

Pandas高级教程之:Dataframe的合并
source link: http://www.flydean.com/04-python-pandas-merge/
Go to the source link to view the article. You can view the picture content, updated content and better typesetting reading experience. If the link is broken, please click the button below to view the snapshot at that time.
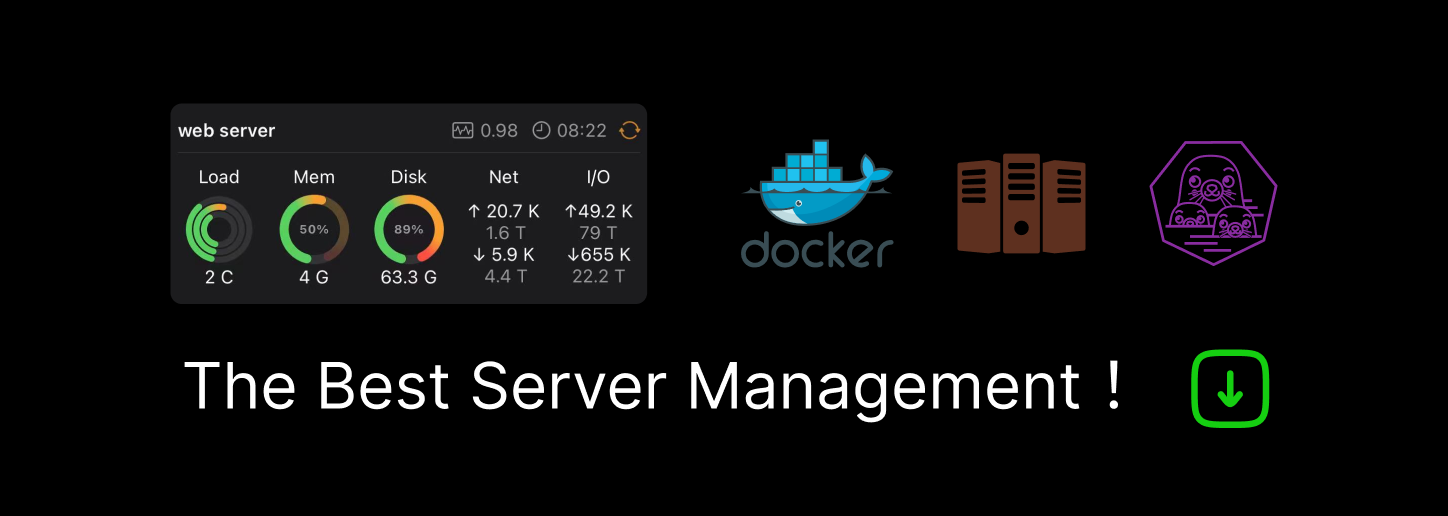
Pandas高级教程之:Dataframe的合并
Pandas提供了很多合并Series和Dataframe的强大的功能,通过这些功能可以方便的进行数据分析。本文将会详细讲解如何使用Pandas来合并Series和Dataframe。
使用concat
concat是最常用的合并DF的方法,先看下concat的定义:
pd.concat(objs, axis=0, join='outer', ignore_index=False, keys=None,
levels=None, names=None, verify_integrity=False, copy=True)
看一下我们经常会用到的几个参数:
objs是Series或者Series的序列或者映射。
axis指定连接的轴。
join
: {‘inner’, ‘outer’}, 连接方式,怎么处理其他轴的index,outer表示合并,inner表示交集。
ignore_index: 忽略原本的index值,使用0,1,… n-1来代替。
copy:是否进行拷贝。
keys:指定最外层的多层次结构的index。
我们先定义几个DF,然后看一下怎么使用concat把这几个DF连接起来:
In [1]: df1 = pd.DataFrame({'A': ['A0', 'A1', 'A2', 'A3'],
...: 'B': ['B0', 'B1', 'B2', 'B3'],
...: 'C': ['C0', 'C1', 'C2', 'C3'],
...: 'D': ['D0', 'D1', 'D2', 'D3']},
...: index=[0, 1, 2, 3])
...:
In [2]: df2 = pd.DataFrame({'A': ['A4', 'A5', 'A6', 'A7'],
...: 'B': ['B4', 'B5', 'B6', 'B7'],
...: 'C': ['C4', 'C5', 'C6', 'C7'],
...: 'D': ['D4', 'D5', 'D6', 'D7']},
...: index=[4, 5, 6, 7])
...:
In [3]: df3 = pd.DataFrame({'A': ['A8', 'A9', 'A10', 'A11'],
...: 'B': ['B8', 'B9', 'B10', 'B11'],
...: 'C': ['C8', 'C9', 'C10', 'C11'],
...: 'D': ['D8', 'D9', 'D10', 'D11']},
...: index=[8, 9, 10, 11])
...:
In [4]: frames = [df1, df2, df3]
In [5]: result = pd.concat(frames)
df1,df2,df3定义了同样的列名和不同的index,然后将他们放在frames中构成了一个DF的list,将其作为参数传入concat就可以进行DF的合并。
举个多层级的例子:
In [6]: result = pd.concat(frames, keys=['x', 'y', 'z'])
使用keys可以指定frames中不同frames的key。
使用的时候,我们可以通过选择外部的key来返回特定的frame:
In [7]: result.loc['y']
Out[7]:
A B C D
4 A4 B4 C4 D4
5 A5 B5 C5 D5
6 A6 B6 C6 D6
7 A7 B7 C7 D7
上面的例子连接的轴默认是0,也就是按行来进行连接,下面我们来看一个例子按列来进行连接,如果要按列来连接,可以指定axis=1:
In [8]: df4 = pd.DataFrame({'B': ['B2', 'B3', 'B6', 'B7'],
