorize,竟然加速了 百万倍???(另外,别人的博客测试结果比 numpy 的 ufunc 提升 30 倍)
- 如果开启parallel=True,并行化,JIT 也能加速到百万倍
numba性能的一些技巧
- JIT 对循环的提升非常强。尽量把循环写在 numba里
参考文献
numba从入门到精通系列:
- https://zhuanlan.zhihu.com/p/68742702
- https://zhuanlan.zhihu.com/p/68743922
- https://zhuanlan.zhihu.com/p/68744646
- https://zhuanlan.zhihu.com/p/68805601
- https://zhuanlan.zhihu.com/p/68846159
- https://zhuanlan.zhihu.com/p/68851264
- https://zhuanlan.zhihu.com/p/68852771
您的支持将鼓励我继续创作!
Recommend
About Joyk
Aggregate valuable and interesting links.
Joyk means Joy of geeK