...: 'D': ['D2', 'D3', 'D6', 'D7'],
...: 'F': ['F2', 'F3', 'F6', 'F7']},
...: index=[2, 3, 6, 7])
...:
In [9]: result = pd.concat([df1, df4], axis=1, sort=False)
默认的 join='outer'
,合并之后index不存在的地方会补全为NaN。
下面看一个join=’inner’的情况:
In [10]: result = pd.concat([df1, df4], axis=1, join='inner')
join=’inner’ 只会选择index相同的进行展示。
如果合并之后,我们只想保存原来frame的index相关的数据,那么可以使用reindex:
In [11]: result = pd.concat([df1, df4], axis=1).reindex(df1.index)
或者这样:
In [12]: pd.concat([df1, df4.reindex(df1.index)], axis=1)
Out[12]:
A B C D B D F
0 A0 B0 C0 D0 NaN NaN NaN
1 A1 B1 C1 D1 NaN NaN NaN
2 A2 B2 C2 D2 B2 D2 F2
3 A3 B3 C3 D3 B3 D3 F3
看下结果:
可以合并DF和Series:
In [18]: s1 = pd.Series(['X0', 'X1', 'X2', 'X3'], name='X')
In [19]: result = pd.concat([df1, s1], axis=1)
如果是多个Series,使用concat可以指定列名:
In [23]: s3 = pd.Series([0, 1, 2, 3], name='foo')
In [24]: s4 = pd.Series([0, 1, 2, 3])
In [25]: s5 = pd.Series([0, 1, 4, 5])
In [27]: pd.concat([s3, s4, s5], axis=1, keys=['red', 'blue', 'yellow'])
Out[27]:
red blue yellow
0 0 0 0
1 1 1 1
2 2 2 4
3 3 3 5
使用append
append可以看做是concat的简化版本,它沿着axis=0
进行concat:
In [13]: result = df1.append(df2)
如果append的两个 DF的列是不一样的会自动补全NaN:
In [14]: result = df1.append(df4, sort=False)
如果设置ignore_index=True,可以忽略原来的index,并重写分配index:
In [17]: result = df1.append(df4, ignore_index=True, sort=False)
向DF append一个Series:
In [35]: s2 = pd.Series(['X0', 'X1', 'X2', 'X3'], index=['A', 'B', 'C', 'D'])
In [36]: result = df1.append(s2, ignore_index=True)
使用merge
和DF最类似的就是数据库的表格,可以使用merge来进行类似数据库操作的DF合并操作。
先看下merge的定义:
pd.merge(left, right, how='inner', on=None, left_on=None, right_on=None,
left_index=False, right_index=False, sort=True,
suffixes=('_x', '_y'), copy=True, indicator=False,
validate=None)
Left, right是要合并的两个DF 或者 Series。
on代表的是join的列或者index名。
left_on:左连接
right_on
:右连接
left_index
: 连接之后,选择使用左边的index或者column。
right_index
:连接之后,选择使用右边的index或者column。
how:连接的方式,'left'
, 'right'
, 'outer'
, 'inner'
. 默认 inner
.
sort
: 是否排序。
suffixes
: 处理重复的列。
copy
: 是否拷贝数据
先看一个简单merge的例子:
In [39]: left = pd.DataFrame({'key': ['K0', 'K1', 'K2', 'K3'],
....: 'A': ['A0', 'A1', 'A2', 'A3'],
....: 'B': ['B0', 'B1', 'B2', 'B3']})
....:
In [40]: right = pd.DataFrame({'key': ['K0', 'K1', 'K2', 'K3'],
....: 'C': ['C0', 'C1', 'C2', 'C3'],
....: 'D': ['D0', 'D1', 'D2', 'D3']})
....:
In [41]: result = pd.merge(left, right, on='key')
上面两个DF通过key来进行连接。
再看一个多个key连接的例子:
In [42]: left = pd.DataFrame({'key1': ['K0', 'K0', 'K1', 'K2'],
....: 'key2': ['K0', 'K1', 'K0', 'K1'],
....: 'A': ['A0', 'A1', 'A2', 'A3'],
....: 'B': ['B0', 'B1', 'B2', 'B3']})
....:
In [43]: right = pd.DataFrame({'key1': ['K0', 'K1', 'K1', 'K2'],
....: 'key2': ['K0', 'K0', 'K0', 'K0'],
....: 'C': ['C0', 'C1', 'C2', 'C3'],
....: 'D': ['D0', 'D1', 'D2', 'D3']})
....:
In [44]: result = pd.merge(left, right, on=['key1', 'key2'])
How 可以指定merge方式,和数据库一样,可以指定是内连接,外连接等:
合并方法 SQL 方法left
LEFT OUTER JOIN
right
RIGHT OUTER JOIN
outer
FULL OUTER JOIN
inner
INNER JOIN
In [45]: result = pd.merge(left, right, how='left', on=['key1', 'key2'])
指定indicator=True ,可以表示具体行的连接方式:
In [60]: df1 = pd.DataFrame({'col1': [0, 1], 'col_left': ['a', 'b']})
In [61]: df2 = pd.DataFrame({'col1': [1, 2, 2], 'col_right': [2, 2, 2]})
In [62]: pd.merge(df1, df2, on='col1', how='outer', indicator=True)
Out[62]:
col1 col_left col_right _merge
0 0 a NaN left_only
1 1 b 2.0 both
2 2 NaN 2.0 right_only
3 2 NaN 2.0 right_only
如果传入字符串给indicator,会重命名indicator这一列的名字:
In [63]: pd.merge(df1, df2, on='col1', how='outer', indicator='indicator_column')
Out[63]:
col1 col_left col_right indicator_column
0 0 a NaN left_only
1 1 b 2.0 both
2 2 NaN 2.0 right_only
3 2 NaN 2.0 right_only
多个index进行合并:
In [112]: leftindex = pd.MultiIndex.from_tuples([('K0', 'X0'), ('K0', 'X1'),
.....: ('K1', 'X2')],
.....: names=['key', 'X'])
.....:
In [113]: left = pd.DataFrame({'A': ['A0', 'A1', 'A2'],
.....: 'B': ['B0', 'B1', 'B2']},
.....: index=leftindex)
.....:
In [114]: rightindex = pd.MultiIndex.from_tuples([('K0', 'Y0'), ('K1', 'Y1'),
.....: ('K2', 'Y2'), ('K2', 'Y3')],
.....: names=['key', 'Y'])
.....:
In [115]: right = pd.DataFrame({'C': ['C0', 'C1', 'C2', 'C3'],
.....: 'D': ['D0', 'D1', 'D2', 'D3']},
.....: index=rightindex)
.....:
In [116]: result = pd.merge(left.reset_index(), right.reset_index(),
.....: on=['key'], how='inner').set_index(['key', 'X', 'Y'])
支持多个列的合并:
In [117]: left_index = pd.Index(['K0', 'K0', 'K1', 'K2'], name='key1')
In [118]: left = pd.DataFrame({'A': ['A0', 'A1', 'A2', 'A3'],
.....: 'B': ['B0', 'B1', 'B2', 'B3'],
.....: 'key2': ['K0', 'K1', 'K0', 'K1']},
.....: index=left_index)
.....:
In [119]: right_index = pd.Index(['K0', 'K1', 'K2', 'K2'], name='key1')
In [120]: right = pd.DataFrame({'C': ['C0', 'C1', 'C2', 'C3'],
.....: 'D': ['D0', 'D1', 'D2', 'D3'],
.....: 'key2': ['K0', 'K0', 'K0', 'K1']},
.....: index=right_index)
.....:
In [121]: result = left.merge(right, on=['key1', 'key2'])
使用join
join将两个不同index的DF合并成一个。可以看做是merge的简写。
In [84]: left = pd.DataFrame({'A': ['A0', 'A1', 'A2'],
....: 'B': ['B0', 'B1', 'B2']},
....: index=['K0', 'K1', 'K2'])
....:
In [85]: right = pd.DataFrame({'C': ['C0', 'C2', 'C3'],
....: 'D': ['D0', 'D2', 'D3']},
....: index=['K0', 'K2', 'K3'])
....:
In [86]: result = left.join(right)
可以指定how来指定连接方式:
In [87]: result = left.join(right, how='outer')
默认join是按index来进行连接。
还可以按照列来进行连接:
In [91]: left = pd.DataFrame({'A': ['A0', 'A1', 'A2', 'A3'],
....: 'B': ['B0', 'B1', 'B2', 'B3'],
....: 'key': ['K0', 'K1', 'K0', 'K1']})
....:
In [92]: right = pd.DataFrame({'C': ['C0', 'C1'],
....: 'D': ['D0', 'D1']},
....: index=['K0', 'K1'])
....:
In [93]: result = left.join(right, on='key')
单个index和多个index进行join:
In [100]: left = pd.DataFrame({'A': ['A0', 'A1', 'A2'],
.....: 'B': ['B0', 'B1', 'B2']},
.....: index=pd.Index(['K0', 'K1', 'K2'], name='key'))
.....:
In [101]: index = pd.MultiIndex.from_tuples([('K0', 'Y0'), ('K1', 'Y1'),
.....: ('K2', 'Y2'), ('K2', 'Y3')],
.....: names=['key', 'Y'])
.....:
In [102]: right = pd.DataFrame({'C': ['C0', 'C1', 'C2', 'C3'],
.....: 'D': ['D0', 'D1', 'D2', 'D3']},
.....: index=index)
.....:
In [103]: result = left.join(right, how='inner')
列名重复的情况:
In [122]: left = pd.DataFrame({'k': ['K0', 'K1', 'K2'], 'v': [1, 2, 3]})
In [123]: right = pd.DataFrame({'k': ['K0', 'K0', 'K3'], 'v': [4, 5, 6]})
In [124]: result = pd.merge(left, right, on='k')
可以自定义重复列名的命名规则:
In [125]: result = pd.merge(left, right, on='k', suffixes=('_l', '_r'))
有时候我们需要使用DF2的数据来填充DF1的数据,这时候可以使用combine_first:
In [131]: df1 = pd.DataFrame([[np.nan, 3., 5.], [-4.6, np.nan, np.nan],
.....: [np.nan, 7., np.nan]])
.....:
In [132]: df2 = pd.DataFrame([[-42.6, np.nan, -8.2], [-5., 1.6, 4]],
.....: index=[1, 2])
.....:
In [133]: result = df1.combine_first(df2)
或者使用update:
In [134]: df1.update(df2)
本文已收录于 http://www.flydean.com/04-python-pandas-merge/
最通俗的解读,最深刻的干货,最简洁的教程,众多你不知道的小技巧等你来发现!
欢迎关注我的公众号:「程序那些事」,懂技术,更懂你!
Recommend
About Joyk
Aggregate valuable and interesting links.
Joyk means Joy of geeK